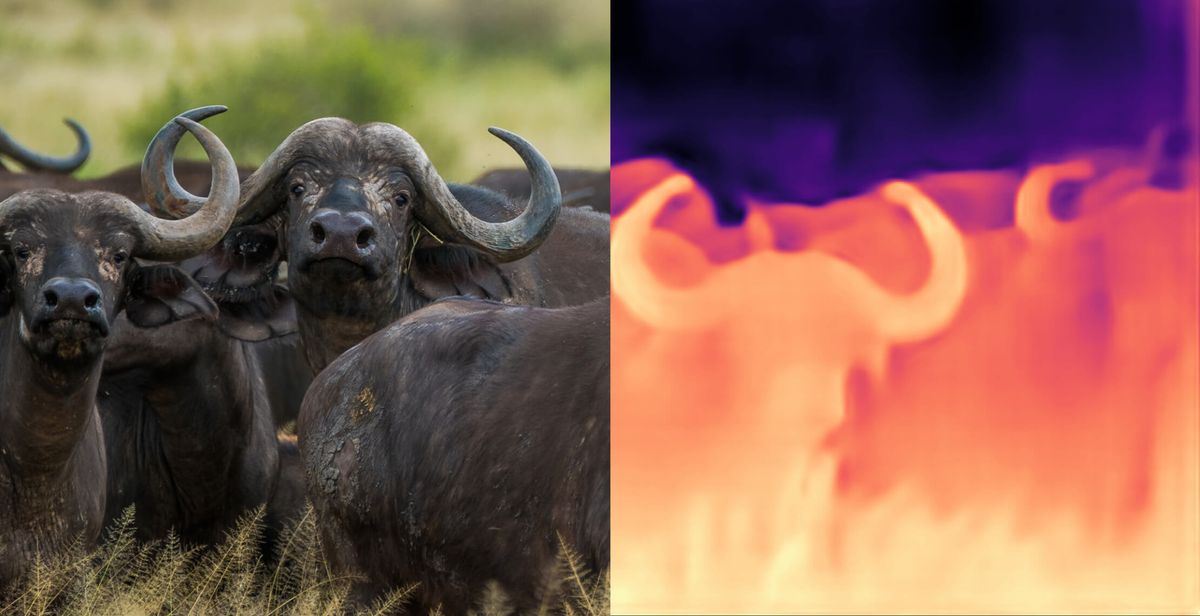
Meta AI, today released DINOv2, a self-supervised Vision Transformer model that is making waves in the AI community. We had the opportunity to test this model, and the initial results have been nothing short of impressive. From depth estimation to semantic segmentation and instance retrieval, DINOv2 showcases remarkable capabilities.
But what makes DINOv2 stand out from the crowd? For starters, it significantly improves upon the previous state of the art in self-supervised learning and rivals the performance of weakly-supervised features. It achieves this by pretraining on a massive, curated dataset of 142 million images without any supervision. The features produced by DINOv2 models are versatile and require no fine-tuning, demonstrating exceptional out-of-distribution performance.


In their research paper introducing DINOv2, the team emphasizes the emergence of properties such as understanding object parts and scene geometry, regardless of the image domains. They foresee that more of these properties will develop as the scale of models and data increases, drawing a parallel with the instruction emergence seen in large language models like GPT-4. They specifically mention:
We expect that more of these properties will emerge at larger scales of models and data, akin to instruction emergence in large language models.
This insight reveals the impressive potential of DINOv2 and its ability to adapt and evolve with even larger datasets and models.
Speaking of applications, the potential for DINOv2 is immense. Its features can be used for image-level visual tasks such as image classification, instance retrieval, and video understanding, as well as pixel-level visual tasks like depth estimation and semantic segmentation. These applications are particularly relevant in industries like autonomous vehicles, robotics, and even augmented reality, where understanding and interpreting visual data is crucial.
DINOv2's release comes on the heels of Meta AI's open-source Segment Anything project, which featured the groundbreaking SAM model for image segmentation. This demonstrates Meta AI's commitment to leading the charge in computer vision technology and pushing the boundaries of what's possible.
As for the future of DINOv2, researchers plan to "leverage this ability to train a language-enabled AI system that can process visual features as if they were word tokens, and extract the required information to ground the system." This suggests an exciting convergence of natural language processing and computer vision, which could have far-reaching implications across numerous domains.
As Meta AI continues to spearhead advancements in the computer vision arena, the prospects for self-supervised Vision Transformer models are becoming increasingly promising, and we eagerly await the company's forthcoming breakthroughs in this rapidly evolving field.