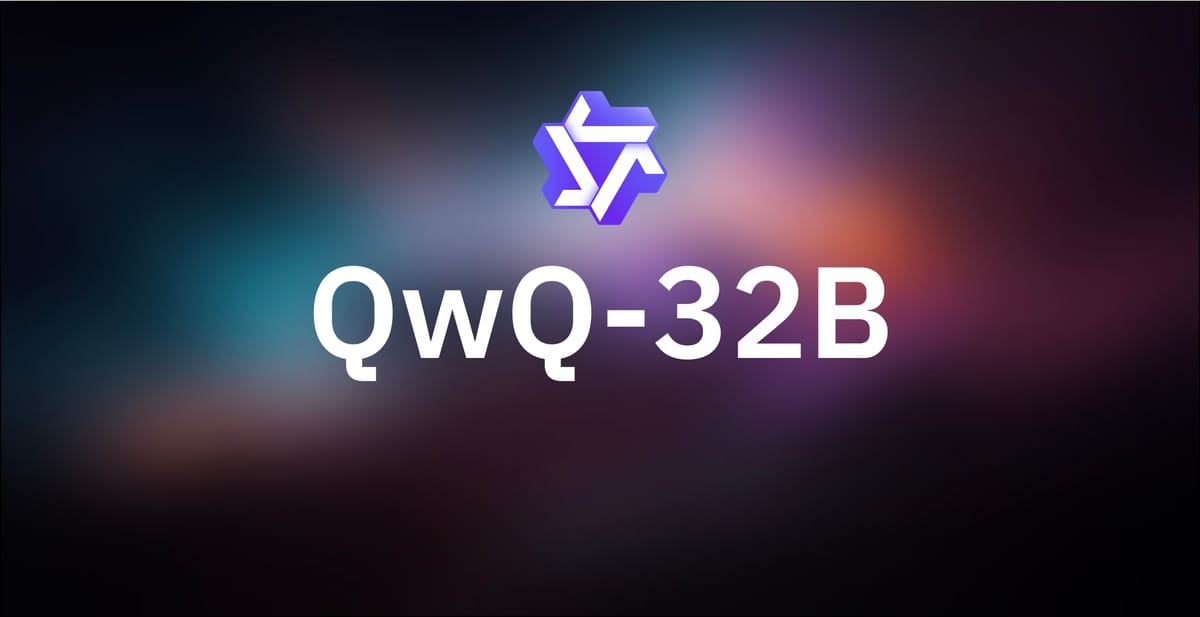
Qwen, Alibaba’s AI research arm, has introduced QwQ-32B, a 32-billion-parameter reasoning model that surpasses much larger rivals in key problem-solving tasks. Despite being significantly smaller than DeepSeek-R1 (671B parameters), QwQ-32B delivers superior performance in math, coding, and scientific reasoning, thanks to a multi-stage reinforcement learning (RL) training approach. The model is now available as open-weight on Hugging Face and ModelScope under an Apache 2.0 license.
Key Points:
- QwQ-32B uses multi-stage RL to refine its reasoning, improving performance beyond traditional pretraining methods.
- Despite having 32B parameters, it outperforms DeepSeek-R1 (671B) and OpenAI o1-mini in math and scientific reasoning.
- Available on Hugging Face and ModelScope under Apache 2.0, allowing enterprises to customize and deploy it.
- Requires significantly less hardware than DeepSeek-R1 while maintaining competitive performance.
The key innovation behind QwQ-32B is its multi-stage RL training process. Initially, the model is fine-tuned for math and coding using an accuracy verifier and a code execution server to validate outputs. Then, a second phase of RL improves general reasoning, instruction-following, and alignment with human preferences—without compromising its specialized strengths.
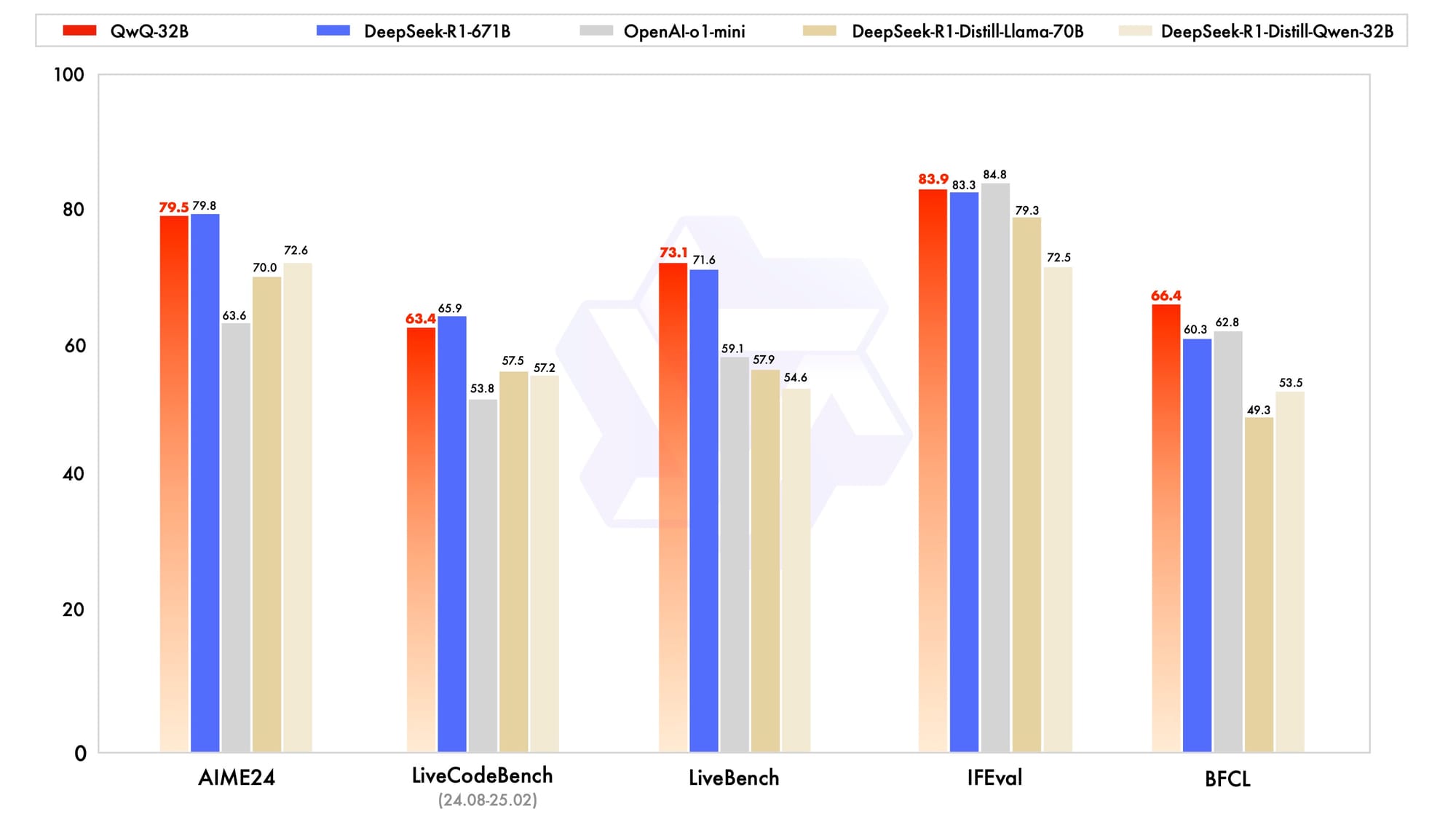
Benchmarks indicate that QwQ-32B outperforms OpenAI’s o1-mini and even beats DeepSeek-R1 in several categories, despite being 20 times smaller. Its extended 131,000-token context length further enhances its ability to handle long-form inputs, making it competitive with OpenAI and Google’s latest models.
Beyond performance, QwQ-32B offers practical advantages for enterprises. Firstly, it is open-weight under an Apache 2.0 license, allowing businesses to fine-tune and deploy it without restrictions. Secondly, it requires significantly less computational power—running on a single high-end GPU, compared to the multi-GPU setup needed for DeepSeek-R1.
The Qwen Team views QwQ-32B as just the beginning of their reinforcement learning journey. They plan to further explore RL scaling, integrate agents with RL for long-horizon reasoning, and continue developing foundation models optimized for these approaches.