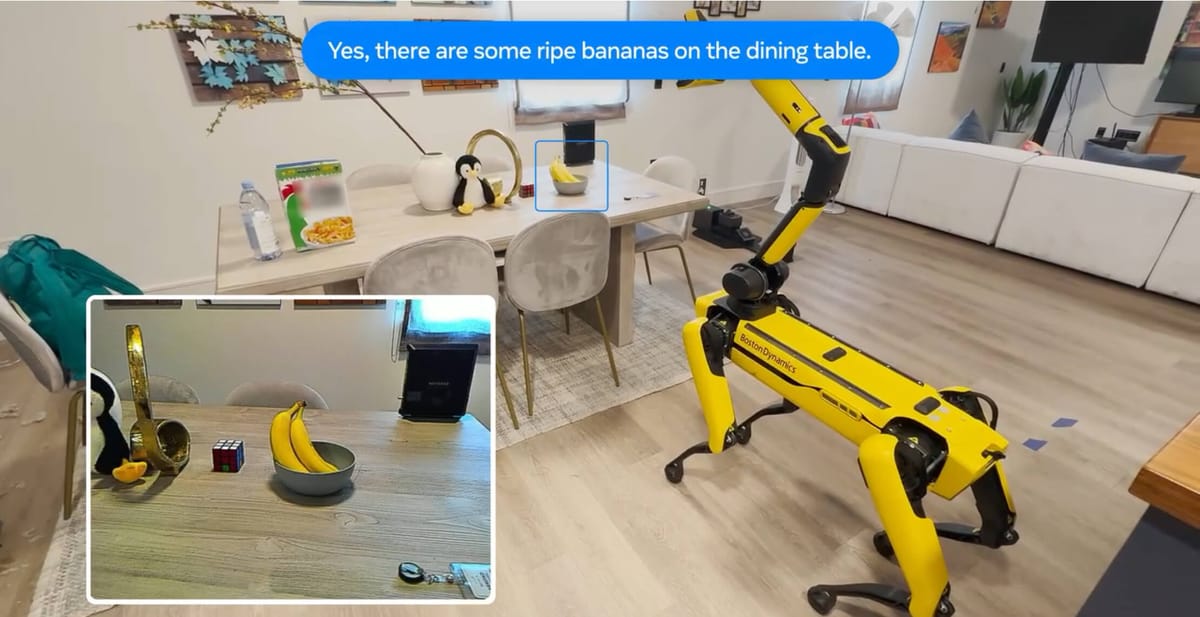
Meta has unveiled a new benchmark called Open-Vocabulary Embodied Question Answering (OpenEQA), designed to evaluate an AI model's understanding of physical spaces through open-ended questions. The benchmark aims to measure an AI agent's ability to answer queries like "Where did I leave my badge?" based on its comprehension of the environment.
OpenEQA consists of over 1,600 non-templated question-answer pairs sourced from human annotators, covering real-world use cases across more than 180 videos and scans of physical environments. The question-answer pairs were validated by different annotators to ensure their answerability and correctness. The benchmark includes two tasks: episodic memory EQA, where the AI agent answers questions based on its recollection of past experiences, and active EQA, which requires the agent to take action within the environment to gather information and respond to queries.
Meta researchers benchmarked state-of-the-art vision and language models (VLMs) using OpenEQA and discovered a significant performance gap between even the best model (GPT-4V at 48.5%) and human-level performance (85.9%). Notably, for questions demanding spatial understanding, today's VLMs are nearly "blind," with access to visual content providing no substantial improvement over language-only models. This finding underscores the need for advancements in both perception and reasoning before embodied AI agents powered by such models can be deployed in real-world scenarios.
The OpenEQA benchmark also introduces LLM-Match, an automatic evaluation metric for scoring open-vocabulary answers. Through blind user studies, Meta found that LLM-Match correlates with human judgment as strongly as two humans do with each other, validating its effectiveness as an evaluation tool.
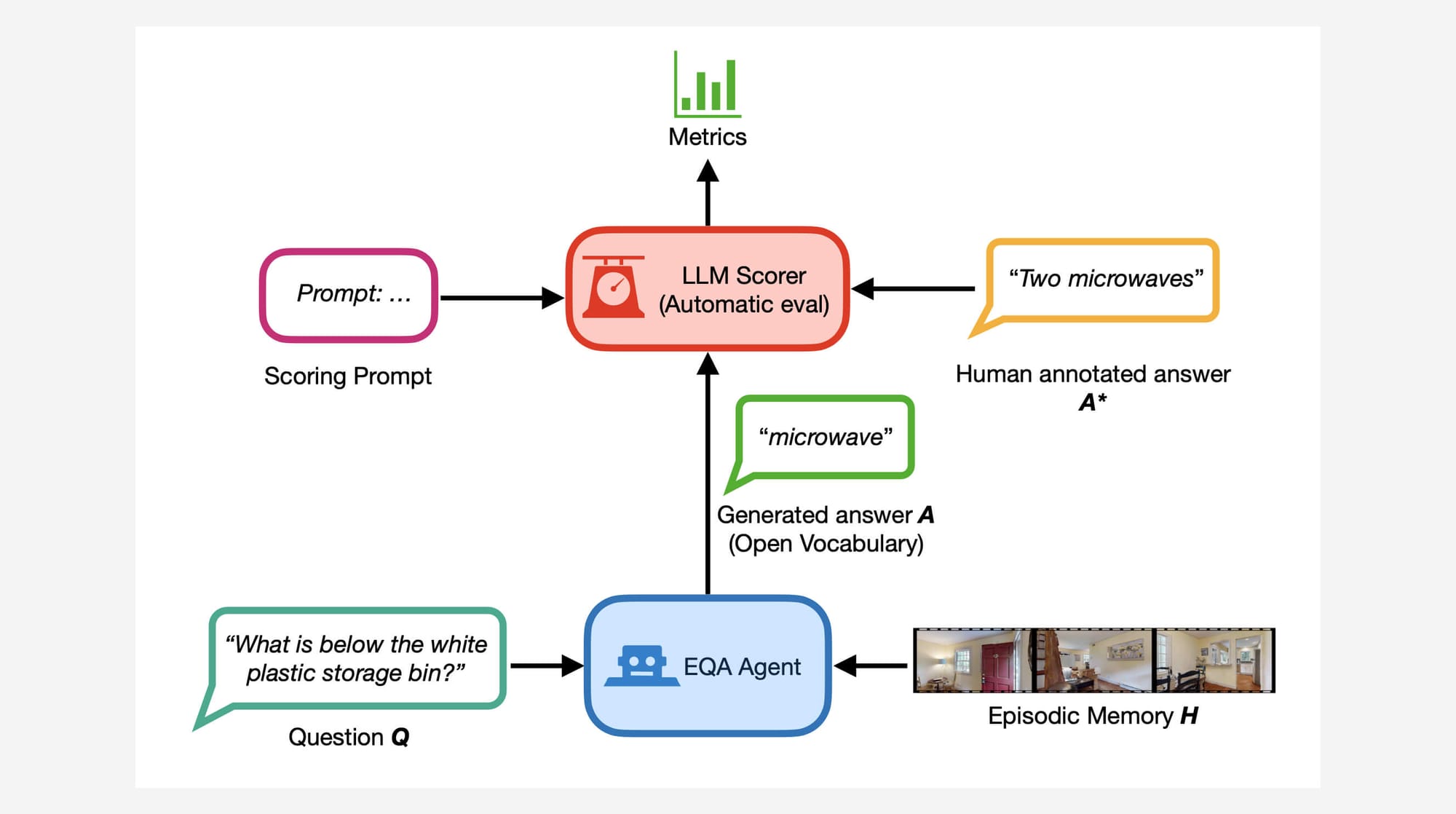
Meta's research paper on OpenEQA highlights the importance of developing "world models" – AI agents capable of understanding and communicating about the world they perceive – as a crucial step towards artificial general intelligence (AGI). The paper states, "OpenEQA combines challenging open-vocabulary questions with the ability to answer in natural language. This results in a straightforward benchmark that demonstrates a strong understanding of the environment—and poses a considerable challenge to current foundational models."
Benchmarks like OpenEQA are important for embodied AI research. By providing a realistic evaluation framework, it highlights the strengths and weaknesses of current AI models in understanding physical spaces. With it, researchers can now focus on improving AI agents' perception, reasoning, and commonsense knowledge, bringing us closer to the vision of artificial general intelligence.