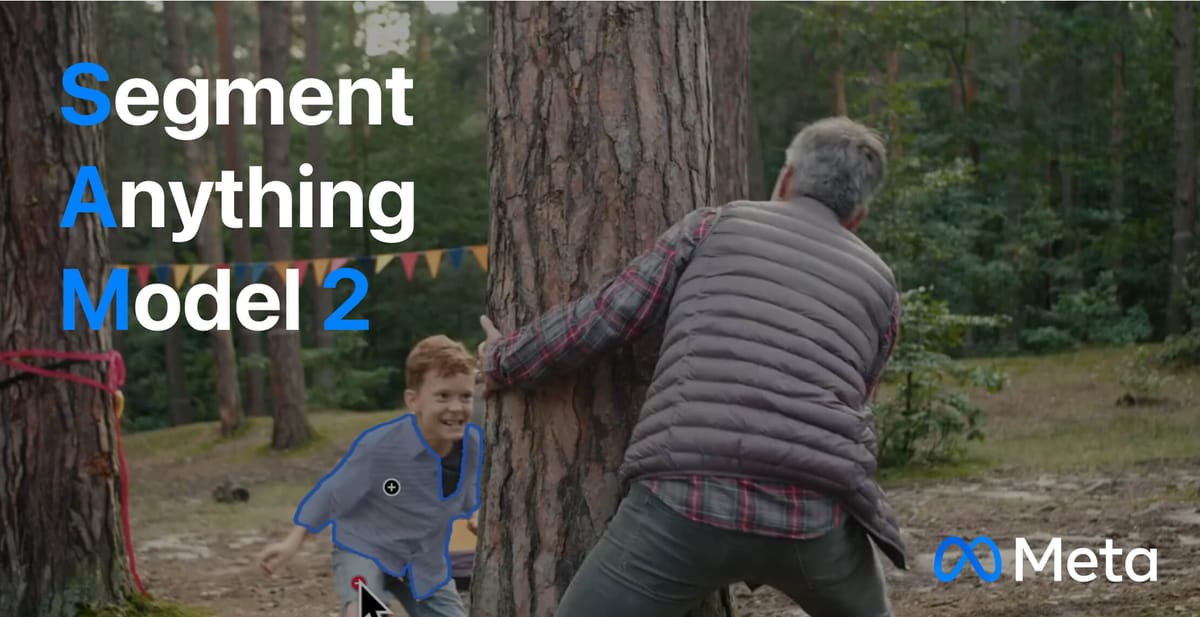
Meta has just announced the Segment Anything Model 2 (SAM 2) at SIGGRAPH. This new model builds upon the success of its predecessor by unifying image and video segmentation capabilities into a single, powerful system.
SAM 2 represents a major advancement in the field, offering real-time, promptable object segmentation for both static images and dynamic video content. The model's architecture employs a innovative streaming memory design, allowing it to process video frames sequentially. This approach makes SAM 2 particularly well-suited for real-time applications, opening up new possibilities across various industries.
In benchmark tests, SAM 2 has demonstrated superior performance, outpacing previous approaches in both accuracy and speed. Perhaps most impressively, the model exhibits remarkable versatility, capable of segmenting virtually any object in images or videos – even those it has never encountered before. This flexibility eliminates the need for custom adaptation to specific visual domains, making SAM 2 a truly general-purpose tool.
Staying true to Meta's commitment to open source AI, SAM 2 is being released under an Apache 2.0 license. This decision allows developers and researchers worldwide to freely build upon and integrate the technology into their own projects, potentially accelerating innovation across the field.
Alongside the model itself, Meta is introducing SA-V, a substantial new dataset designed to push the boundaries of video segmentation research. SA-V comprises approximately 51,000 real-world videos and over 600,000 spatio-temporal masks, providing a rich resource for training and evaluating future segmentation models.
The implications of SAM 2 are far-reaching. In video editing, for instance, the model's ability to segment objects across entire clips with minimal user input could dramatically streamline workflows. Similarly, fields like autonomous vehicles, robotics, and scientific research stand to benefit from SAM 2's powerful analytical capabilities.
However, Meta has pointed out some areas in which SAM2 still struggles. For example, the model can struggle with accurately tracking objects across drastic camera viewpoint changes, during long occlusions, or in crowded scenes. It may also have difficulty precisely segmenting objects with very thin or fine details, especially when they are fast-moving. Additionally, while SAM 2 can track multiple objects simultaneously, it processes each object separately, which may impact efficiency in complex scenes with numerous objects. Meta acknowledges these challenges and suggests that incorporating more explicit motion modeling could help mitigate some of these issues in future iterations.
Nevertheless, SAM 2 is a big deal for the field of computer vision. Once researchers and developers get their hands on it, we will likely see a new wave of more intelligent systems that can better understand and interact with visual information in increasingly sophisticated ways.
Meta has released the model, the dataset, a web-based demo, and the research paper.