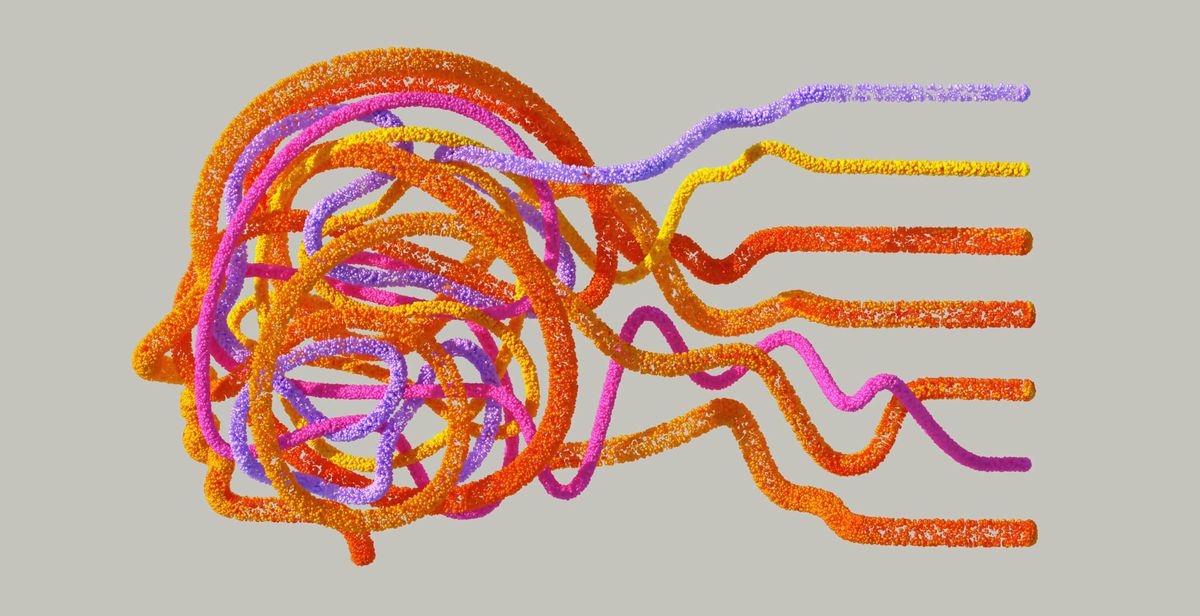
The rapid proliferation of AI technology into industries worldwide has been remarkable, but its integration into healthcare, a field where stakes are sky-high, has been met with reasonable caution. This is because any underperformance or errors from AI systems in medical applications could potentially put patients' health and lives at risk. But new research unveiled in a joint paper by Google DeepMind and Google Research proposes a promising solution.
The research presents Complementarity-driven Deferral-to-Clinical Workflow (CoDoC), an AI model that learns when to trust a predictive AI's diagnosis or defer to a clinician. This idea is essential for successfully integrating AI into areas like medical imaging, where predictive models are being rapidly adopted.
CoDoC represents an evolution in building more reliable AI systems. It is intended to be an add-on tool for human-AI collaboration. It does this not by re-engineering predictive models, which is an onerous task that most healthcare providers cannot accomplish, but by learning from these systems' decisions and accuracy when working with human clinicians. It was designed to meet three critical criteria:
- It should be deployable by non-machine learning experts such as healthcare providers
- Require only a small amount of data for training.
- Be compatible with proprietary AI models without needing access to their internal workings or training data.
To illustrate how it works, consider a clinician interpreting a chest x-ray to determine whether a tuberculosis test is necessary. CoDoC takes in three inputs for each case in the training dataset:
- The confidence score from the predictive AI model
- The clinician's interpretation
- The ground truth of whether disease was present.
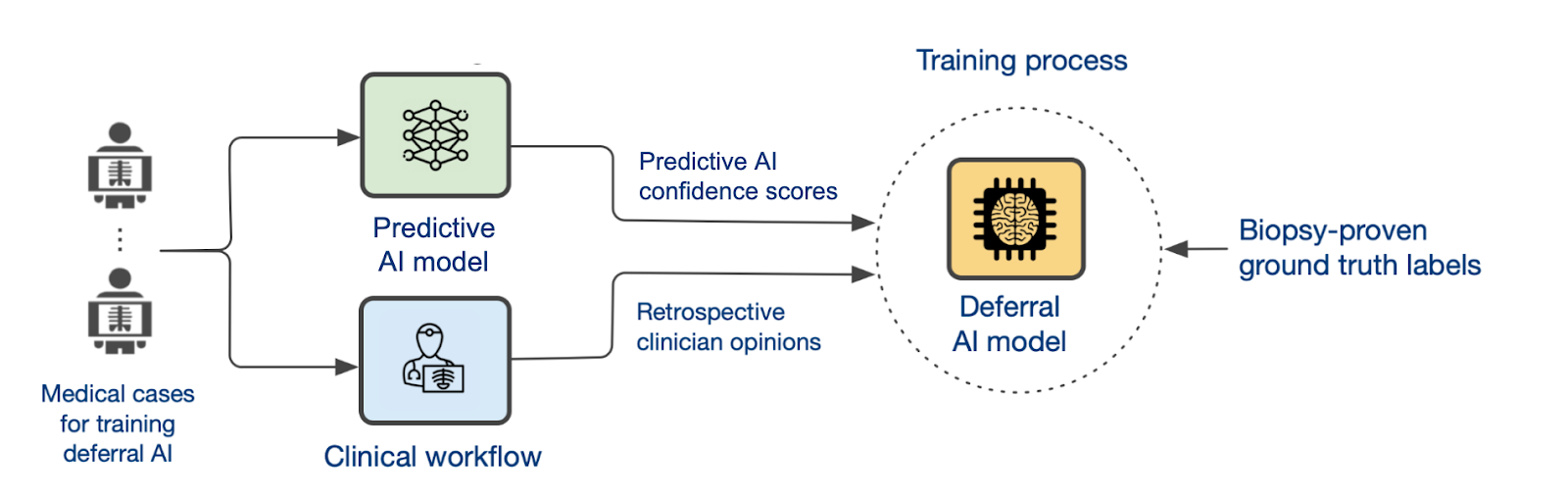
Then it learns to gauge the relative accuracy of the AI model compared with the clinician's interpretation, and how that relationship varies with the confidence score.
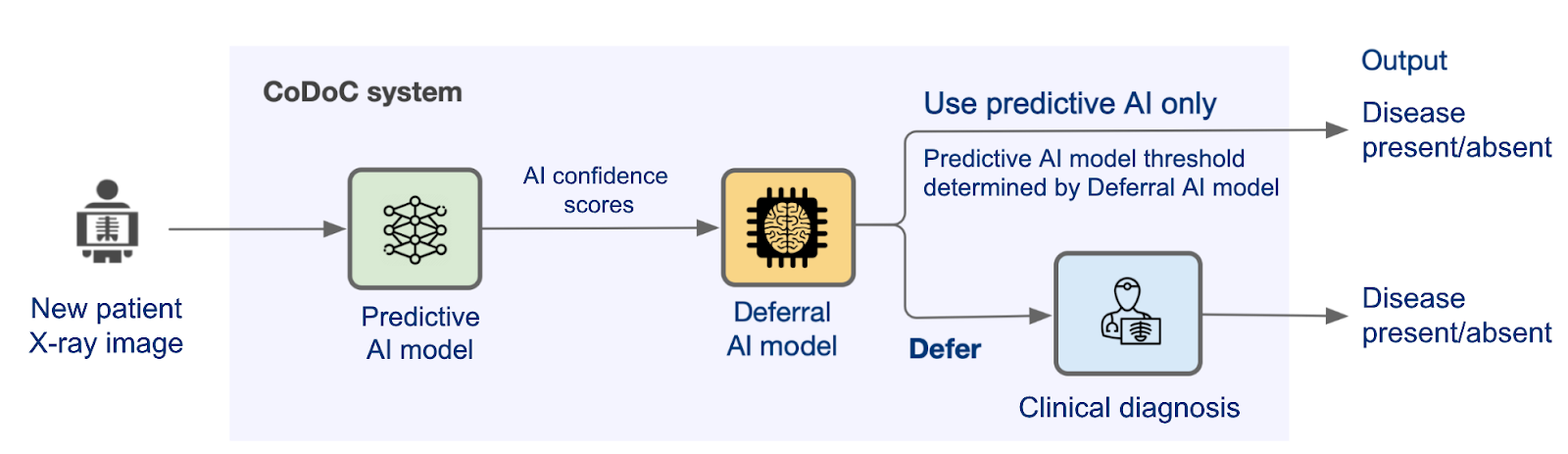
In practice, CoDoC would sit between the AI and the clinician in a clinical workflow. It would evaluate the confidence score generated by the AI model for a new patient image, then decide whether to accept the AI's interpretation or defer to the clinician to achieve the most accurate outcome.
In its initial testing phase, CoDoC performed impressively, demonstrating its ability to reduce the number of false positives by 25% for a large, de-identified UK mammography dataset, without missing any true positives. Additionally, it demonstrated how it could improve the triage of chest X-rays for tuberculosis testing, reducing the need for clinician reads by two-thirds.
It is important to remember that while CoDoC has shown promise in theoretical models, it still requires extensive real-world evaluation before deployment in healthcare settings. That said, the research is undoubtedly a significant step forward in the journey towards responsibly integrating AI into healthcare.
CoDoC offers a glimpse into a future where AI doesn't replace but rather complements human expertise to deliver safer and more efficient healthcare. It represents a future where AI systems know their limitations and when to defer to human judgment, allowing clinicians to lead when necessary to ensure optimal outcomes.
By open-sourcing CoDoC's code, DeepMind has extended an invitation for researchers worldwide to build on this work. Their goal is to collaborate with the broader scientific community to continue improving the transparency and safety of AI systems for real-world medical applications. This research marks an important milestone, but more open and collective effort is still needed to integrate predictive models responsibly and effectively in high-stakes domains like healthcare where human expertise remains invaluable.