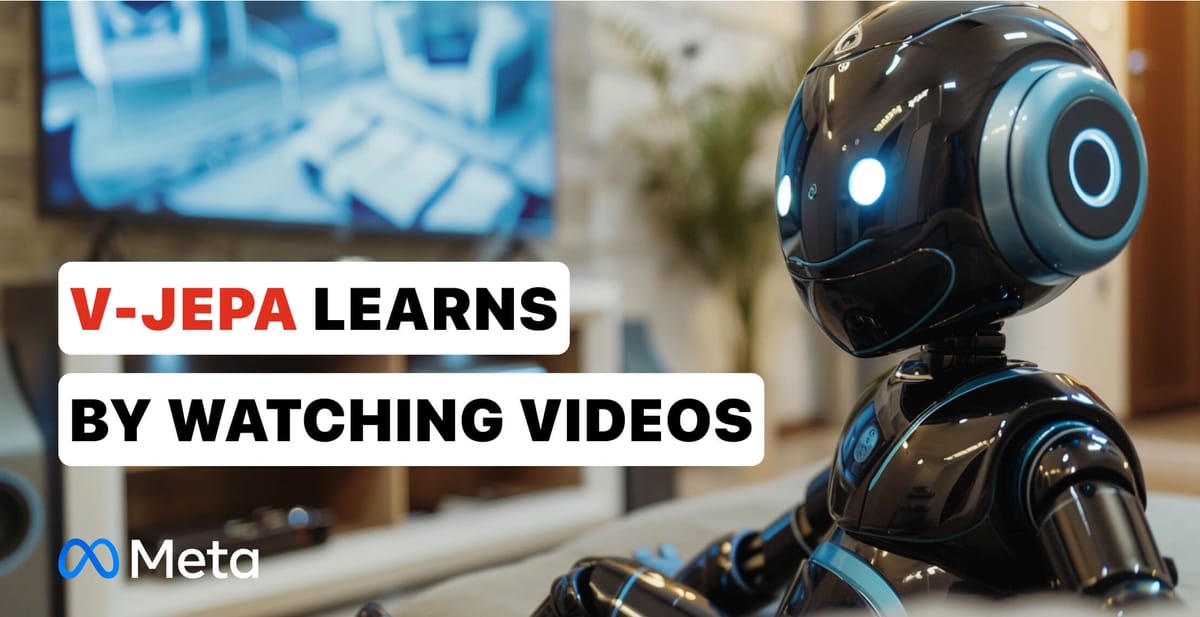
Today, Meta unveiled V-JEPA ( short for Video Joint Embedding Predictive Architecture), a new vision model that learns to understand the physical world by watching videos. The JEPA initiative aims to equip AI with the ability to plan, reason, and execute complex tasks by forming an internal model of their surroundings
The release of V-JEPA follows in the footsteps of last year's introduction of I-JEPA (Image Joint Embedding Predictive Architecture), the first AI model embodying Yann LeCun’s vision for a more human-like approach to AI. I-JEPA set a precedent by learning through the creation of an internal model of the outside world, focusing on abstract representations rather than direct pixel comparison. Its ability to deliver high performance across various computer vision tasks while remaining computationally efficient highlighted the potential of predictive architectures. V-JEPA extends this vision to video, leveraging the foundational principles of I-JEPA to understand dynamic interactions and the temporal evolution of scenes.
What sets V-JEPA apart is its self-supervised learning approach that predicts missing sections of video within an abstract feature space instead of generative approach of filling in missing pixels. This technique builds conceptual understanding of the video footage not by manual labeling, but through passive observation like a human.
V-JEPA leverages unlabeled video to learn, and needs minimal labeled data for fine-tuning specific tasks. By comparing compact latent representations, the approach also concentrates compute on high-level semantic information rather than unpredictable visual details.
Researchers report marked gains in pre-training efficiency over existing video models, with improvements ranging from 1.5 to 6 times in sample and compute efficiency. The streamlined methodology paves the way for quicker and more economical development of future video comprehension models.
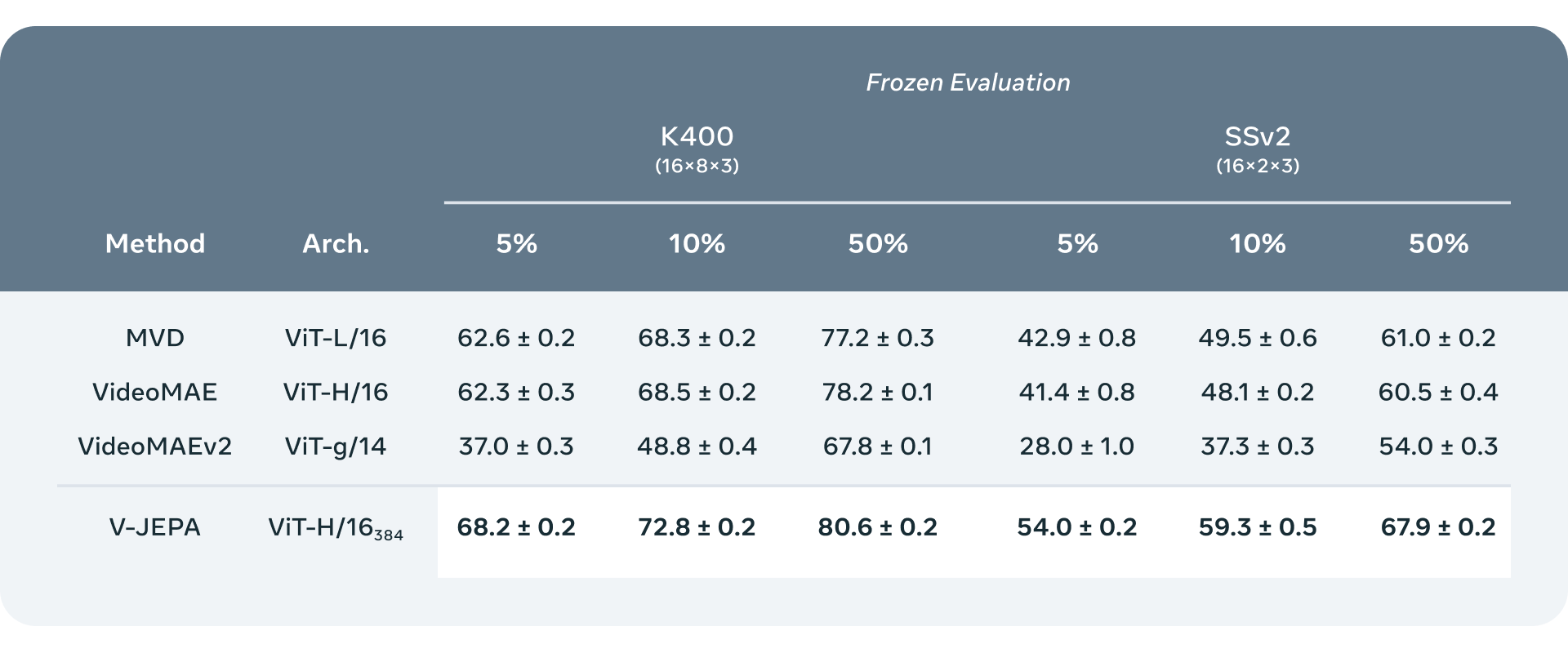
Initial benchmark results already match or top existing video recognition models on Kinetics-400, Something-Something-v2, and ImageNet. More impressively, when researchers freeze V-JEPA and add a specialized classification layer, the model reaches new performance heights—all trained on a fraction of previous data requirements.
The introduction of V-JEPA is not just about advancing video understanding; it's about redefining the possibilities for AI in interpreting the world. By learning to predict and understand unseen segments of videos, V-JEPA moves closer to a form of machine intelligence that can reason about and anticipate physical phenomena, akin to human learning from observation. Moreover, the model's flexibility in applying learned representations to various tasks without extensive retraining opens new avenues for research and application, from action recognition to assisting in augmented reality environments.
Looking forward, the V-JEPA team says it is exploring the integration of multimodal data, such as audio, to enrich the model's understanding of the world. This evolution represents an exciting frontier for AI research and promises to unlock new capabilities in machine intelligence. LeCun posists that this could lead to more flexible reasoning, planning, and general intelligence.
Meta has released V-JEPA under a Creative Commons NonCommercial license. You can read the full paper here, and get the code on GitHub.