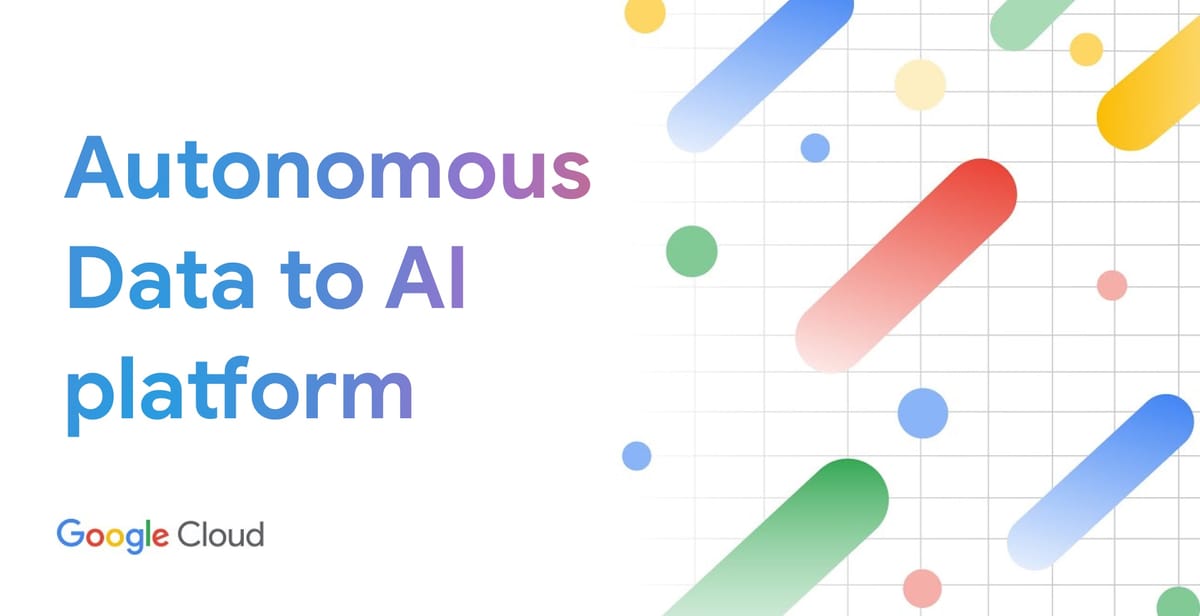
At Google Cloud Next 2025, the company unveiled significant updates to its data analytics platform that aim to make data work more conversational, contextual, and increasingly intelligent. During an exclusive interview, Peter Bailis, VP of Engineering at Google Cloud, explained the strategy behind these developments and discussed what the company calls an “autonomous data to AI platform.
Key Points:
- Gemini is now integrated into BigQuery and Looker, powering code assist, analytics, and data agents
- Google introduced specialized agents for roles like data engineers and business analysts
- A new BigQuery Knowledge Engine improves accuracy by understanding context and semantics
- Real-world customers are already reporting major cost savings and productivity boosts
"Google is the only frontier model provider with models at the top of the leader boards in all the benchmarks and evaluations that are tracked, that also has a data stack," Bailis told me. "Our vision is that we're going to infuse the data tools and the surface areas where data can be best utilized with intelligence from Gemini."
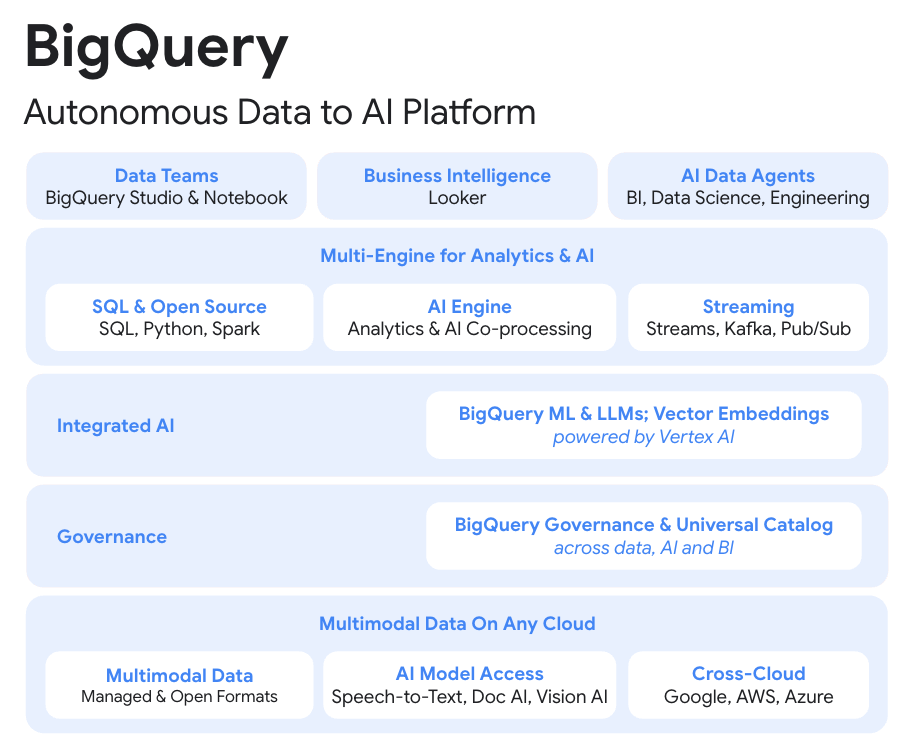
Let’s start with what’s new. Google launched specialized agents inside BigQuery and Looker, tailored to different user roles. Think data engineering agents that help build and maintain pipelines, data science agents that assist with model development in Colab notebooks, and conversational analytics agents in Looker that let you query data in plain English.
These aren’t just glorified chatbots. They’re deeply embedded into your workflows, trained on your organization’s data, and grounded in context through a new BigQuery Knowledge Engine that understands schema relationships, business terms, and query history.
“Autonomous” here doesn’t just mean set-it-and-forget-it infrastructure. It means the system can suggest joins, recommend transformations, and surface relevant datasets — without you manually wrangling CSVs or chasing down documentation.
Bailis put it this way: “The emphasis on autonomous is that the platform should be making more data available to more people without them doing more work.”
The results are impressive. Their SQL and Python code assistant in BigQuery has achieved a 60% acceptance rate among thousands of customers – double what Bailis describes as typical for coding models. Usage has grown 350% in the past nine months.
Why does this matter? Because today, data work largely remains a slog. Much of it comes down to handling repetitive tasks like remembering the correct formula for parsing timestamps into canonical formats. "A lot of data work is still drudgery," Bailis acknowledged, particularly in data engineering. "What data do I have and where do I go find it? How do I go join it? How do I go clean it up? It's repetitive."
The real magic isn't just in having a sophisticated AI model – it's in teaching that model to leverage organizational context. Gemini can understand the specific data patterns and query history within a company, making its suggestions far more relevant.
"Most logs never get read – must be something bad happens," Bailis explained. "Here we're saying the logs have important signal about what's important to each user and users like them."
Google is also targeting unstructured data – the images, audio, video, and text that often remain untapped in organizations. The company is making this "a first-class citizen" in BigQuery with multimodal tables, allowing unified storage and querying alongside structured data.
For businesses considering how to measure success with these tools, Bailis recommends looking at the time it takes to complete critical tasks for different roles. "Data work is ultimately a reflection of an organization's priorities," he said. The most telling metric? "The number of questions getting asked and answered should go up dramatically."
Beyond Dashboards
The most exciting part of the conversation was around what’s coming next. Google isn’t stopping at conversational BI. The company is envisioning data agents that behave more like analysts — watching your business day-to-day and proactively surfacing changes.
“Imagine an analyst that just looks at things all day and tells you when something meaningful happens — and not just you, but every knowledge worker in your organization,” Bailis said.
For the Skeptics: Start Small, But Start Now
I pressed Bailis on the elephant in the room for enterprise: trust. Many companies are still wary of putting AI in the loop for critical business decisions. But his advice was pragmatic — start small. Build internal-facing agents. Set clear instructions. Ground them in a semantic model. And layer in governance the way you would with any other software product.
“You don’t have to hand decision-making over to AI,” he said. “But you do need to build a path to using it effectively — and that means creating space to experiment.”
For enterprises still struggling with their data foundation, Google's invitation to experiment with autonomous data to AI platform couldn't come at a better time. The future of data work isn't just about smarter tools—it's about fundamentally changing who can participate in data conversations and how quickly those conversations transform into action.