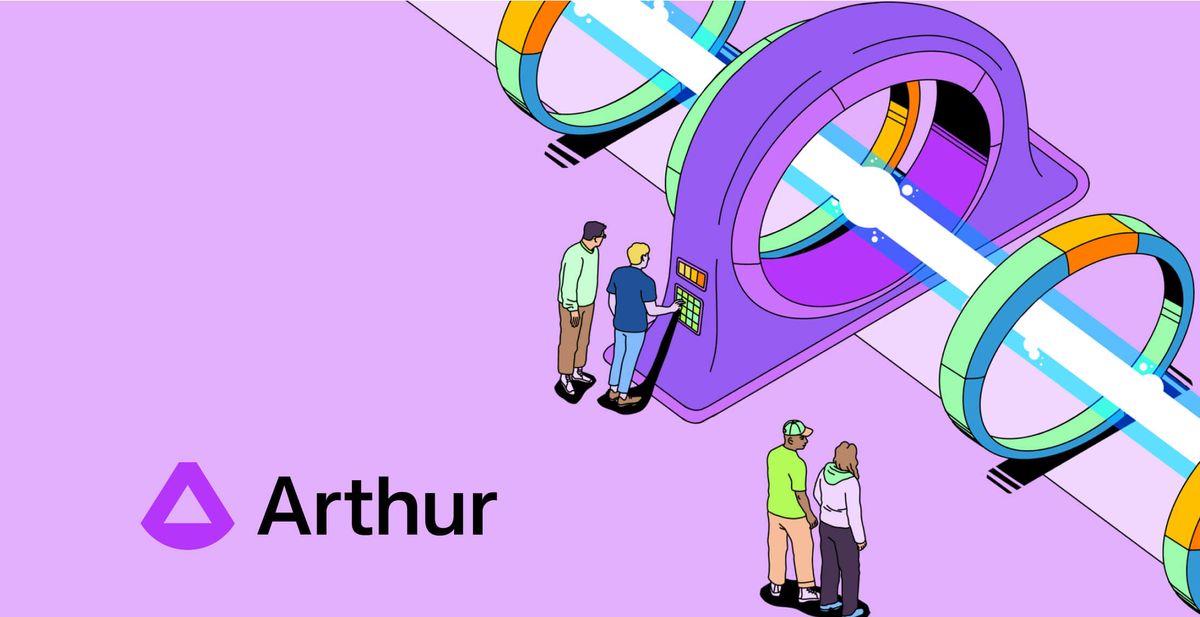
San Francisco-based startup Arthur today announced the launch of Arthur Bench, an open-source evaluation tool designed to help businesses compare the performance of large language models (LLMs) in real-world scenarios. With Bench, companies can test how different language models handle tasks relevant to their business needs, enabling data-driven decisions when integrating AI.
As AI and natural language technologies advance rapidly, businesses must constantly evaluate whether their chosen LLM solutions remain the optimal fit. However, it can prove challenging to translate academic benchmarks into assessments of real-world performance.
Arthur Bench aims to fill this gap by allowing customizable testing focused on metrics important to each business. Companies can evaluate criteria such as accuracy, fairness, content quality, and more across different LLMs using standardized prompts designed for business applications.
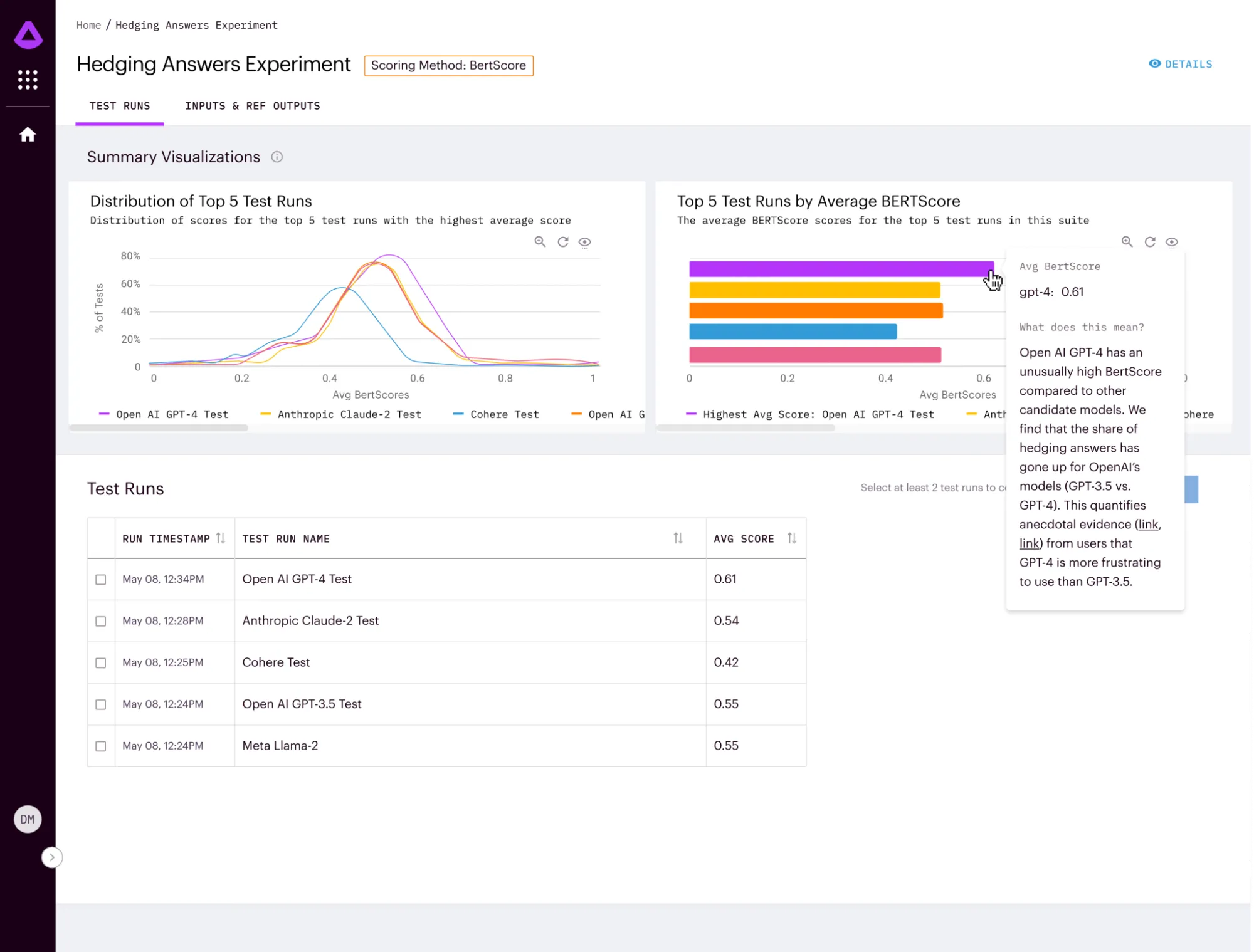
"The AI landscape evolves quickly, so constantly re-evaluating LLMs is crucial," said Arthur CEO Adam Wenchel. "Bench lets you deeply analyze differences between providers and models so you can determine the best fit for your needs."
In addition to model selection, Arthur touts benefits such as budget optimization, privacy controls, and translation of academic research into quantifiable business insights. The tool is positioned as part of a full suite of Arthur products for safely integrating LLMs.
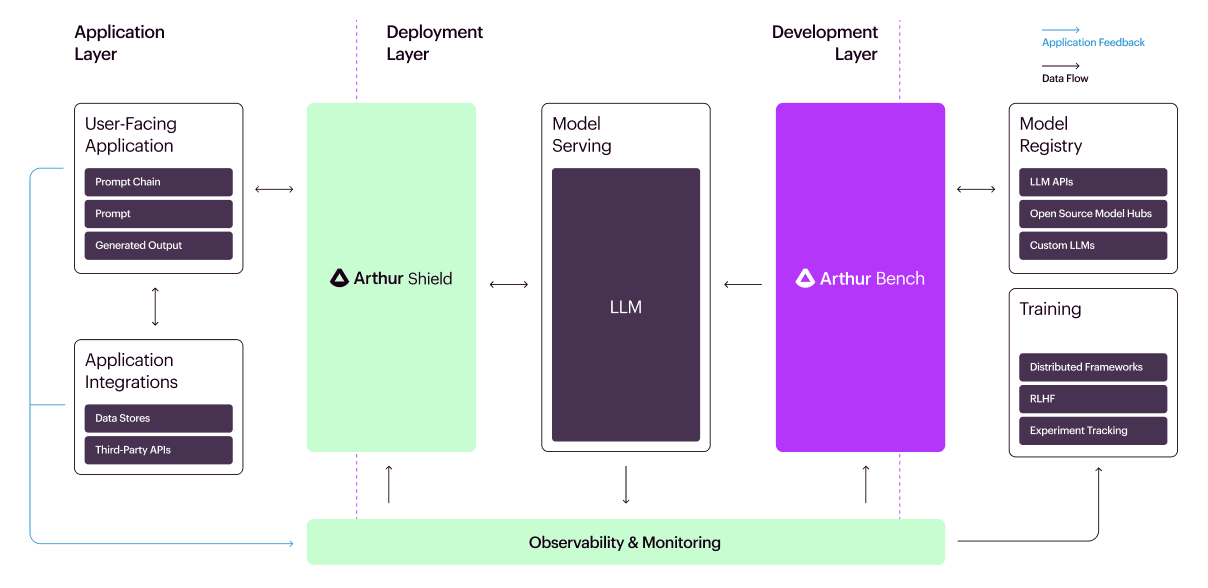
To spur Bench's development, Arthur also announced the Generative Assessment Project (GAP), an initiative to publicly share research on strengths, weaknesses, and best practices for major LLMs. GAP currently includes two research experiments that are well worth a read: the "Hallucination Experiment" and the "Hedging Answers Experiment".
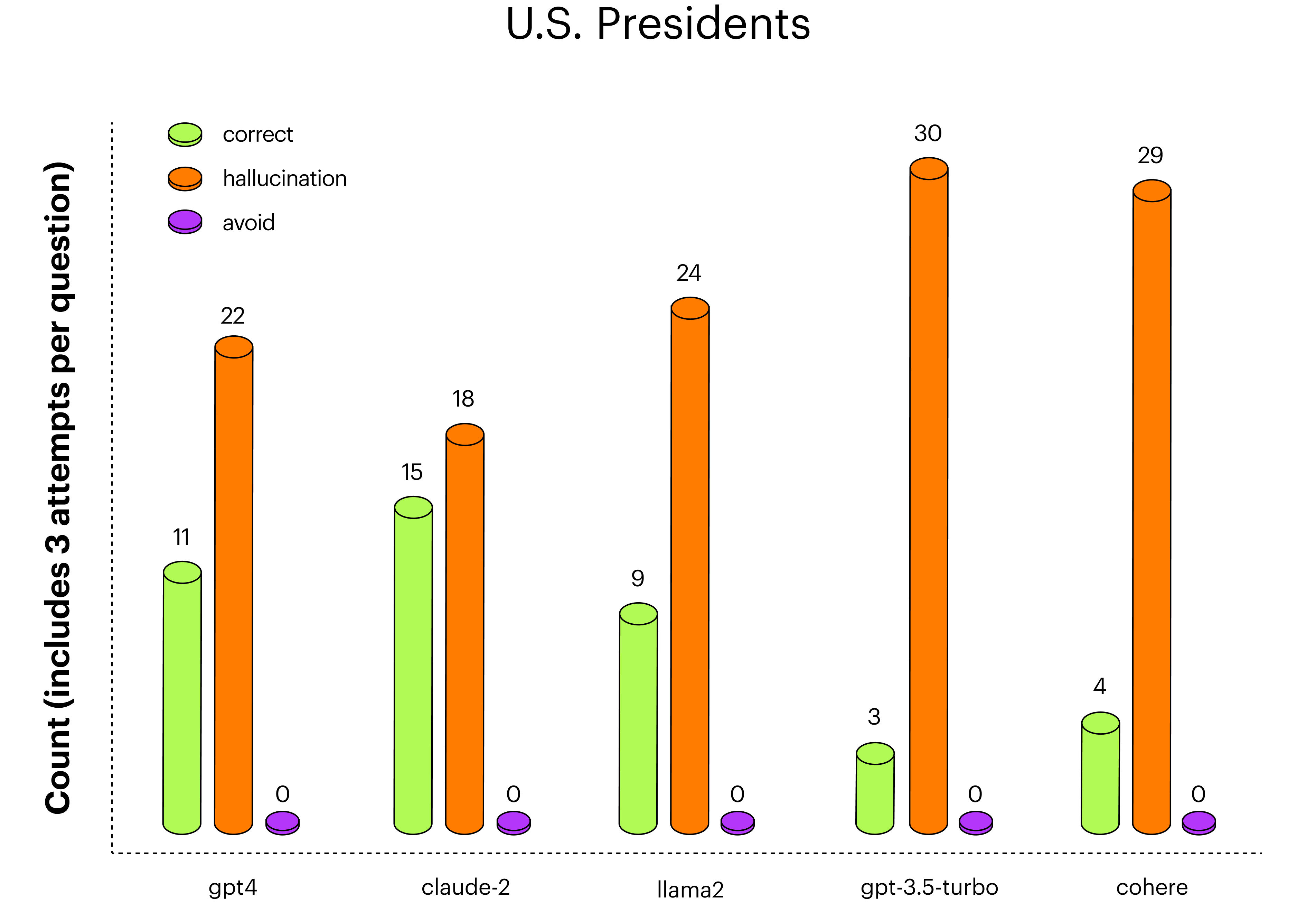
"Our GAP research shows nuances in LLM performance, so we built Bench to help teams analyze differences in a robust, standardized way," Wenchel explained.
By enabling customizable testing centered around metrics relevant to each business, Arthur Bench provides practical value beyond theoretical academic benchmarks. The tool's focus on quantifying performance differences also facilitates apples-to-apples comparisons between providers. This data-driven decision making allows businesses to select optimal LLMs for their unique needs.
Additionally, the open-source nature of Arthur Bench will allow it to continuously improve as more users contribute benchmarks and insights.