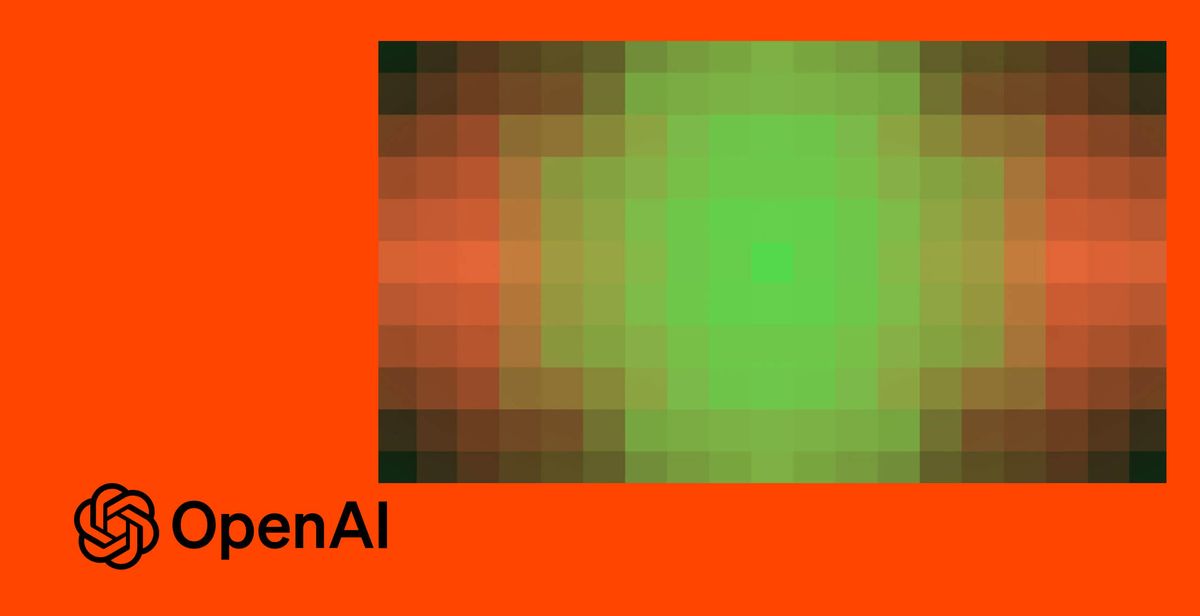
Researchers at OpenAI published a paper demonstrating that process supervision (providing feedback on each step of reasoning) leads to far more reliable AI models than outcome supervision (only providing feedback on the final result). The work focused on mathematical reasoning, with models ranking solutions to problems from the challenging MATH dataset.
OpenAI's language models have often faced criticism and have been the butt of jokes over their poor mathematical abilities. While GPT-3.5 and GPT-4 showed significant improvements in language understanding, their math skills still left much to be desired. To build truly intelligent systems, mathematical reasoning is crucial, and flaws there undermine credibility in other areas. The company's latest research exploring the application of process supervision to mathematical reasoning however stands to address this issue and impact far more than just their models’ math skills.
Their approach involved generating a multitude of potential solutions for each problem using a single fixed model (the generator), then evaluating each reward model's ability to perform a best-of-N search. The intriguing result here is that not only does the process-supervised reward model consistently outperform its outcome-supervised peer, but this gap in performance also amplifies as more solutions are assessed for each problem. This clearly underscores the superior reliability of the process-supervised model in this mathematical proving ground.

Outcome supervision only provides feedback on whether the final answer is right or wrong. As a result, models can develop unreliable reasoning that happens to reach the correct result. Process supervision addresses this by giving precise feedback on each step, directly training models to follow logical reasoning endorsed by humans. This helps ensure models do not develop undesirable behaviors, even if they achieve good outcomes.
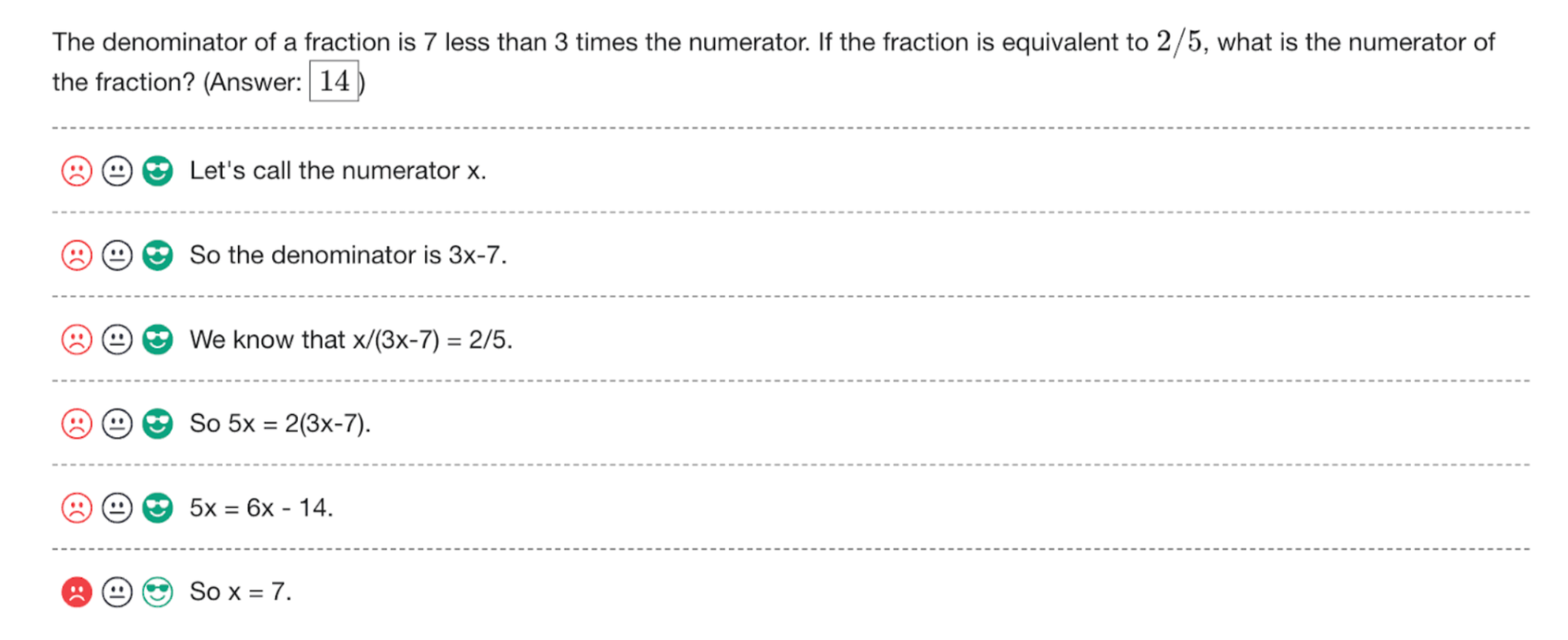
Beyond performance gains, process supervision has key benefits for AI alignment. It produces more interpretable solutions by encouraging step-by-step reasoning. It also avoids rewarding models that use incorrect logic but guess the right answer. The researchers suggest process supervision could mitigate hallucinations and logical mistakes in models.
By focusing models on logical reasoning steps that people can follow and trust, process supervision may yield AI with fundamentally sound judgment—the kind of judgment that anchors human thinking and allows for open-ended learning. Rather than criticisms after the fact, or hoping for the "right" outcomes, this technique helps ensure models develop reasoning ability and fair judgment from the start.
OpenAI has also released their full dataset of 800,000 step-level labels to encourage further work on process supervision and reliable reasoning models. Their results so far are promising and suggest process supervision could lead to AI systems that are both highly capable and aligned with human reasoning. If these findings generalize beyond math problems, process supervision may be key to developing trustworthy AI for complex, multi-step domains.