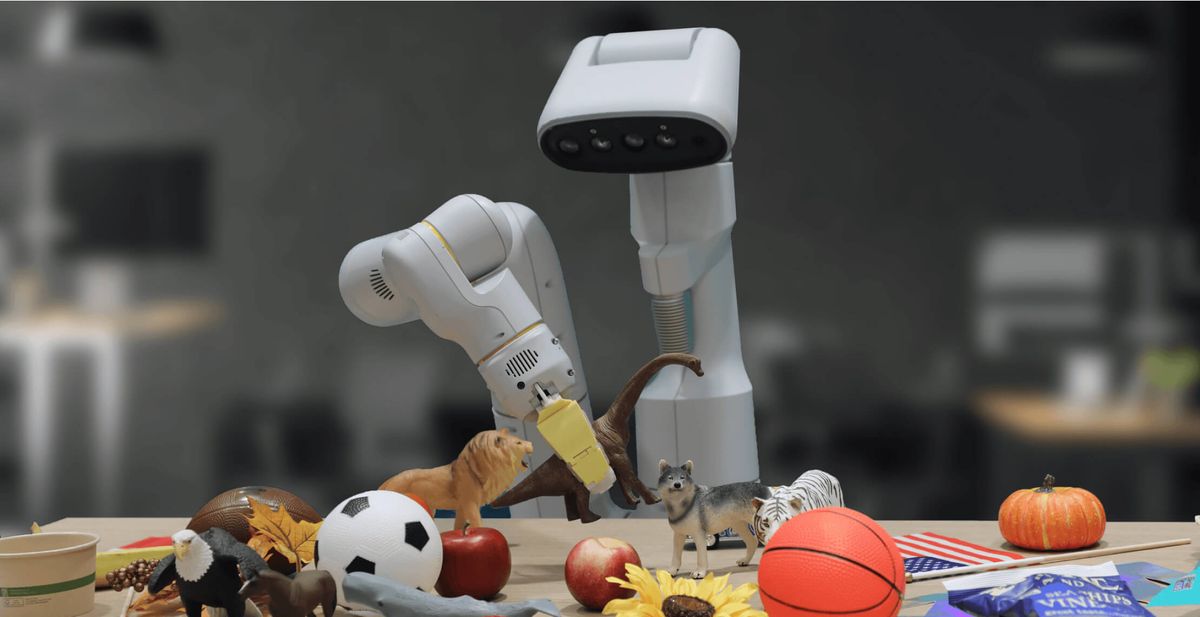
Google DeepMind has taken a major leap forward in artificial intelligence for robotics with the introduction of Robotic Transformer 2 (RT-2), a first-of-its-kind vision-language-action model. The new system demonstrates unprecedented ability to translate visual inputs and natural language commands directly into robotic actions, even for novel situations never seen during training.
As described in a new paper published by DeepMind, RT-2 represents a breakthrough in enabling robots to apply knowledge and reasoning from large web datasets to real-world robotic tasks. The model is built using a transformer architecture, the same technique behind revolutionary large language models like GPT-4.
Historically, the path to creating useful, autonomous robots has been strewn with hurdles. Robots need to understand and interact with their environment, a feat requiring exhaustive training on billions of data points covering every conceivable object, task, and situation. This extensive process, both time-consuming and costly, has largely kept the dream of practical robotics within the realm of science fiction.
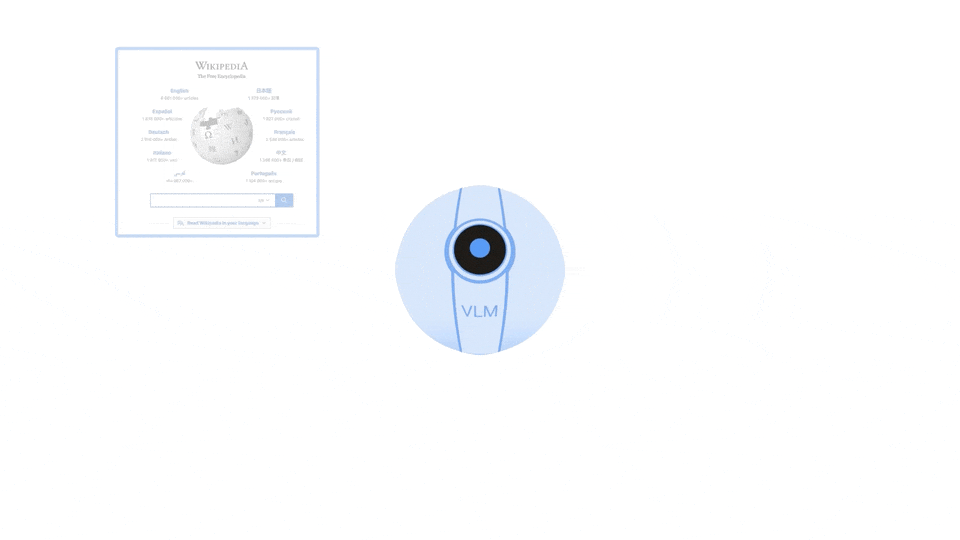
DeepMind's RT-2, however, represents a revolutionary new approach to this problem. Recent advancements have boosted the reasoning abilities of robots, allowing for chain-of-thought prompting, or the dissection of multi-step problems. Vision models such as PaLM-E have enhanced their understanding of surroundings, and previous models like RT-1 have demonstrated that Transformers can facilitate learning across diverse robot types.
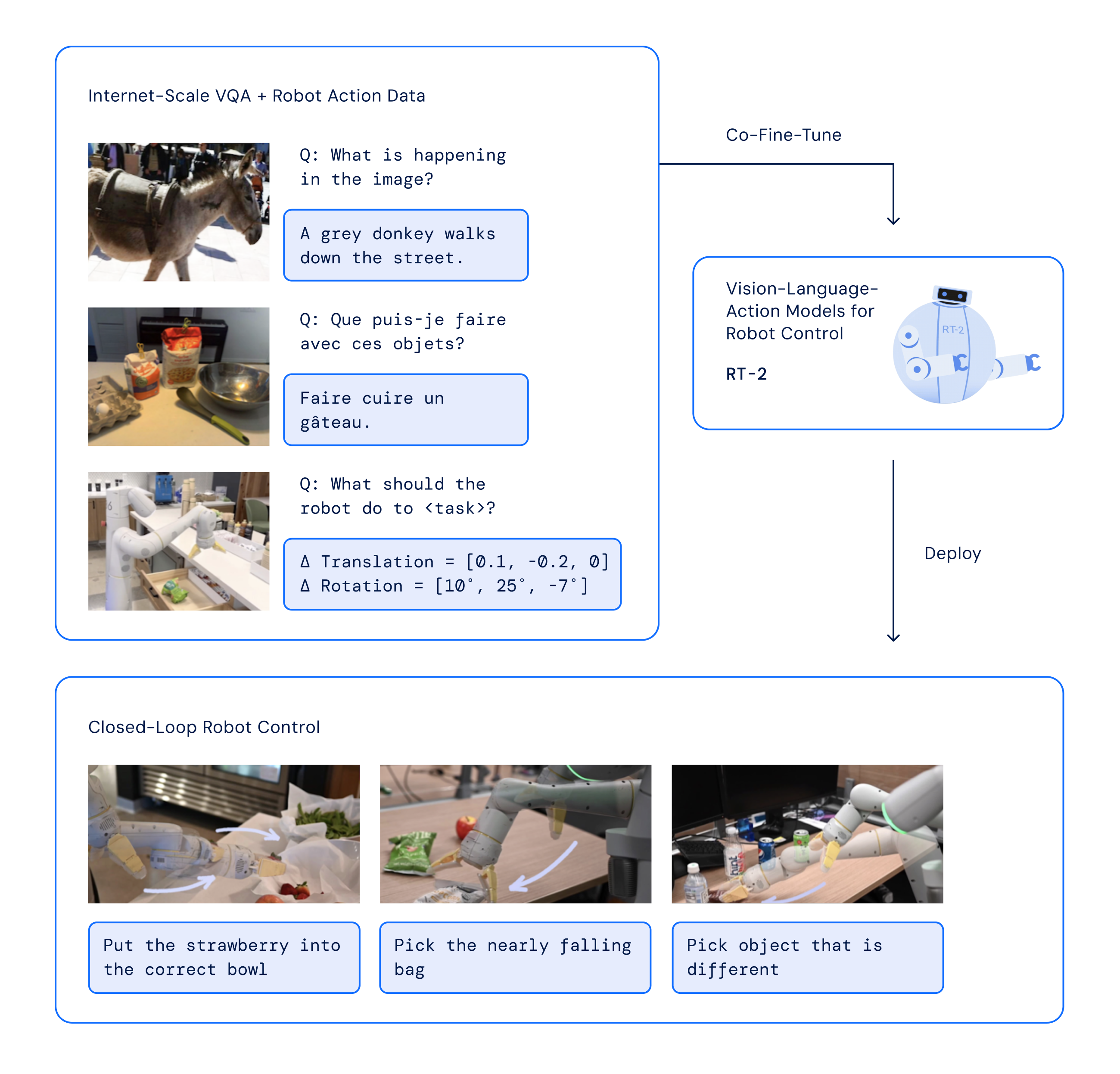
By leveraging the vast corpus of text, images and videos on the internet, RT-2 acquires a much broader understanding of concepts and tasks compared to previous robot learning systems reliant solely on physical trial-and-error. According to DeepMind, this allows RT-2 to exhibit intelligent behaviors such as using deductive reasoning, applying analogies, and displaying common sense when confronted with unfamiliar objects or scenarios.
For example, commands like “move banana to the sum of 2 plus 1” means the robot needs knowledge transfer from web pre-training 𝗮𝗻𝗱 showing skills not present in the robotics data.
The potential of RT-2 lies in its capability to quickly adapt to novel situations and environments. In over 6,000 robotic trials, RT-2 demonstrated its proficiency, equalling the performance of the previous model, RT-1, on familiar tasks and almost doubling its performance to 62% in unfamiliar, unseen scenarios. This development signifies that robots can now learn in a manner similar to humans, transferring learned concepts to new situations.
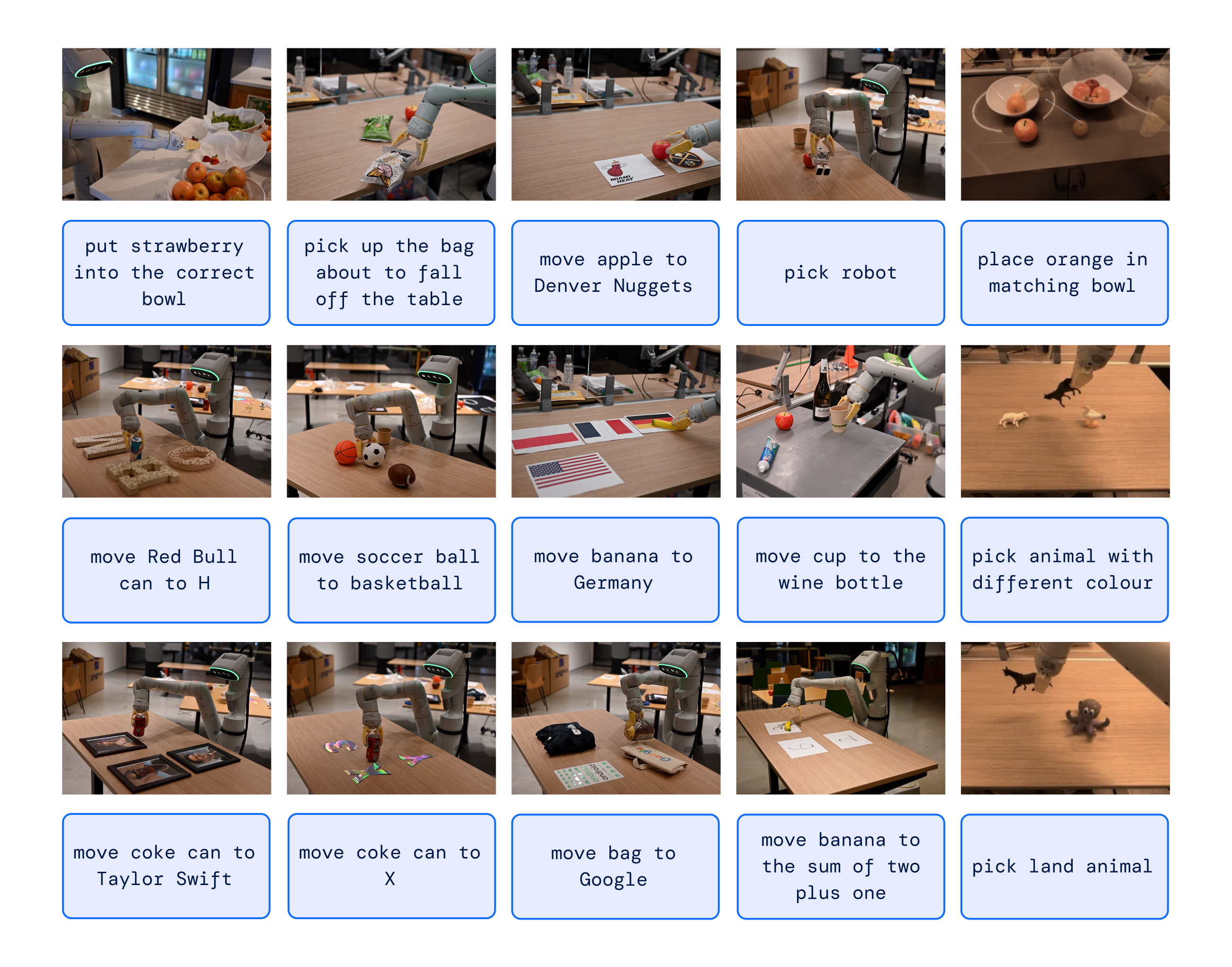
During testing, an RT-2 equipped robot was able to successfully interpret abstract commands like "discard the trash" without needing explicit training on identifying trash items or motions to throw them away. The robot was able to deduce the meaning and perform the task appropriately, displaying the type of adaptable general intelligence that has been a holy grail in the field.
RT-2 represents a paradigm shift away from robots requiring precise, step-by-step programming for every single object and scenario toward more flexible, learning-based approaches. While still far from perfect, its ability to acquire common sense and reasoning without direct experience moves us substantively toward the possibility of widely capable assistive robots.
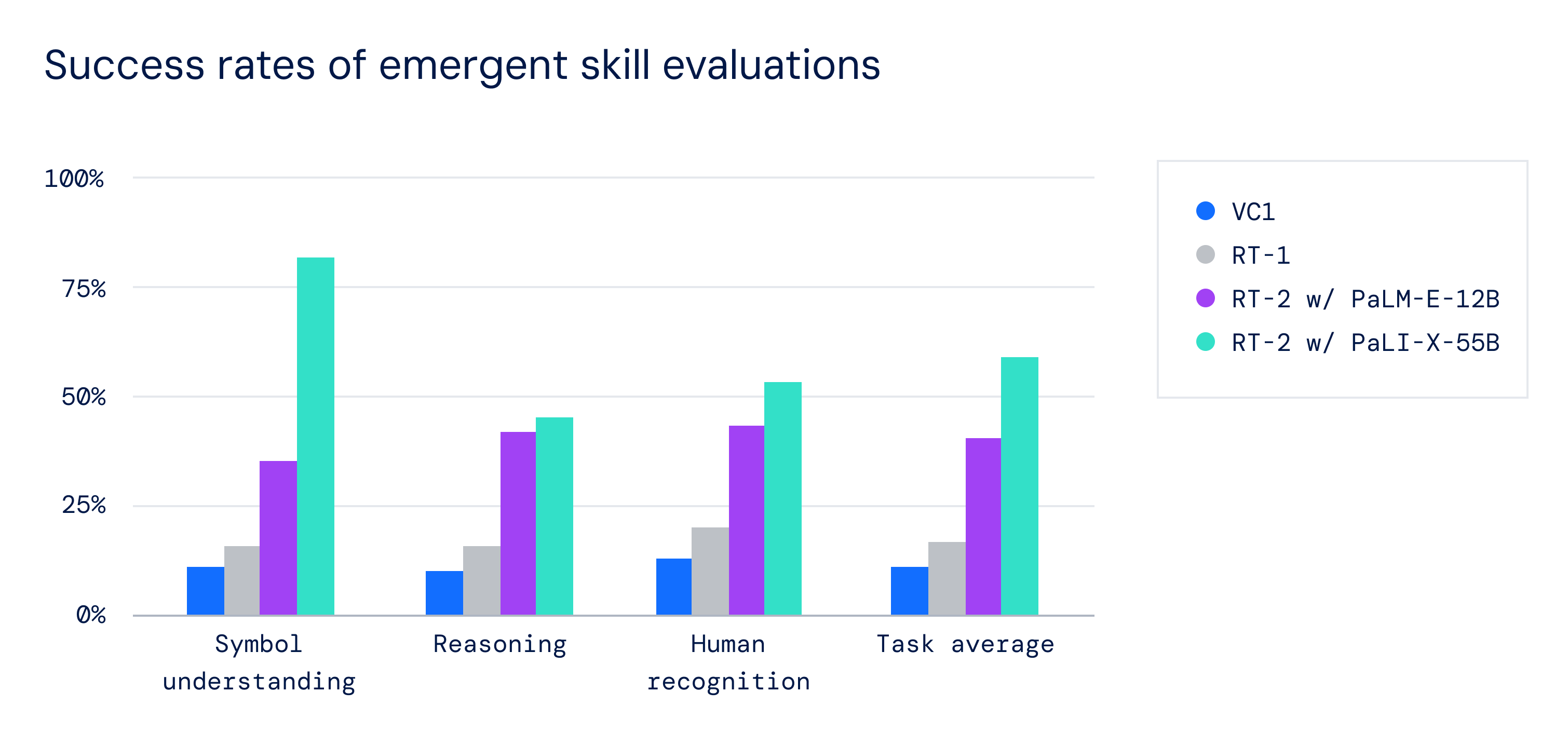
DeepMind notes there remains significant work to realize this goal fully, including improving the reliability and adaptability of RT-2's behaviors. But the implications are profound, suggesting future AI that can understand the nuances of our everyday physical world at a much deeper level.
As DeepMind pushes ahead with developing more capable and general robot learning systems, questions around responsible AI development are bound to arise. How can we ensure safety during training and deployment? Should robots have transparency around when they are operating in novel situations? What are the implications for employment as robots take on new skills?
Addressing these questions will require input from diverse voices beyond the tech world. But what seems clear is that with RT-2, DeepMind has opened the door to a new reality where AI-endowed robots begin permeating our homes, workplaces and daily lives. For better or worse, the age of thinking machines acting in our physical environment may arrive sooner than we realize.