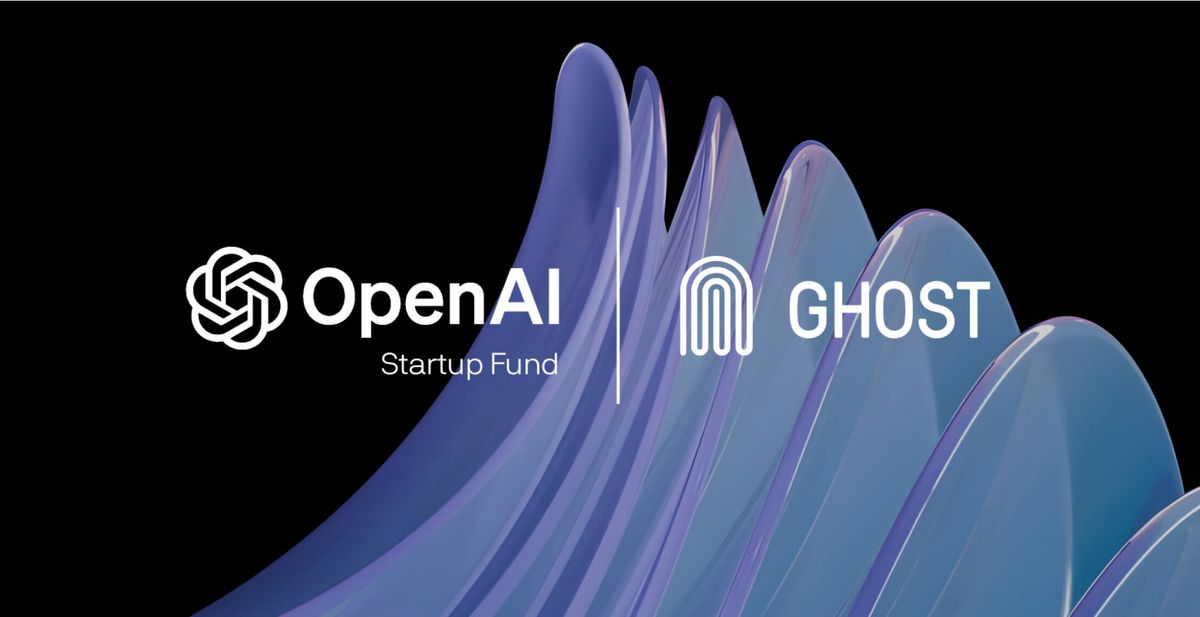
San Francisco-based Ghost Autonomy, a startup working on scalable autonomous driving software, announced that they have secured a $5 million investment from OpenAI’s startup fund. The injection of capital will accelerate Ghost’s research applying large language models (LLMs) and multi-modal LLMs to enable more robust urban autonomous driving capabilities.
The new investment brings Ghost’s total funding to $220 million as it works to bring LLM-powered intelligence to major automakers. OpenAI’s COO Brad Lightcap said multi-modal models have “the potential to expand the applicability of LLMs to many new use cases including autonomy and automotive.” By combining diverse inputs like video, images and audio, multi-modal LLMs may better understand complex driving scenarios.
Ghost believes LLMs represent a potential breakthrough in solving urban autonomy’s toughest challenges. Today's single-purpose neural networks powering self-driving cars have limitations in reasoning about novel, unusual situations. In contrast, LLMs like OpenAI's GPT-4 leverage broad world knowledge to make human-like inferences about novel inputs. This could let autonomous vehicles handle edge cases via true scene understanding, not just pattern recognition.
Ghost is actively testing these capabilities by feeding visual driving data captured by its fleet of vehicles to LLMs online. The startup believes fine-tuning and customizing LLMs specifically for autonomous driving will further unlock their potential. This includes on-road testing and validation to improve performance and safety.
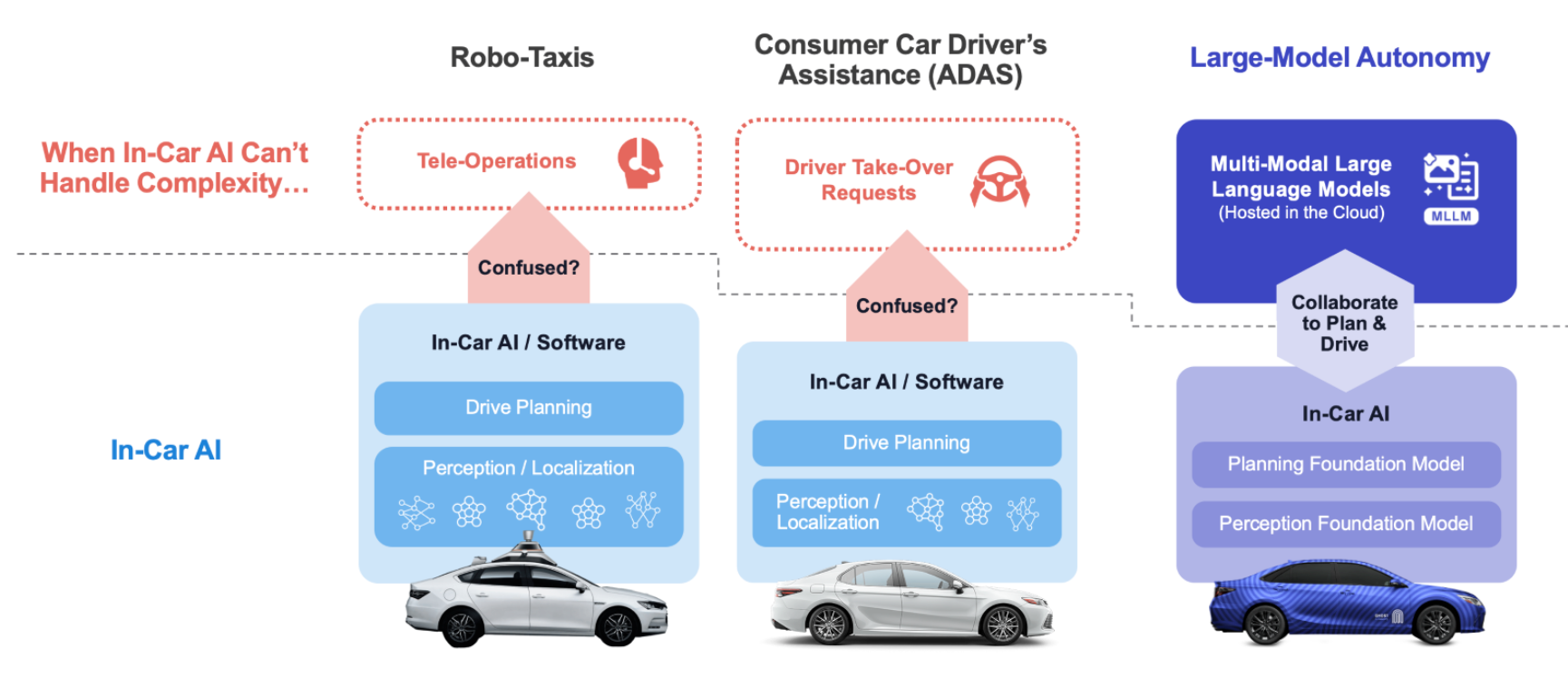
The potential of MLLMs to transform the way vehicles perceive and react to their surroundings is evident. For instance, while traditional AI systems excel in recognizing trained objects or deciphering signs via OCR, MLLMs can holistically assess a scene and make nuanced decisions based on a comprehensive scene analysis. Whether it's navigating through erratic construction zones, interpreting complex road rules, or maneuvering in densely populated urban settings, MLLMs promise a level of interpretation and adaptability that far exceeds the capabilities of conventional AI systems.
The company sees multi-modal LLMs enabling a rethinking of the entire autonomy software stack. Rather than today’s complex array of individual AI tools for perception, planning and control, LLMs could provide a more end-to-end solution. A large model trained holistically on world knowledge could potentially perform sophisticated reasoning from perception inputs to driving outputs in one integrated step.
However, the implementation of such advanced MLLMs presents architectural challenges, particularly in adapting them for in-vehicle use. Ghost believes a hybrid architecture with collaboration between cloud and in-car systems will be required. The cloud would run heavyweight LLMs, while embedded systems handle real-time operations. Work is still needed to build and validate this approach, but LLMs are already improving offline autonomy processes like data labeling.
While the journey towards a fully MLLM-driven autonomy stack is still in progress, Ghost Autonomy is already leveraging MLLMs to refine the way data is processed, labeled, and simulated for autonomous driving systems. The company is actively inviting collaboration with automakers to pioneer the integration of MLLM reasoning and intelligence into advanced driver-assistance systems.