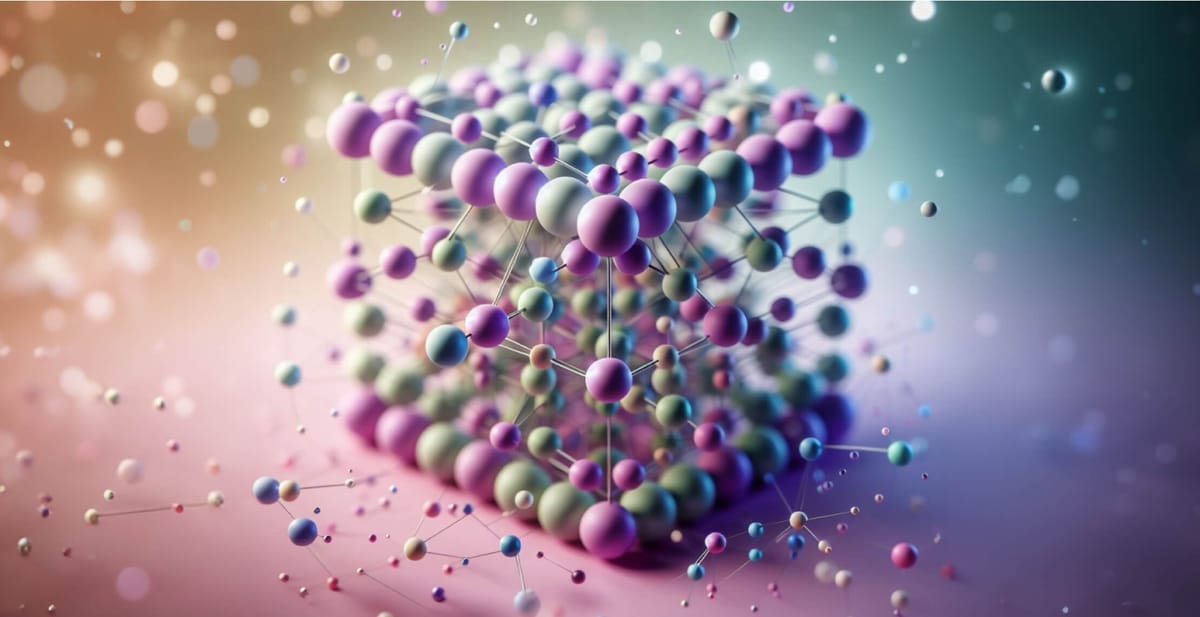
Microsoft Research AI4Science has unveiled MatterGen, a new generative AI model designed to accelerate the discovery of novel materials with desired properties. MatterGen represents an innovative application of the latest advances in generative AI to one of the biggest challenges in materials science.
The key innovation of MatterGen is its ability to directly generate hypothetical materials that satisfy specified property constraints, rather than the traditional approach of randomly searching for materials then filtering based on properties. This is analogous to using AI image generation models like DALL·E 3 to create a picture of a cat, rather than generating random images and picking out the one with a cat.
Specifically, MatterGen is a diffusion model fine-tuned with adapters to account for constraints including chemistry, symmetry, and target properties like magnetism, electronic properties, and mechanical strength. Compared to prior generative models, MatterGen produces materials much closer to stable ground states, making them more promising candidates for experimental validation.
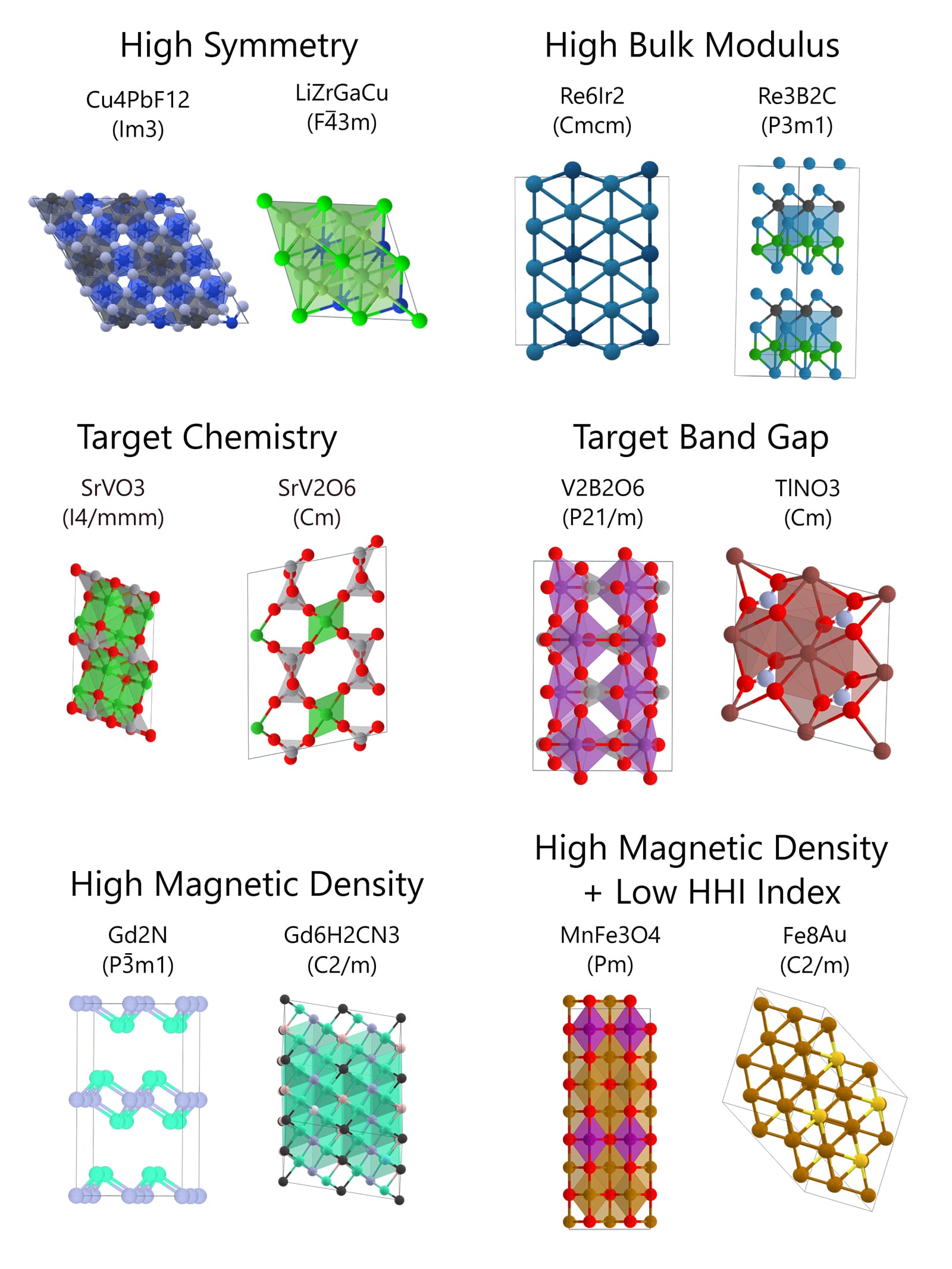
In evaluations based on density functional theory (DFT) computations, MatterGen achieved substantially higher yields of stable, novel materials meeting desired criteria compared to baseline approaches:
- 2.9x more stable, unique structures than a leading generative model
- 17.5x closer to local energy minimum
- Over 250 high bulk modulus materials, vastly exceeding screening methods
Additionally, MatterGen excelled at multi-constraint materials discovery problems like identifying magnet materials with reduced supply chain risks. This showcases how AI-guided generation can explore vast materials design spaces supporting real-world manufacturing needs.
Microsoft views MatterGen as validation of AI's potential to unlock new materials breakthroughs once considered intractable. By directly generating hypothetical materials optimized to satisfy multiple constraints, MatterGen provides a more targeted approach compared to exhaustive search and filtering. This represents a promising new paradigm for accelerated discovery by better aligning AI capabilities with the most pressing real-world needs.
The MatterGen team aims to continue enhancing the model's capabilities and pursuing physical validation to drive impact. For any revolutionary new material MatterGen proposes, experimental confirmation will be the ultimate test. Microsoft is optimistic about the prospects while acknowledging the hard work ahead to transform proofs of concept into solutions making a tangible difference. Still, MatterGen stands as an encouraging indicator of how AI could redefine what's possible, not just in materials science but any field placing heavy reliance on search and simulation.