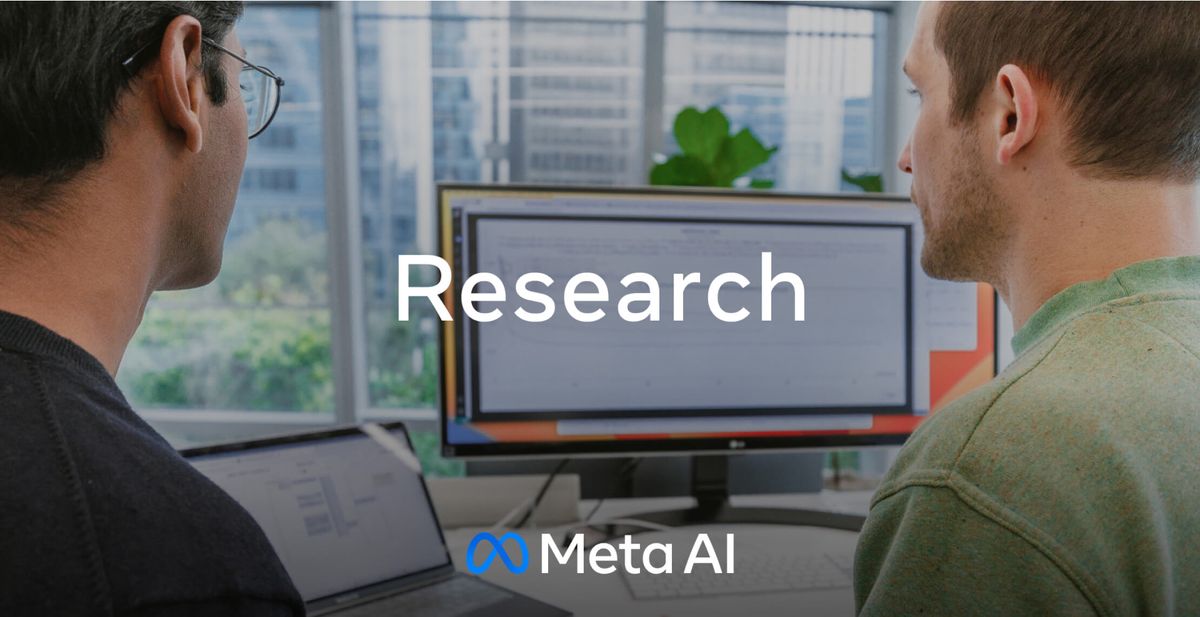
Researchers at Meta AI, in collaboration with Reka AI and Abridge AI, have published new research and open-sourced BELEBELE, a dataset for evaluating natural language understanding across 122 diverse languages. Additionally, they used this dataset to evaluate the capabilities of multilingual masked language models (MLMs) and large language models (LLMs).
BELEBELE represents the largest parallel multilingual benchmark ever created specifically for reading comprehension. The dataset contains 900 multiple-choice reading comprehension questions based on short passages. The questions test for general language understanding without requiring external knowledge. Each question has four possible answers, only one of which is correct.
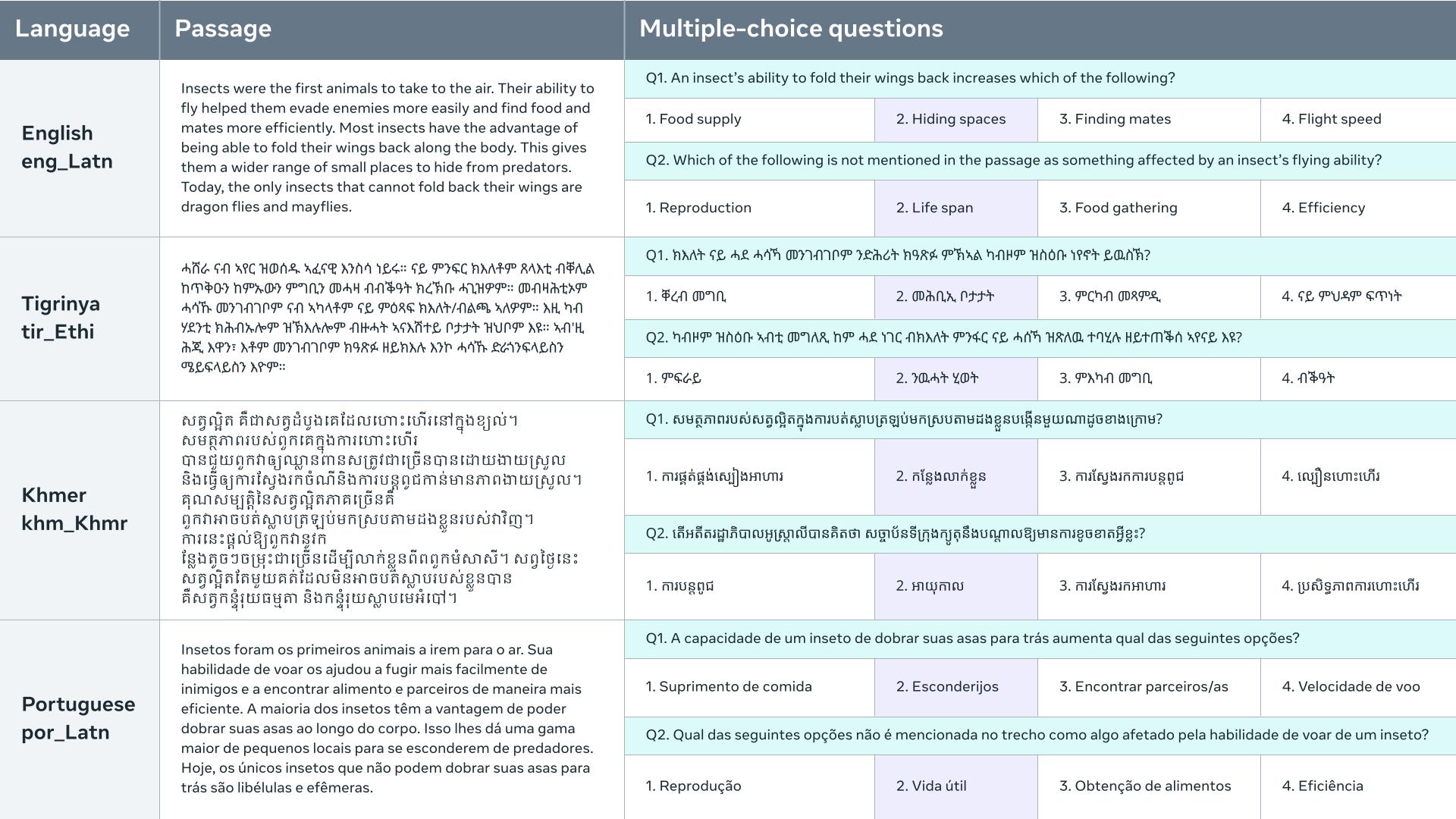
Notably, BELEBELE was created completely without machine translation, relying solely on human experts fluent in both English and each target language. This meticulous process aims to maximize quality and alignment across all translations.
The passages come from the FLORES-200 translation dataset, covering topics like science, technology, and travel. The questions were authored in English then carefully translated to each language, ensuring equivalence in difficulty and minimizing "translationese" effects. Questions often require understanding multiple sentences and ruling out distraction answers - challenging biases or shortcut strategies.
BELEBELE includes languages never before seen in an NLU benchmark, such as ones using non-Latin scripts like Cyrillic, Brahmic, Arabic, Chinese, Korean, Hebrew, and Amharic.
The questions in BELEBELE are intentionally challenging to test the limits of machine learning models' natural language understanding (NLU) capabilities. Researchers found that while human performance yielded an impressive 97.6% accuracy rate on a subset of English multiple-choice questions, the ROBERTA-base model only achieved a 71.7% accuracy. The results highlight the performance gap between humans and models, showcasing room for improvement.
BELEBELE serves to better understand how well models generalize across languages. According to the study, the performance of a language model depends on two key factors: the amount of pretraining data in the target language, and the level of cross-lingual transfer from languages in the pretraining data to the target language. This study provides detailed insights into how these factors impact multilingual capabilities among MLMs and LLMs.
One striking difference was the data distribution used during pretraining. LLMs like Llama-2-chat that are primarily pretrained in English, outperformed MLMs like XLM-R in English, but showed significant drops in performance when applied to non-English languages.
Despite this gap however, the researchers note that LLMs like Llama 2 and FALCON still demonstrated unexpectedly robust comprehension across a large number of languages. Llama-2-chat is above 35% accuracy (i.e. 10 points above the random baseline) for 59/122 languages and above 50% accuracy for 33 languages. "This shows that English-centric LLMs pretrained model are a promising starting point to build multilingual models."
The introduction of BELEBELE aims to catalyze advancements in high-, medium-, and low-resource language research. It also highlights the need for better language identification systems and urges language model developers to disclose more information about their pretraining language distributions.
The breadth of linguistic diversity captured in BELEBELE makes it a significant step towards inclusive AI systems that work equally well across the world’s cultures and languages.