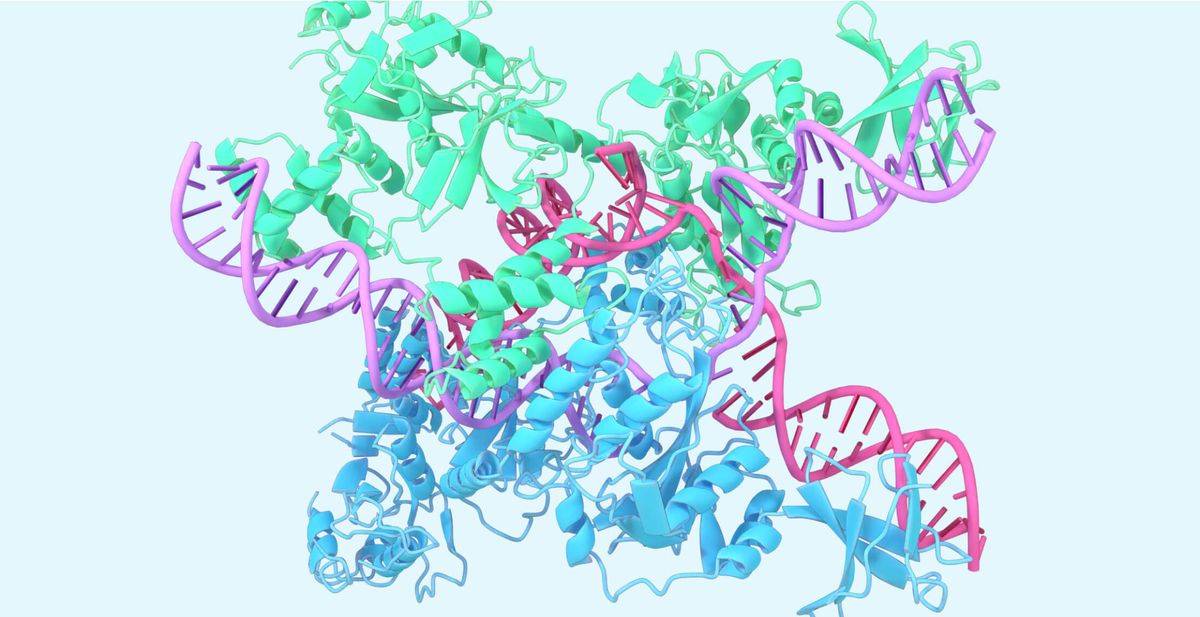
In 2020, DeepMind's AlphaFold AI system shocked researchers by predicting protein structures with unprecedented accuracy. Now, Google and biotech startup Isomorphic Labs have previewed AlphaFold's next generation, showing dramatically expanded capabilities beyond proteins to model complexes containing DNA, RNA, small molecule ligands, and post-translational modifications (PTMs).
This progress solidifies AlphaFold as a versatile tool that can accelerate discoveries across biology and drug development. While the original AlphaFold transformed protein folding predictions, the latest model provides a more complete picture of the diverse molecules driving biological mechanisms. The latest model can now generate predictions for nearly all molecules in the Protein Data Bank (PDB), frequently reaching atomic accuracy.
The new AlphaFold model achieves high precision across major molecule classes like ligands, nucleic acids, and PTMs that enable key cellular processes but have been difficult to model accurately before. For example, PTMs regulate protein activity through chemical modifications like phosphorylation, essential for cell signaling pathways implicated in cancer.
For ligands, AlphaFold's de novo structure predictions now significantly outperform molecular docking, the standard industry approach for modeling ligand-protein interactions for drug discovery. Molecular docking requires an existing protein structure and limits flexibility, whereas AlphaFold jointly models fully flexible complexes. This could enhance and accelerate rational drug design targeting novel proteins.
The model’s expanded capabilities and performance can help accelerate biomedical breakthroughs and realize the next era of 'digital biology’ — giving new insights into the functioning of disease pathways, genomics, biorenewable materials, potential therapeutic targets, mechanisms for drug design, and new platforms for enabling protein engineering, synthetic biology, and improving plant immunity.
Accurately predicting structures for this expanded range of biomolecules unlocks new ways AlphaFold could enable breakthroughs. Rational drug design could benefit from better modeling of ligands and antibody-antigen complexes. Understanding genomic mechanisms relies on nucleic acid structures. Even targeted gene editing via CRISPR/Cas systems, where AlphaFold recently modeled key protein-RNA-DNA complexes, could be enhanced.
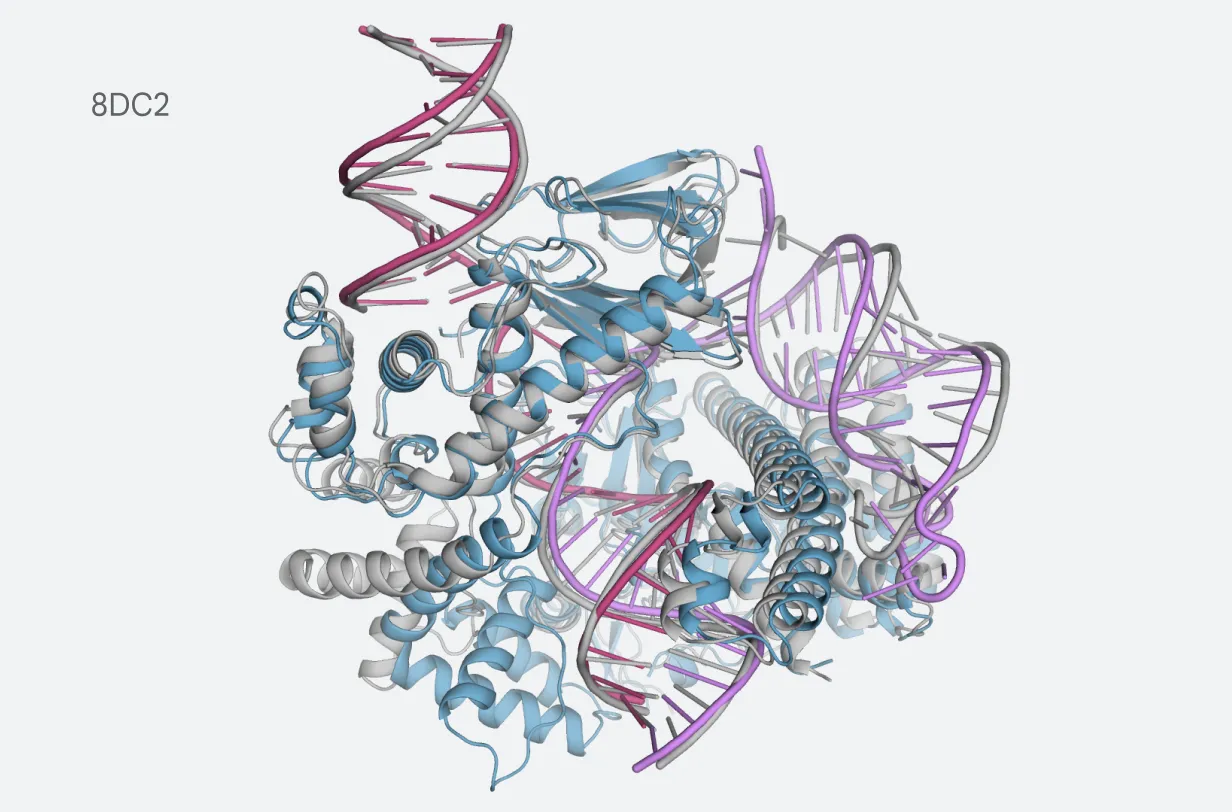
Isomorphic Labs is already applying the model for therapeutic design by rapidly characterizing disease-relevant macromolecular structures. Researchers worldwide can gain new insights into biological pathways, disease mechanisms, and potential therapeutic targets. Beyond human health, AlphaFold's capabilities could aid renewable bioproduct development, plant research, and synthetic biology engineering.
This demonstration of AI rapidly and accurately modeling diverse molecular structures represents a leap towards the futuristic vision of "digital biology." Simulating biological systems in silico using AI could someday revolutionize how researchers study life's complex molecular machinery.
While an immense challenge, such digital models calibrated against real-world data could yield biological insights at unprecedented speed, scope, and detail. For now, AlphaFold's expanded repertoire accelerates physical experiments by predicting structures researchers would otherwise have to determine through costly, laborious methods like X-ray crystallography or cryogenic electron microscopy.
The new model reflects sustained progress in AI research since AlphaFold first decoded the protein folding problem in 2020. AlphaFold Multimer then modeled multi-chain protein complexes in 2021. AlphaFold 2 in 2022 brought massive database expansion and accuracy gains, placing structure prediction within reach for almost any sequenced protein.
This latest model vaults beyond individual proteins to model complexes central to cells' intricate biochemical activity. It highlights the capability of modern AI systems to capture enormously multifaceted patterns in biological data that evaded human-crafted algorithms for decades.
Researchers emphasize AlphaFold is not solving these structures from scratch but rather inferring patterns from volumes of experimentally-determined structures. Still, its flexibility to adapt to modeling new molecule classes highlights the generalization power underpinning recent AI breakthroughs.
By predicting structures for this range of key molecules, AlphaFold's next generation could accelerate biomedical insights and therapeutic applications. But it also exemplifies AI's potential to enhance fundamental scientific understanding of life's complex molecular machinery.
Much work remains to integrate AlphaFold into pharmaceutical pipelines and basic research workflows. But its rapidly advancing capabilities promise to aid scientists in unlocking nature's deepest mysteries - a digital microscope illuminating biology's intricate nano-scale world.