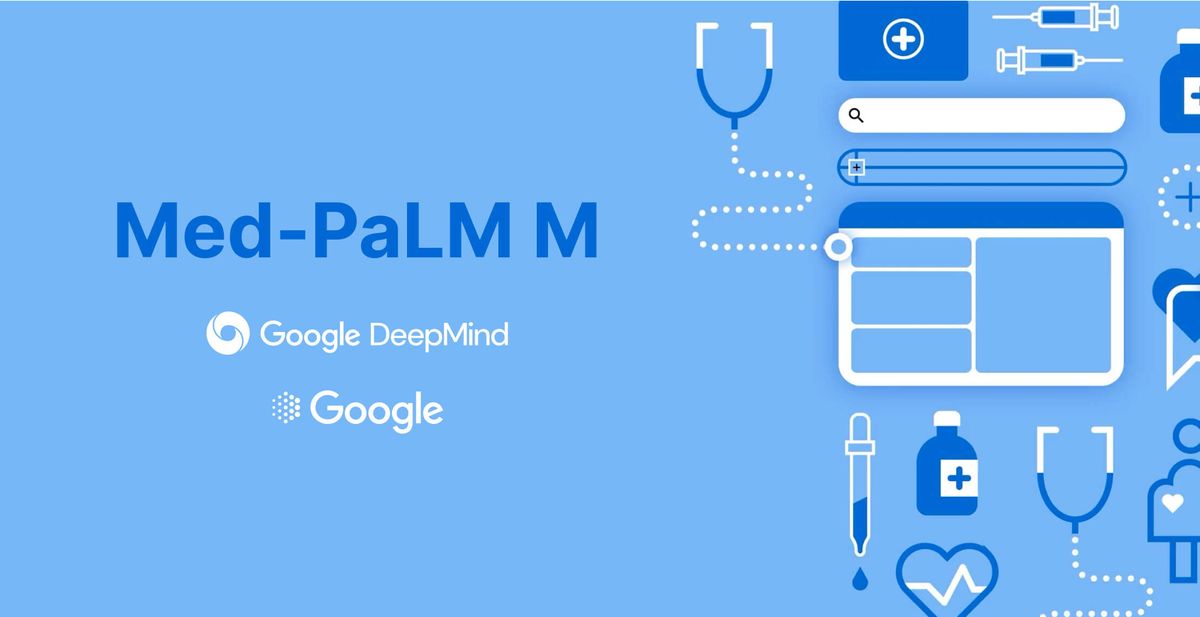
Researchers from Google and DeepMind have unveiled Med-PaLM M, the first demonstration of a generalist multimodal biomedical AI system. Med-PaLM M encodes and interprets diverse types of medical data spanning text, images, genomics and more - all within the same model architecture. This development highlights the potential of flexible, general-purpose AI systems to unlock new capabilities in biomedicine.
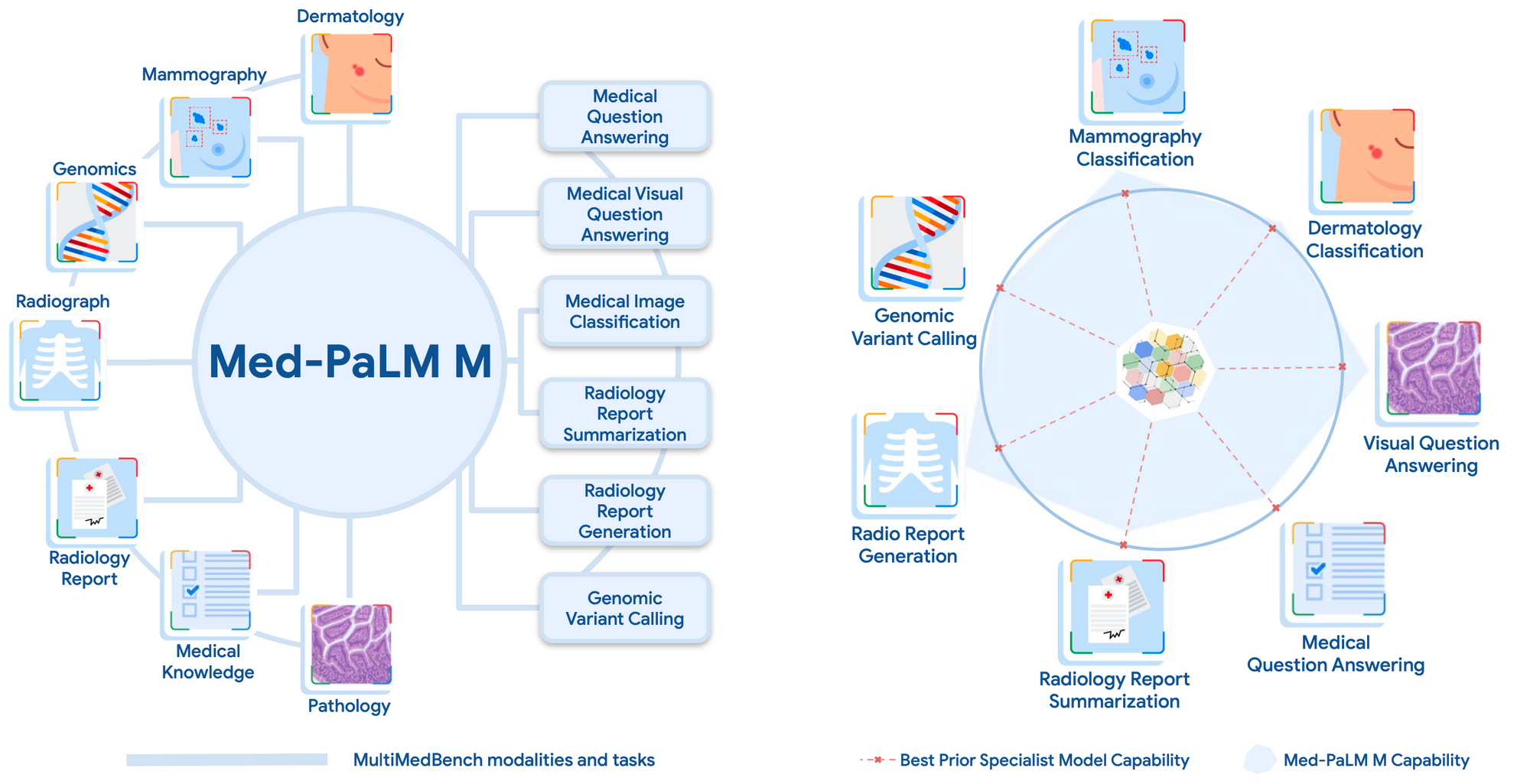
To enable the development and benchmarking of Med-PaLM M, the researchers curated MultiMedBench - a new multimodal medical dataset spanning 14 tasks across modalities including text, medical imaging, and genomics. MultiMedBench contains over 1 million examples for question answering, report generation, classification, and other clinically relevant tasks. This comprehensive benchmark was key to training and evaluating Med-PaLM M's capabilities across diverse biomedical applications.
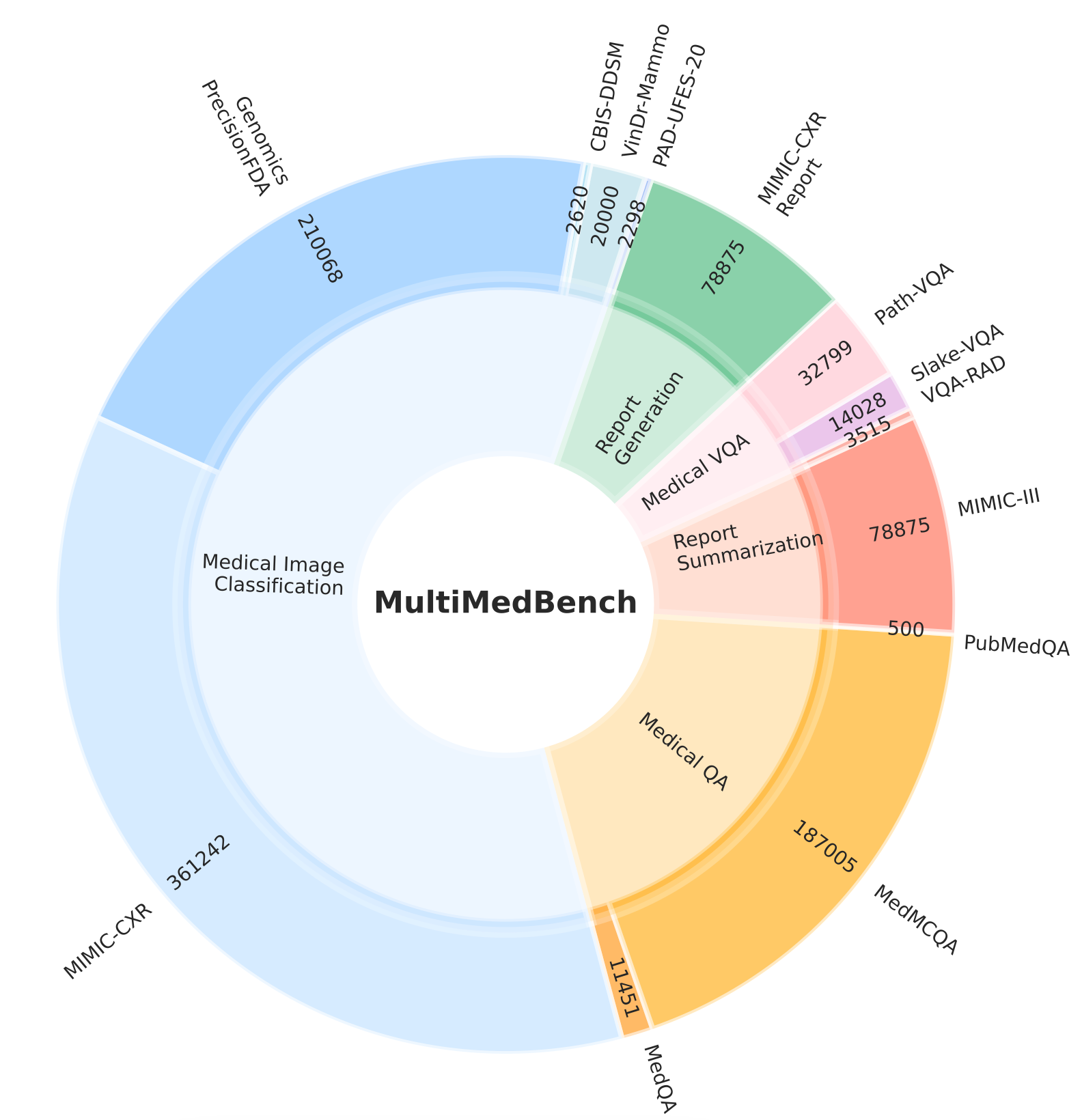
Med-PaLM M is built on PaLM-E, a recently introduced generalist AI model capable of strong performance on language, vision and multimodal tasks. By further training PaLM-E using MultiMedBench, the researchers adapted it into a versatile system for biomedical applications.
Across all tasks in the benchmark, Med-PaLM M reached or exceeded state-of-the-art performance, often surpassing specialized models optimized for individual tasks by a wide margin.
Med-PaLM M is not just about setting new performance benchmarks. It signifies a paradigm shift in how we approach biomedical AI. A single flexible model that can understand connections across modalities has major advantages. It can incorporate multimodal patient information to improve diagnostic and predictive accuracy. The common framework also enables positive transfer of knowledge across medical tasks. In an ablation study, excluding some training tasks hurt performance, demonstrating the benefits of joint training.
Preliminary evidence suggests that Med-PaLM M can generalize to novel medical tasks and concepts and perform zero-shot multimodal reasoning, all through language-based instructions and prompts. For instance, the model has been found to accurately identify and describe tuberculosis in chest x-rays despite having never encountered presentations of the disease before in images.
To assess the clinical applicability of Med-PaLM M, a radiologist evaluation of AI-generated reports across model scales was conducted. The clinically significant error rate for Med-PaLM M was found to be on par with radiologists from prior studies, suggesting potential clinical utility. In a side-by-side ranking on 246 retrospective chest X-rays, clinicians expressed a pairwise preference for Med-PaLM M reports over those produced by radiologists in up to 40.5% of cases.
While significant work remains to validate these models in real-world use cases, the results achieved by Med-PaLM M represent a milestone towards the development of generalist biomedical AI systems. The development of Med-PaLM M has not only introduced a new multimodal biomedical benchmark, but also demonstrated the first generalist biomedical AI system that reaches performance competitive with or exceeding state-of-the-art specialist models on multiple tasks.
Evidence of novel emergent capabilities in Med-PaLM M, such as zero-shot medical reasoning, generalization to novel medical concepts and tasks, and positive transfer across tasks, hint at the promising potential of such systems in downstream data-scarce biomedical applications.
The work done by the teams at Google Research and Google DeepMind is a significant leap forward in the field of biomedical AI, paving the way for AI systems that can interpret multimodal data with complex structures to tackle many challenging tasks. As biomedical data generation and innovation continue to increase, the potential impact and applications of such models are expected to broaden, spanning from fundamental biomedical discovery to care delivery.