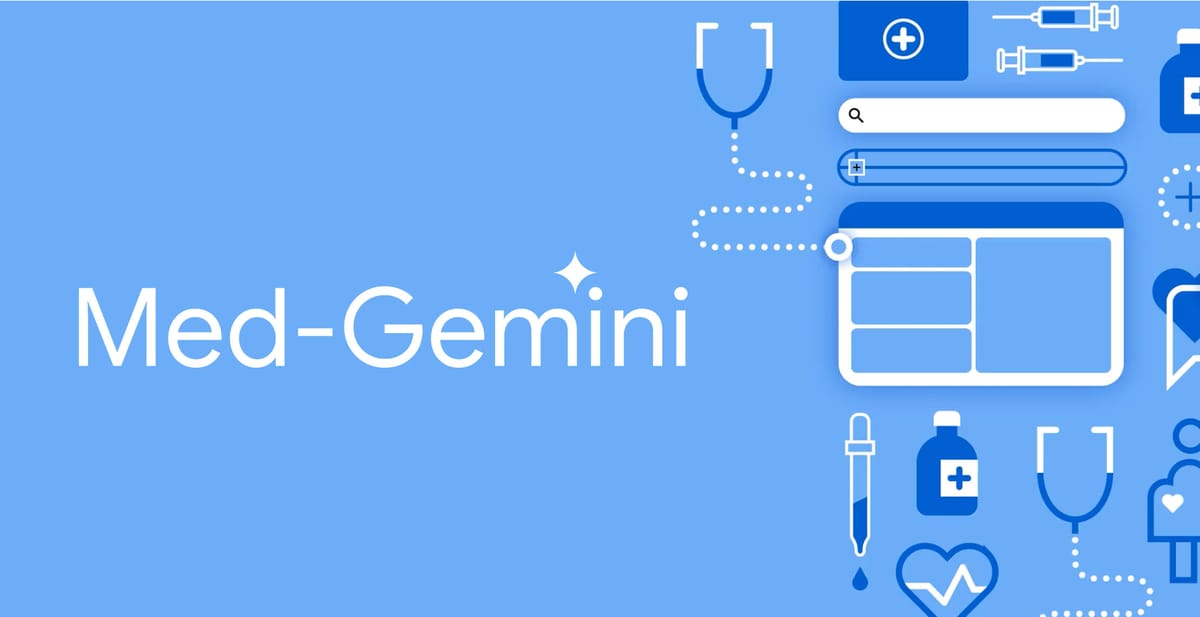
Researchers at Google have released a paper showcasing Med-Gemini, a new family of highly capable multimodal models built on top of their powerful Gemini models. By leveraging advances in clinical reasoning, multimodal understanding, and long-context processing, Med-Gemini achieves state-of-the-art performance across an extensive suite of medical benchmarks, suggesting exciting potential for real-world applications.
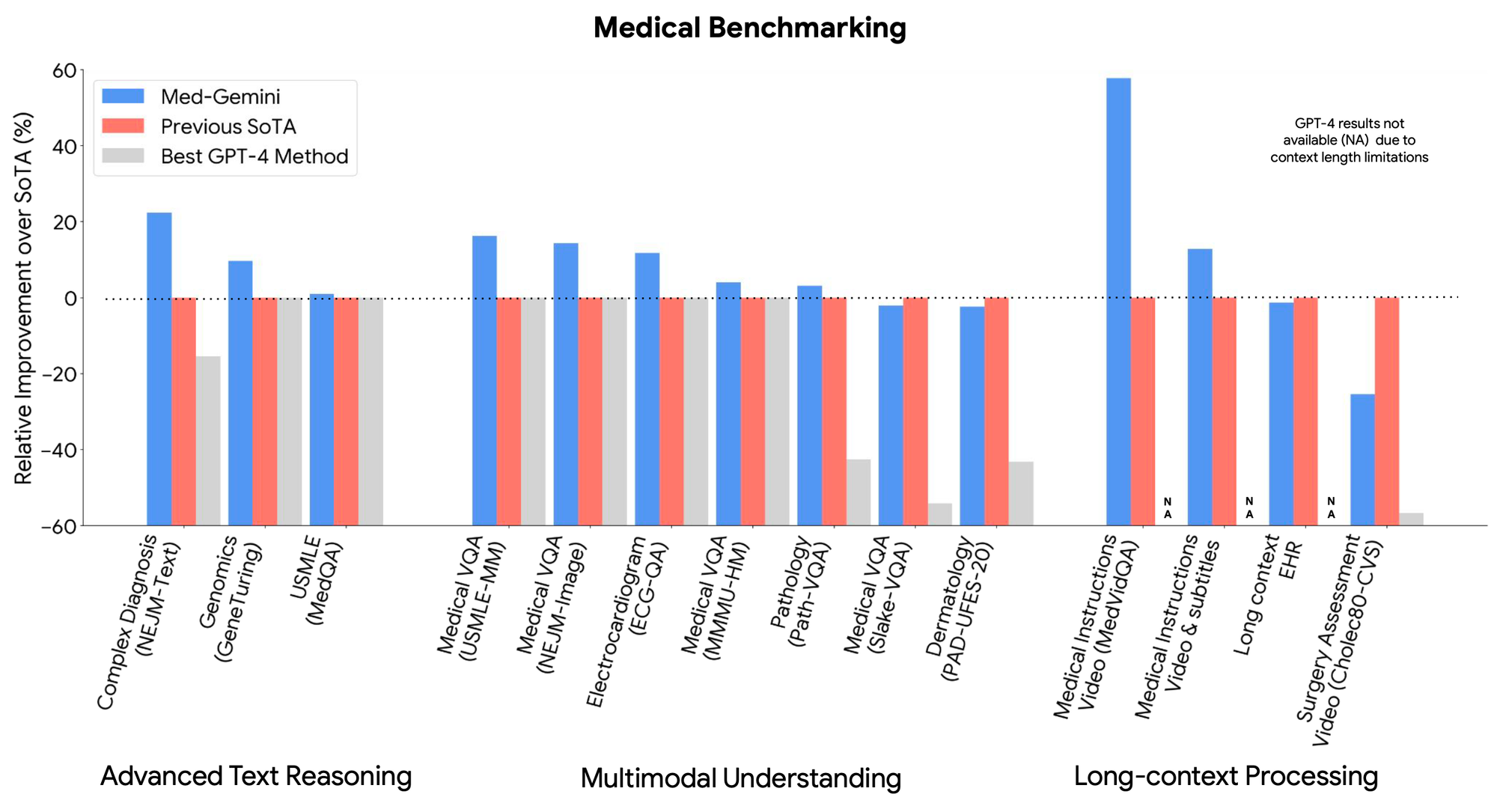
Med-Gemini builds upon the Gemini 1.0 and Gemini 1.5 models and are fine-tuned and specialised for medicine. They were trained using a combination of fine-tuning and self-training techniques, with a focus on enhancing their ability to perform advanced reasoning and make use of web search.
For tasks requiring advanced reasoning, the Med-Gemini-L 1.0 model was developed by fine-tuning the Gemini 1.0 Ultra model. This involved generating synthetic datasets with reasoning explanations, known as "Chain-of-Thoughts" (CoTs), and integrating web search results to improve the model's ability to utilise external information.
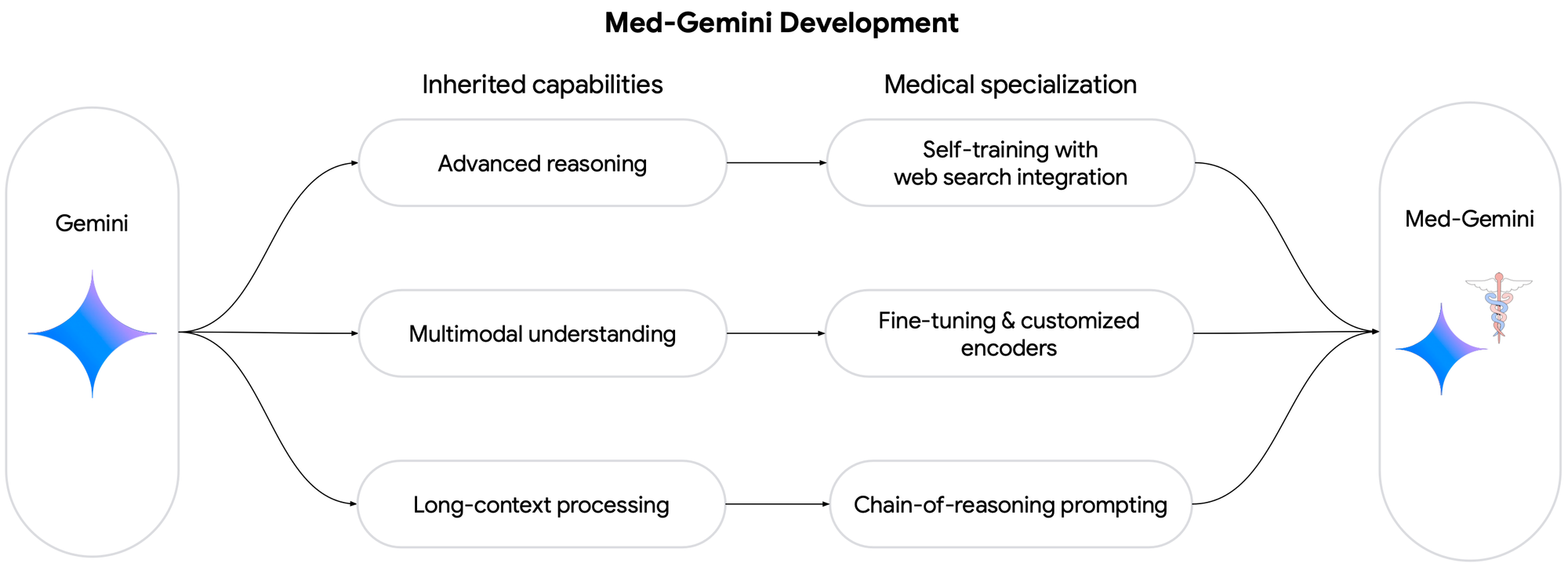
For multimodal understanding, the Med-Gemini-M 1.5 model was created by fine-tuning the Gemini 1.5 Pro model on a range of multimodal medical datasets. Additionally, the Med-Gemini-S 1.0 model was developed by augmenting the Gemini 1.0 Nano model with a specialised encoder to process raw biomedical signals, such as electrocardiograms (ECGs).
The Med-Gemini models introduce several key innovations:
- Advanced reasoning capabilities are bolstered through self-training and integration with web search, enabling the models to provide more factually accurate and nuanced responses to complex clinical queries. This is exemplified by Med-Gemini-L 1.0 achieving a new state-of-the-art accuracy of 91.1% on MedQA (USMLE), an important benchmark for medical question answering.
- Multimodal understanding is enhanced via fine-tuning and customized encoders, allowing Med-Gemini to adapt to novel medical data types like electrocardiograms. Across seven multimodal benchmarks including NEJM Image Challenges, Med-Gemini improves over GPT-4V by an average margin of 44.5%. Qualitative examples also showcase Med-Gemini-M 1.5's promise for multimodal medical dialogue.
- Efficient long-context processing empowers Med-Gemini to reason over lengthy medical records and videos. On a challenging "needle-in-a-haystack" task requiring identification of subtle findings in extensive EHR data, Med-Gemini-M 1.5 matches carefully engineered baselines. It also sets a new bar on medical instructional video question answering.
These capabilities translate into potential for meaningful real-world utility. Quantitative evaluations show Med-Gemini generating medical visit summaries and referral letters that clinicians judge to be on par with expert-written versions. Early demonstrations highlight the models' aptitude for applications like multimodal diagnostic assistance, biomedical research summarization, and medical education.
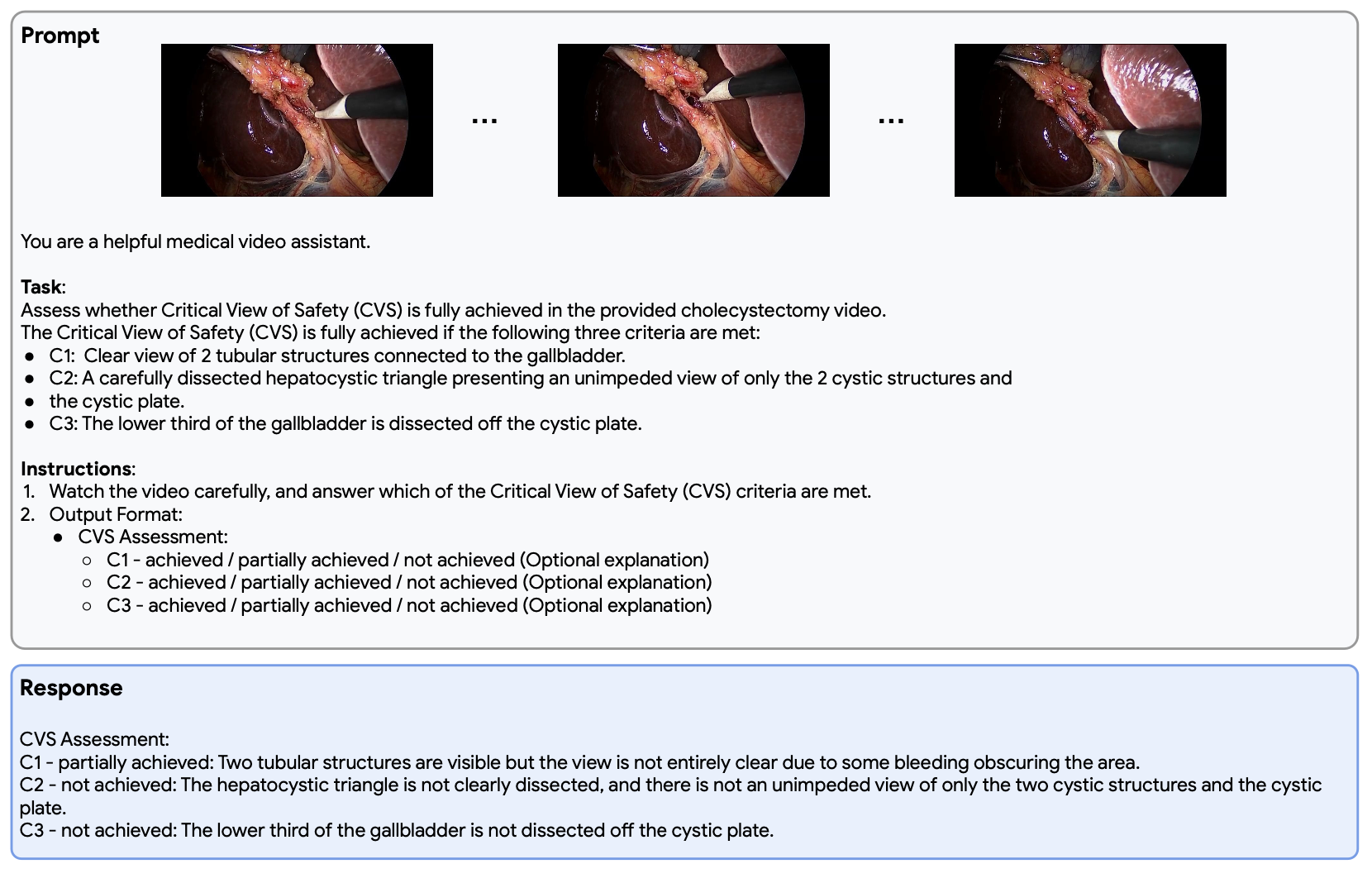
However, the researchers emphasize the need for further rigorous evaluation and refinement before deploying these AI systems in safety-critical medical settings. Key focus areas include fairness, privacy, transparency and mitigating unintended biases.
Overall, Med-Gemini is an exciting leap forward for AI in medicine, offering a powerful substrate for accelerating biomedical progress and improving healthcare experiences.