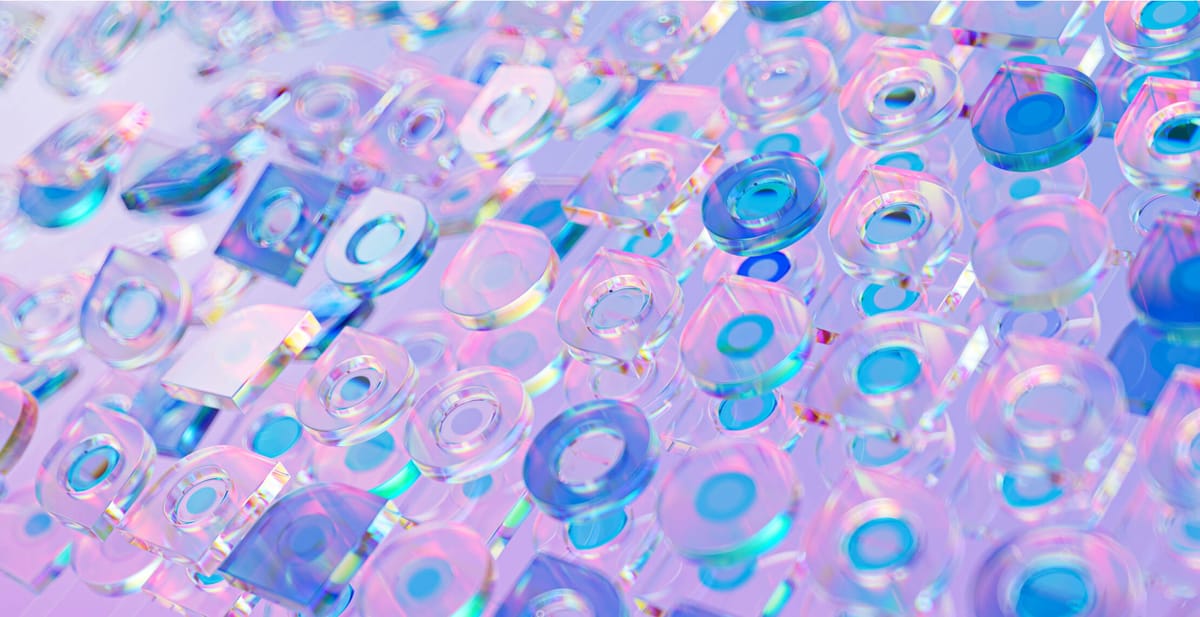
Google has announced the release of two domain-specific tools, Derm Foundation and Path Foundation, designed to generate embeddings from dermatological and pathology images, respectively. These tools aim to facilitate the development of custom machine learning models for specialized medical imaging domains, requiring less data and computational resources compared to traditional methods.
Derm Foundation and Path Foundation operate by converting input images into compressed numerical vectors, known as embeddings, which capture the essential features and patterns within the images. These embeddings serve as a pre-learned understanding of the domain, enabling researchers to efficiently train models for various downstream tasks, such as classifying skin conditions or identifying tumors in histopathology patches.
The introduction of these tools comes at a critical time, as there is a global shortage of medical imaging experts across specialties, including radiology, dermatology, and pathology. By leveraging AI technology, healthcare professionals can potentially interpret images more accurately and efficiently, alleviating the burden on specialists and improving patient outcomes.
Path Foundation, in particular, builds upon Google's previous research in self-supervised learning models for pathology images. The tool takes into account the unique characteristics of digital pathology images, such as their large size and information-dense nature, and incorporates pathology-specific optimizations to enhance the robustness and generalizability of the embeddings.
Similarly, Derm Foundation leverages dermatology-specific pre-training to capture the relevant features in skin condition images. The tool underwent a two-stage training process, combining contrastive learning on image-text pairs from the internet and fine-tuning for condition classification using clinical datasets.
Google's evaluation of these tools has yielded promising results. In the case of Path Foundation, linear classifiers trained using the embeddings significantly outperformed those trained on traditional ImageNet embeddings across multiple histopathology tasks. For Derm Foundation, models built on top of the dermatology-specific embeddings achieved higher quality compared to standard pre-trained image models, particularly when working with smaller training datasets.
The release of Derm Foundation and Path Foundation marks an exciting development in the field of AI-assisted healthcare. By providing researchers with access to these tools, Google aims to catalyze the development of innovative applications, such as diagnostic models, quality assurance systems, image indexing, and biomarker discovery.
However, it is important to note that while these tools offer a valuable starting point, downstream models built using the embeddings still require careful evaluation to understand their performance in real-world settings. As Google continues to explore the generalizability of these embeddings across different task types, patient populations, and image settings, the potential impact of Derm Foundation and Path Foundation on medical research and clinical practice remains promising.
Both tools have been made available to the research community to explore their utility for dermatology and pathology data. Researchers can sign up for access to each tool's terms of service and use the API to retrieve embeddings from images stored in Google Cloud. Approved users can also utilize example Colab notebooks to train models using public data for classifying common skin conditions or identifying tumors in histopathology patches.
By providing researchers with domain-specific embedding tools, Google aims to catalyze the development of machine learning applications in medical imaging, ultimately improving the accuracy and efficiency of medical image interpretation across various specialties.