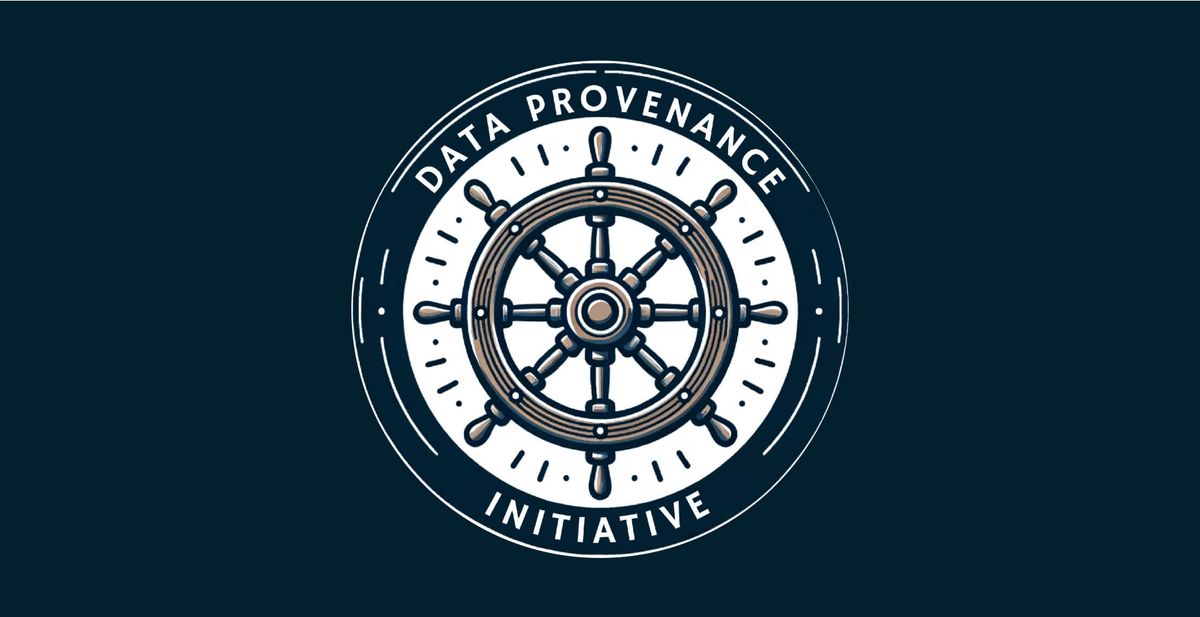
A coalition of leading AI researchers has taken a major step toward addressing the growing crisis around data transparency in artificial intelligence.
Earlier this week, an interdisciplinary team from institutions including MIT and Cohere For AI unveiled the Data Provenance Explorer, a platform that for the first time allows users to easily track and filter nearly 2,000 popular AI datasets based on criteria such as licensing, attribution, and other ethical considerations.
The launch comes amid rising concern over the origins and stewardship of data used to train AI models, particularly large language models that have demonstrated impressive but sometimes unpredictable capabilities.
In a newly published paper, the group highlighted the systemic issues plague current data provenance practices. Through an extensive audit, the researchers found high rates of missing or incorrect licensing information in widely used datasets sourced from crowdsourced aggregators like GitHub.
The initiative represents the most extensive audit of AI datasets to date, equipping them with tags that link back to original data sources, capturing re-licensing instances, creators, and other essential data properties.
This lack of transparency creates cascading risks, allowing datasets to be repackaged and shared under licenses that differ from the original authors' intent. It also leaves developers unable to responsibly attribute data sources.
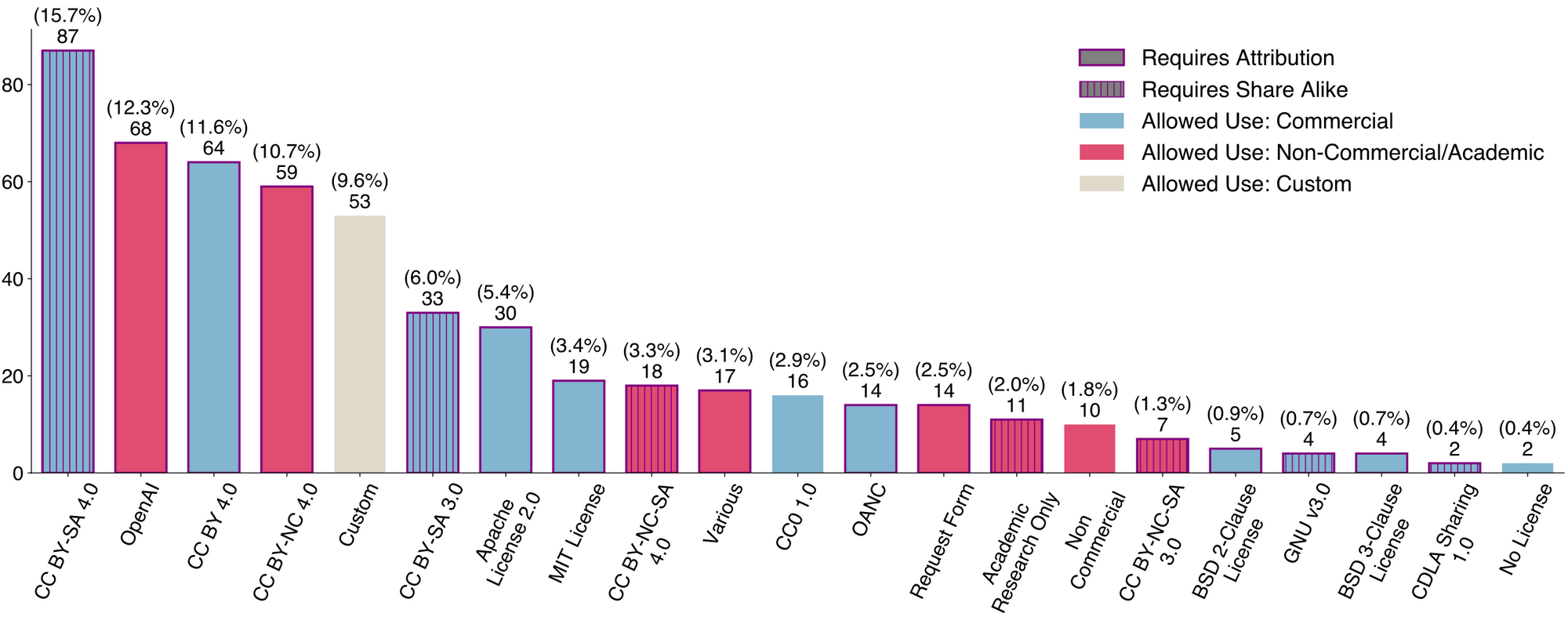
Beyond licensing, the audit revealed other problematic trends in publicly available datasets. Data permitting commercial use appears to be declining, even as demand rises from AI startups. Additionally, geographical diversity is sorely lacking, with Western-centric data dominating at the expense of other global regions.
While the new Data Provenance Explorer promotes transparency around existing datasets, the researchers acknowledge it cannot resolve fundamental legal ambiguities that complicate responsible data use. License applicability across jurisdictions, conflicts between licenses in bundled datasets, and the common misuse of software licenses for data remain open challenges.
Nonetheless, by aggregating and structuring information on thousands of datasets, the Explorer represents an important leap forward. Developers finally have a reliable resource to inform ethical and legal considerations in data selection. More broadly, it lays the groundwork for wider collaboration to enhance transparency and provenance standards in the AI community.