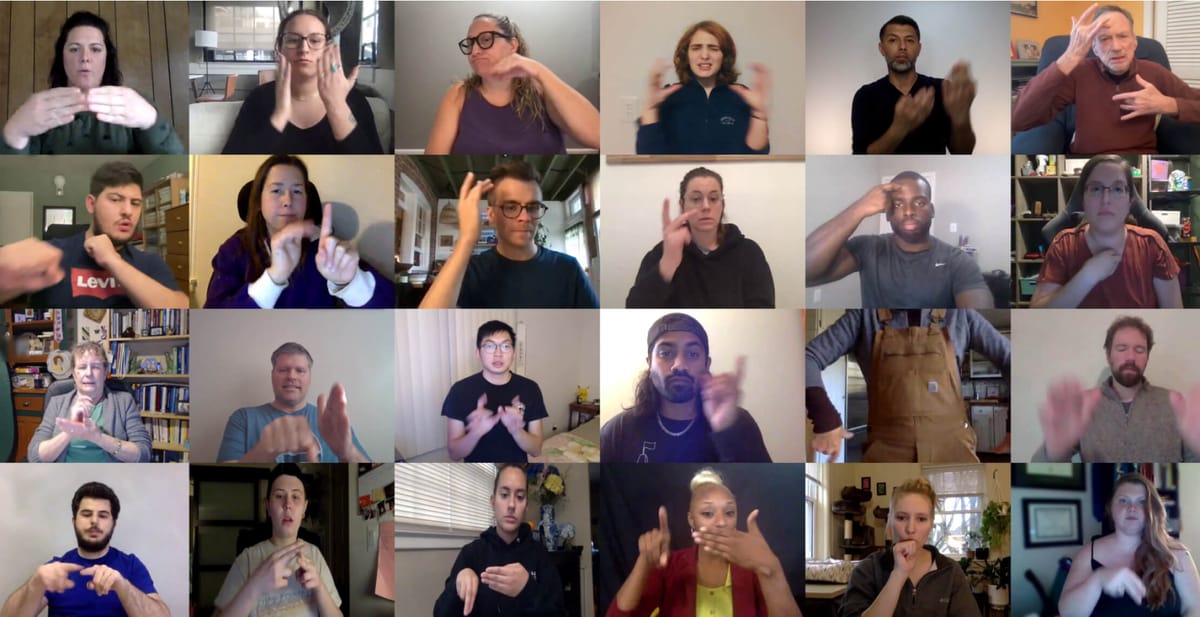
Microsoft researchers have introduced a first-of-its-kind crowdsourced dataset to advance sign language recognition capabilities of artificial intelligence systems. The dataset, called ASL Citizen (released earlier this year), aims to expand information accessibility for the 70 million deaf individuals worldwide who use sign language as their primary means of communication.
The project builds on Microsoft's mission of societal empowerment and researcher Danielle Bragg's focus on advancing sign language technology through human-centered, interdisciplinary work.
As detailed in a recent research paper, ASL Citizen contains over 84,000 video samples of American Sign Language (ASL) signs collected from contributors through a custom crowdsourcing platform. It is now the largest dataset available for training isolated sign language recognition (ISLR) algorithms.
According to Bragg, the principal researcher on the project, existing sign language datasets have suffered from small size, limited diversity, lack of real-world settings, and consent issues. For example, some datasets scrape videos from the internet without permission, while others rely on small in-person lab recordings.
To overcome such limitations, the researchers designed a community-focused web platform that enabled distributed contributers to securely submit videos within their own environments. The interface also ensured pre-labeled data by asking users to record preset signs.
Through an iterative design process involving community members, the platform enabled efficient accumulation of a large, diverse training dataset grounded in real-world contexts.
Using ASL Citizen, the team established new deep learning baselines for sign lookup tasks. Despite testing on entirely novel signers, models trained on the crowdsourced data increased isolated sign recognition accuracy from 16% to 71% compared to prior datasets.
"Even without algorithmic advances, training and testing on ASL Citizen improves ISLR accuracy," explained Bragg. The paper frames ISLR as a practical dictionary search problem to assist deaf users.
Deaf researchers and community organizations played an integral role throughout the project's development. "Deaf research team members were involved throughout," said Bragg, also highlighting collaborators from Boston University and the University of Washington.
By embracing community participation and focusing on consent, the crowdsourcing methodology behind ASL Citizen demonstrates that inclusive data collection can advance accessibility while producing state-of-the-art machine learning results. Microsoft has open-sourced the dataset with a non-commercial license to support further research.