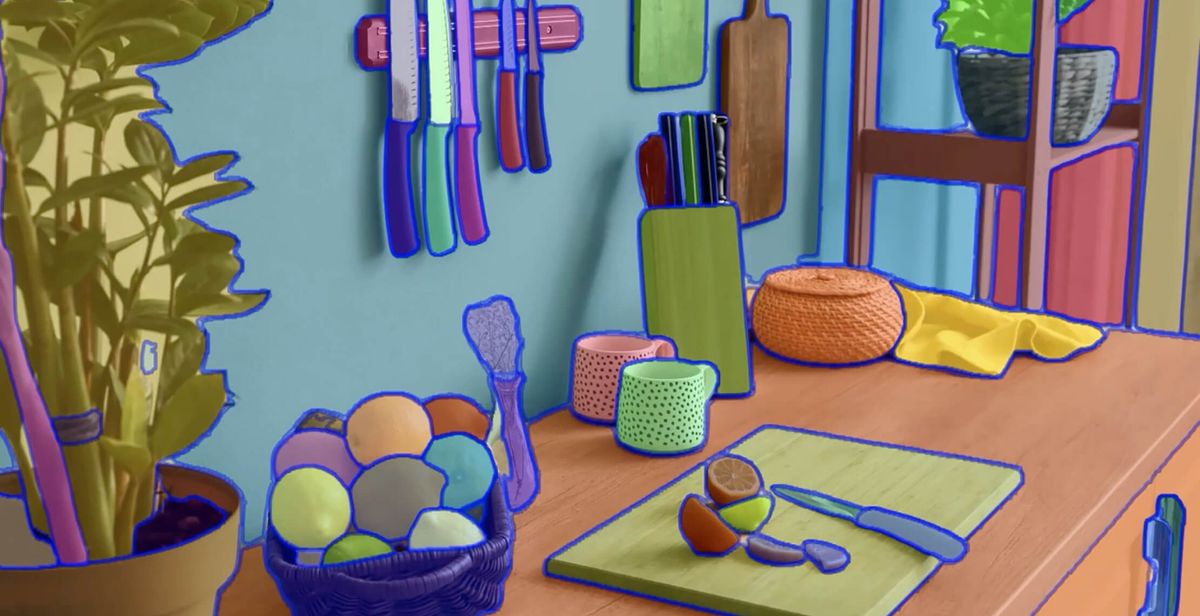
Meta AI, has announced a new project called Segment Anything, which aims to democratize segmentation using its new AI model called SAM. Segmentation, or identifying which image pixels belong to an object, is a core task in computer vision that has many applications, from analyzing scientific imagery to editing photos.
Previously, creating an accurate segmentation model for specific tasks typically required highly specialized work by technical experts with access to AI training infrastructure and large volumes of carefully annotated in-domain data. With the Segment Anything project, this could be a thing of the past.
The project is centered around SAM, a new AI model that can "cut out" any object, in any image, with a simple "click". SAM is trained on a diverse, high-quality dataset of over 1 billion masks, making it possible to generalize to new types of objects and images beyond what it observed during training. The model's promptable interface allows it to be used in flexible ways that make a wide range of segmentation tasks possible simply by engineering the right prompt for the model, such as clicks, boxes, text, and more.



SAM can be used for both interactive segmentation, where a person guides the model by iteratively refining a mask, and automatic segmentation, where the model segments specific object categories defined ahead of time. The model can output multiple valid masks when faced with ambiguity about the object being segmented, which is an important capability for solving segmentation in the real world.
SAM's promptable design enables it to take input prompts from other systems, such as using a user's gaze from an AR/VR headset to select an object. The model can also take input prompts from object detectors, such as bounding box prompts, to enable text-to-object segmentation. Output masks generated by SAM can be used as inputs to other AI systems for object tracking in videos, image editing applications, lifting objects to 3D, and creative tasks like collaging.
SAM has learned a general notion of what objects are, which enables it to perform zero-shot generalization to unfamiliar objects and images without requiring any additional training. This is made possible by SAM's sophisticated ambiguity-aware design. SAM was trained on millions of images and masks using a model-in-the-loop "data engine." Researchers used SAM to interactively annotate images and update the model, which was repeated many times to improve both the model and the dataset.
To build the SA-1B dataset, which includes over 1.1 billion segmentation masks collected on about 11 million licensed and privacy-preserving images, Meta AI used SAM to interactively annotate images, and then used the newly annotated data to update SAM iteratively. The dataset is the largest to date and has the potential to benefit a broad range of applications and researchers.
One of the most impressive aspects of SAM is its ability to generate multiple valid masks for ambiguous prompts, which is a crucial capability for solving segmentation in the real world. SAM's promptable design also enables flexible integration with other systems, making it an ideal component in AR/VR, content creation, and scientific study applications, among others.
Furthermore, the fact that Meta is open sourcing the research and releasing both the SAM model and the SA-1B dataset for public use is highly commendable. This move not only encourages further research into foundation models for computer vision but also provides a more equitable opportunity for individuals and organizations who may not have had access to the resources needed to develop and train their own segmentation models.
You can try a demo of SAM directly in your browser.