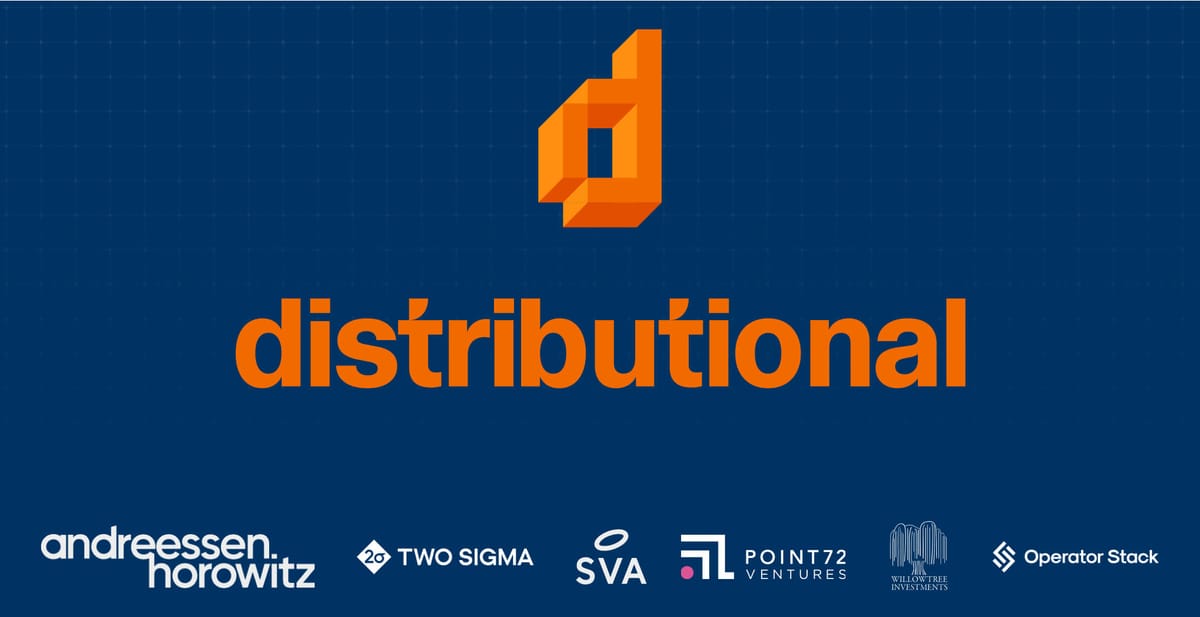
Artificial intelligence startup Distributional announced it has secured $11 million in seed funding to develop a testing and evaluation platform aimed at making AI systems safer, more secure, and more reliable for enterprise use cases.
The seed round was led by Andreessen Horowitz, with participation from Operator Stack, Point72 Ventures, SV Angel, Two Sigma, Willowtree Investments, and dozens of AI industry leaders.
As AI models grow more complex, unpredictable and prone to issues like instability and inaccuracy, robust testing methodologies are needed to identify and resolve risks before deployment. However, many enterprises currently rely on ad-hoc, incomplete testing approaches.
Distributional CEO Scott Clark has directly experienced AI testing challenges at companies like Yelp, SigOpt (acquired by Intel in 2020), and Intel, where he led a 100-person AI and HPC engineering team.
"I learned that to robustly test AI I needed to evaluate distributions of outcomes and that there is no purpose-built software for this task," said Clark.
"Lack of reliability in AI systems is one of the biggest barriers to widespread enterprise adoption," said Martin Casado, General Partner at Andreessen Horowitz. "We are excited for Distributional to address this problem by building a platform for robust and repeatable AI testing."
Most enterprises today either rely on manual testing, passive monitoring, or avoid deploying AI altogether due to uncertainty around model performance. Distributional's platform will provide continuous insights into risks across statistical models, machine learning systems, natural language models, and other AI types.
The founding team of 12 has experience building AI systems at companies like Google, Meta, Uber, Bloomberg, Intel and more. Distributional plans to launch its enterprise product line in 2024 to help financial services, manufacturing, energy, and other industries deploy AI more confidently.
By empowering enterprises to proactively evaluate AI model performance, Distributional aims to increase real-world AI adoption by enabling companies to catch issues before they negatively impact end users. More rigorous testing methodologies are imperative as AI systems grow more complex and critical across industries.