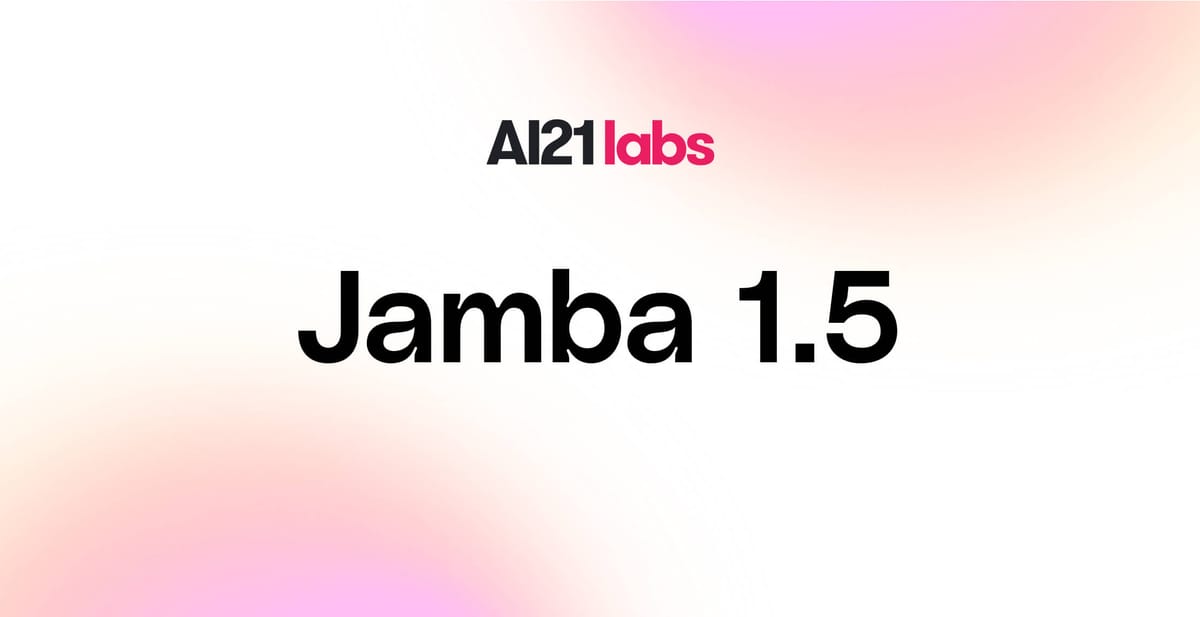
AI21 has launched the Jamba 1.5 model family, setting a new standard for long-context AI models. This release introduces two powerful open models: Jamba 1.5 Mini and Jamba 1.5 Large, designed to offer unparalleled speed, efficiency, and performance for enterprise AI applications.
At the heart of these models is a hybrid SSM-Transformer architecture that combines Transformer and Mamba layers with a Mixture-of-Experts approach. This innovative design allows Jamba models to process vast amounts of information with exceptional speed and accuracy. Jamba models are up to 2.5X faster than competitors in their size class, making them ideal for tasks that require the processing of extensive data, such as financial analysis, document summarization, and customer service applications.
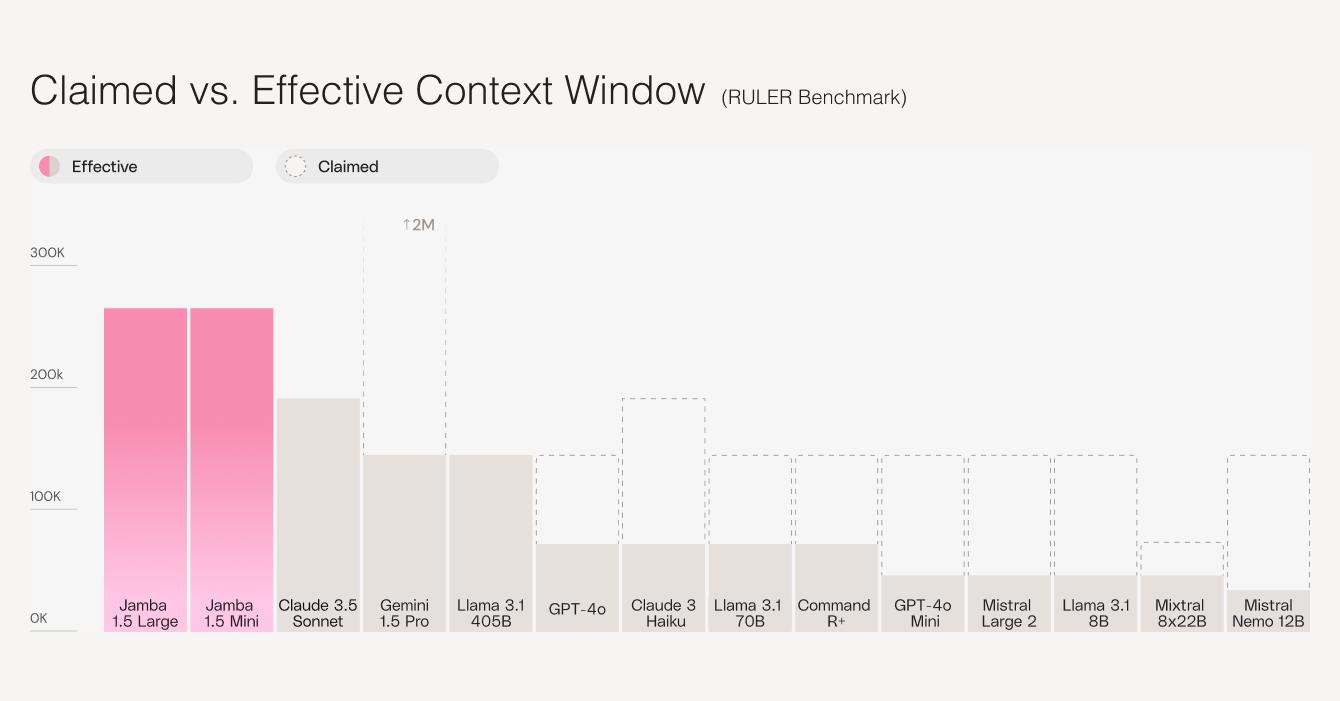
The standout feature of the Jamba 1.5 family is its impressive 256K token context window (about 800 pages of text), currently the largest available among open models today. This expansive context enables the models to handle complex tasks like document analysis and multi-step reasoning with unprecedented effectiveness. By eliminating the need for constant data chunking, the Jamba 1.5 models reduce both time and cost, while boosting performance for Retrieval-Augmented Generation workflows.
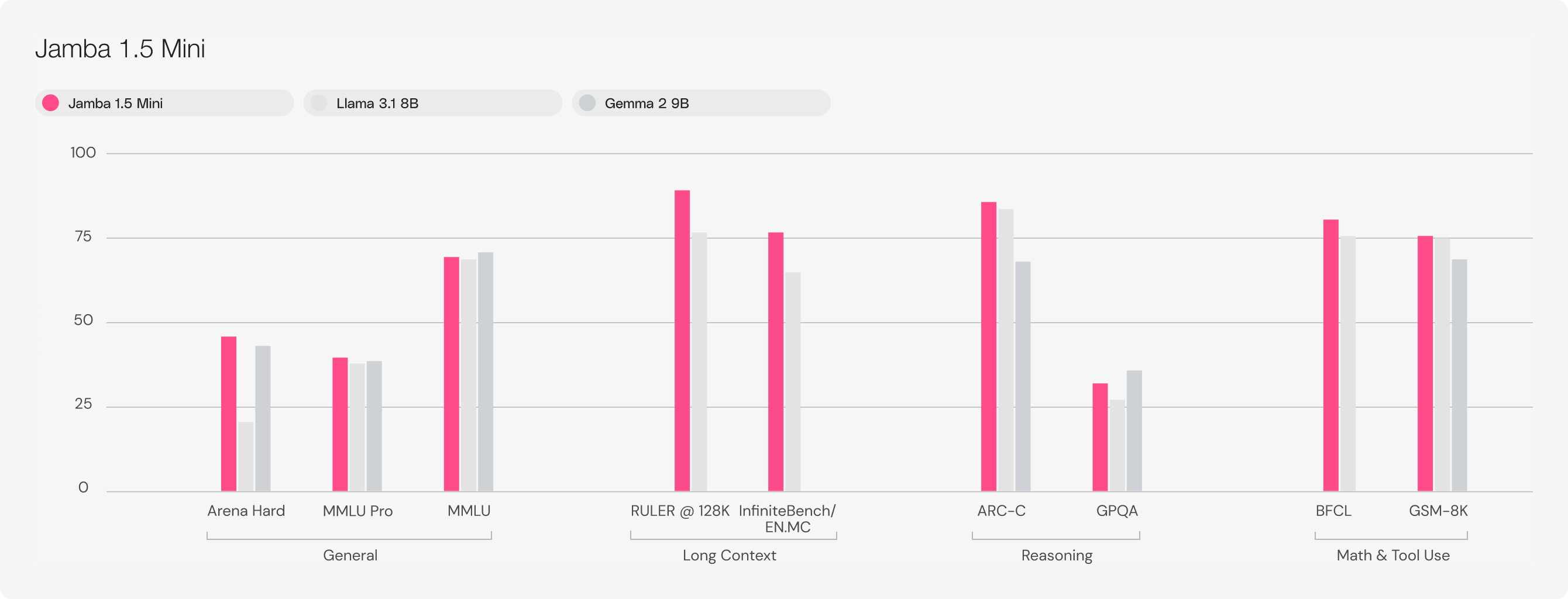
Jamba 1.5 Mini is the strongest open model in its size class with a score of 46.1 on the Arena Hard benchmark, surpassing larger models like Mixtral 8x22B and Command-R+. Jamba 1.5 Large, with a score of 65.4, outpaces both Llama 3.1 70B and 405B.
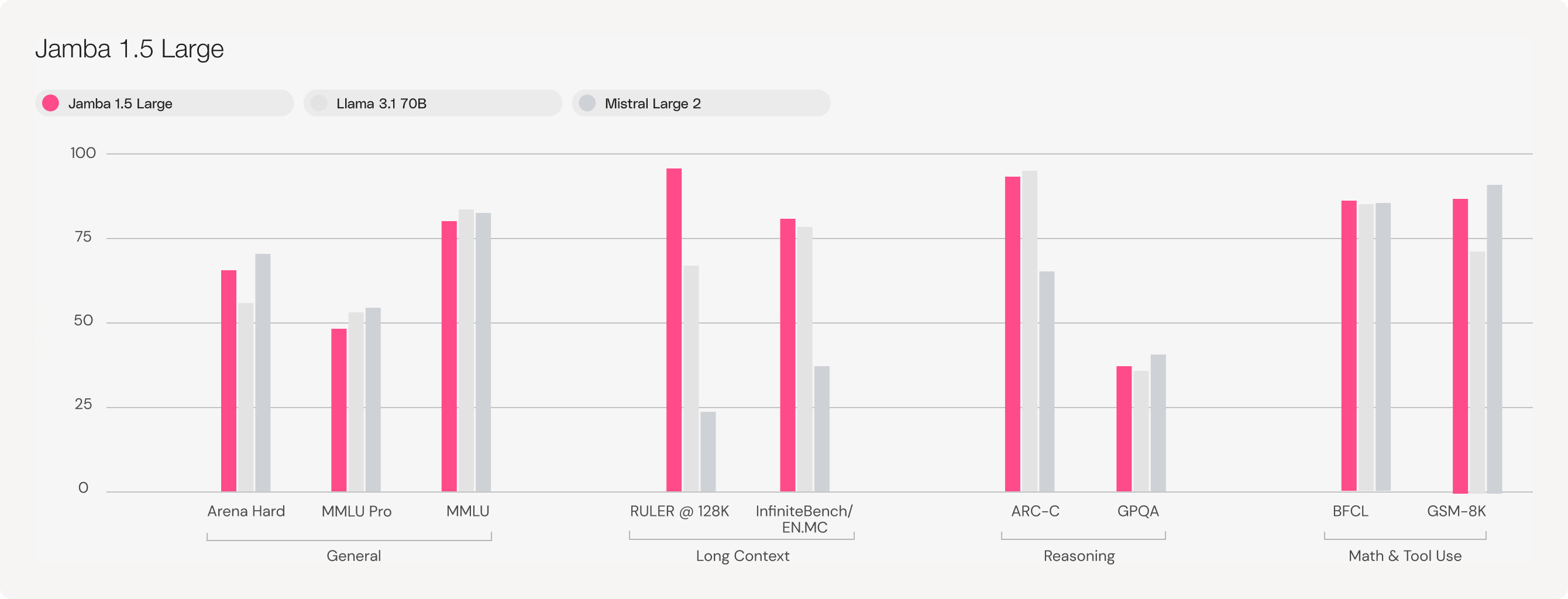
Both models demonstrate superior speed, with Jamba 1.5 Large outperforming competitors like Llama 3.1 70B and Mistral Large 2 in end-to-end latency tests at 10K contexts. For large context windows, it proved twice as fast as competitive models.
The Jamba 1.5 models are designed with developers in mind, supporting features such as function calling, JSON mode, and structured document objects. This makes them particularly well-suited for building Agentic AI systems and Retrieval-Augmented Generation workflows.
The hybrid architecture underlying these models uses a combination of Mamba and Transformer layers to manage long contexts more efficiently. This approach, combined with AI21’s proprietary ExpertsInt8 quantization technique, allows Jamba models to maintain high performance without sacrificing memory or speed—making them resource-efficient even with large-scale deployments.
The Jamba 1.5 models are available on multiple platforms, including AI21 Studio, Google Cloud Vertex AI, Microsoft Azure, and NVIDIA NIM, with plans for broader distribution across Amazon Bedrock, Databricks, and other major providers. The models are also available for private on-prem deployments. This accessibility should allow developers to quickly integrate these models into their systems with ease, using familiar tools like LangChain and Hugging Face.