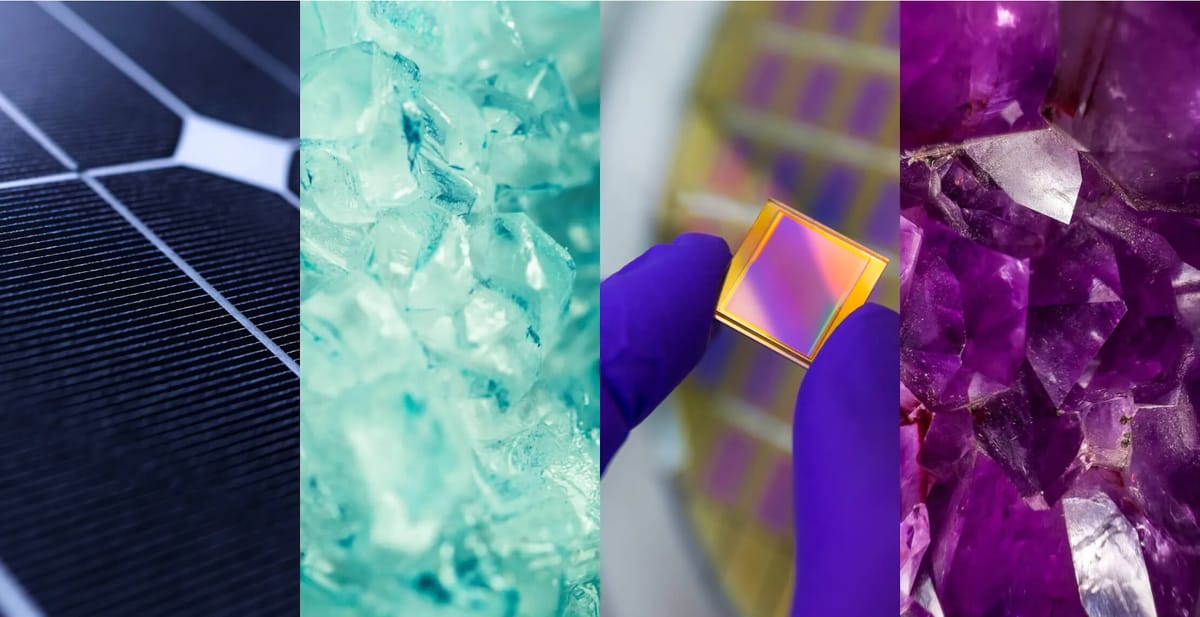
Google DeepMind has unveiled a groundbreaking new AI tool called GNoME (Graph Networks for Materials Exploration) that has discovered 2.2 million new stable crystals, greatly expanding the number of known viable materials that could enable development of new technologies.
The discovery process employed by GNoME is a testament to the power of AI in scientific exploration. The tool uses two pipelines for discovering low-energy (stable) materials: a structural pipeline that creates candidates with structures similar to known crystals and a compositional pipeline that follows a more randomized approach based on chemical formulas. The predictions from these pipelines are then evaluated using established Density Functional Theory calculations, feeding into an iterative cycle of active learning.
Of the 2.2 million materials found, 380,000 are predicted to be the most stable and therefore the top candidates for experimental synthesis in labs. This represent a more than eightfold increase over previously known stable inorganic crystals.
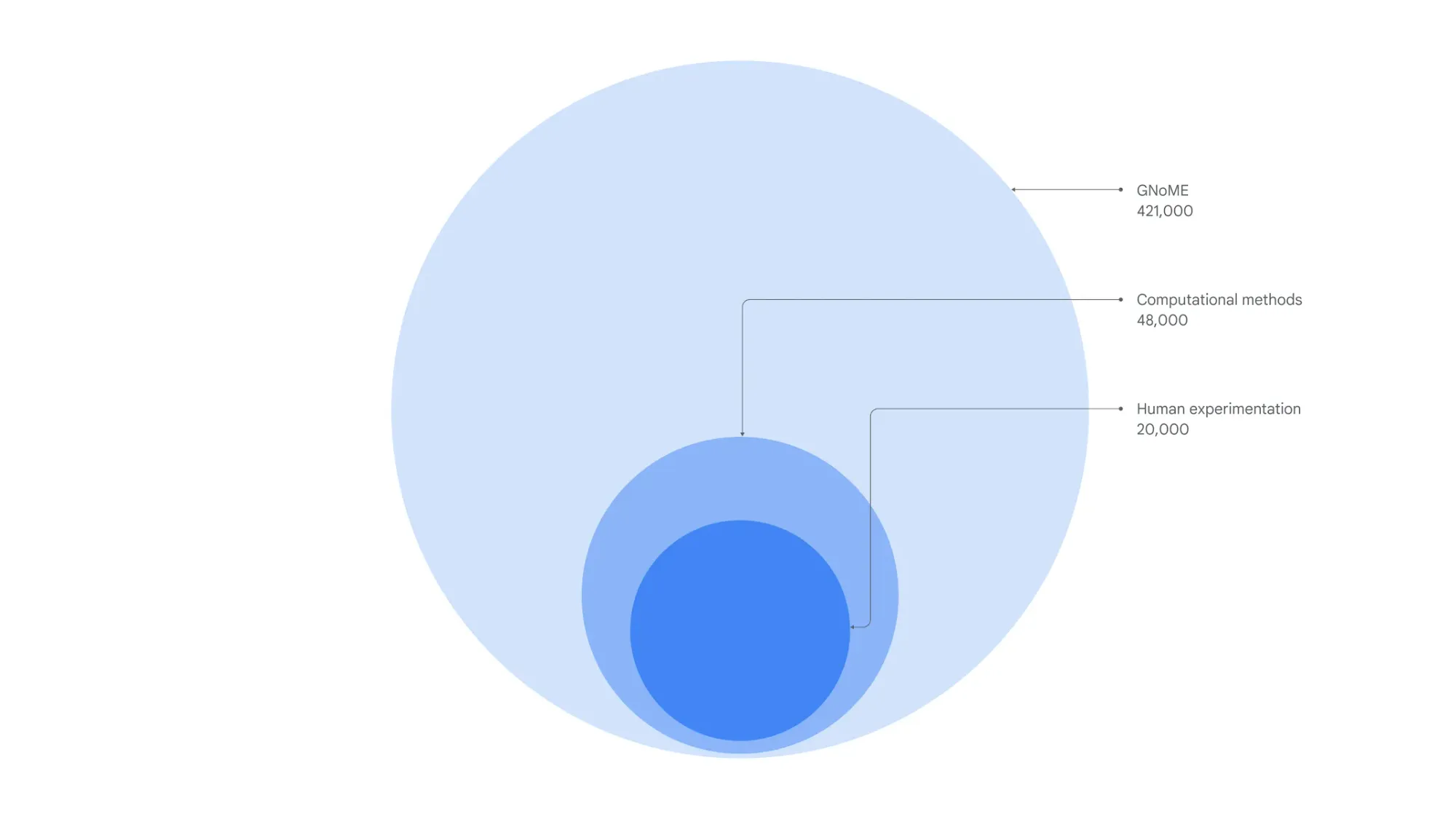
Among the newly discovered materials are potential superconductors that could advance computing, improved lithium ion conductors that might boost battery performance in electric vehicles, and over 50,000 layered compounds with similarities to wonder material graphene.
At the core of this breakthrough is GNoME, DeepMind’s custom graph neural network architecture tailored to materials discovery by leveraging data on atomic configurations and stability of known crystals. Through an iterative process the AI rapidly evaluates candidate materials, expanding its knowledge to make ever more accurate predictions.
In effect, GNoME learned to expertly navigate the enormous search space of possible stable inorganic crystals, overcoming limitations that stymied traditional trial-and-error approaches requiring months of experimentation per material. This demonstrates the power of AI to transform the efficiency of scientific discovery.
The potential applications of these materials are vast and varied. For instance, the discovery includes 52,000 new layered compounds similar to graphene, which could revolutionize electronics, and 528 potential lithium-ion conductors that could enhance battery performance.
The research team has contributed the 380,000 predicted stable materials to the Materials Project database for public access, hoping to accelerate research and development of new technologies dependent on such materials.
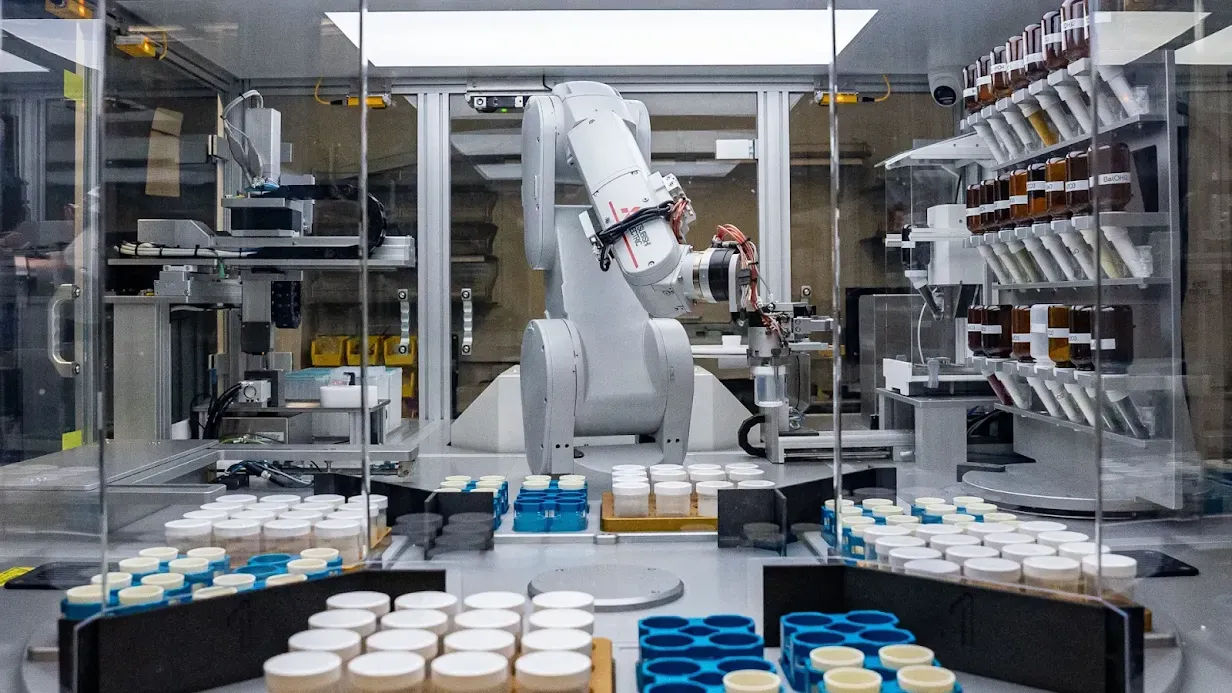
In a parallel study, DeepMind collaborated with the Lawrence Berkeley National Laboratory to showcase autonomous robotic synthesis guided by the materials insights from GNoME. Successfully creating over 40 new predicted crystals proves viability for AI-driven closed loop materials discovery and production.
While further research is still required on manufacturing and properties, DeepMind's work represents a watershed moment for intelligently exploring and designing new inorganic materials with transformational applications. Their research points towards AI playing an increasingly valuable role partnering with scientists to develop solutions for a sustainable future.