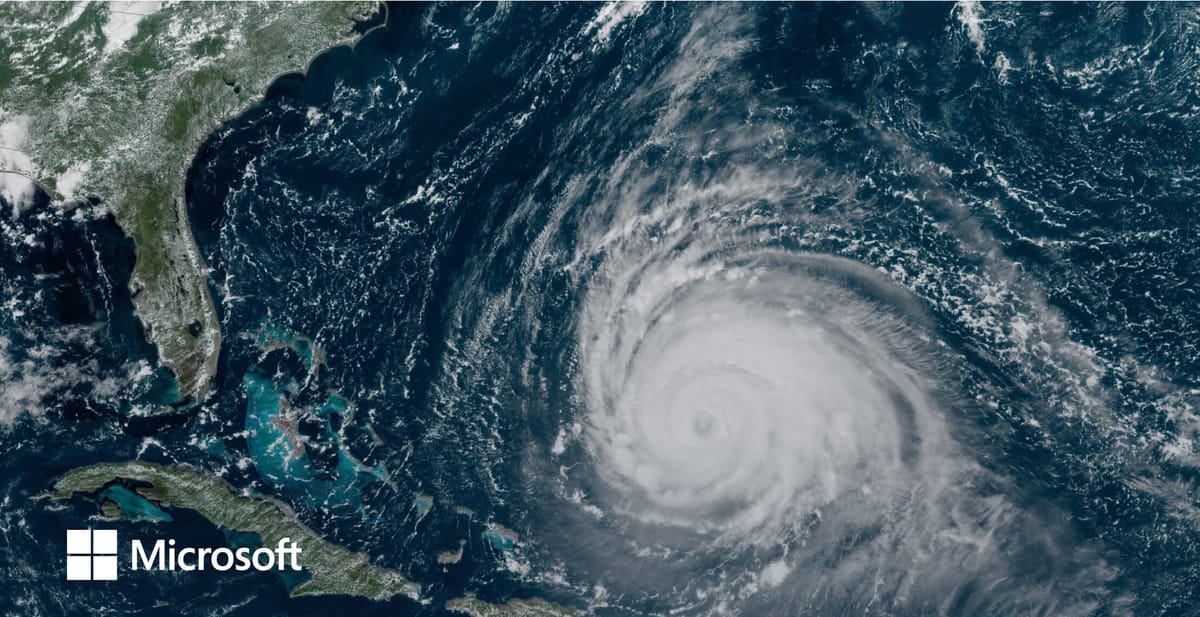
Microsoft researchers have unveiled Aurora, an AI model that could transform weather forecasting and air pollution monitoring on a global scale. By leveraging vast amounts of atmospheric data and innovative deep learning techniques, Aurora produces highly accurate predictions at unprecedented speeds, paving the way for a new era in environmental modeling.
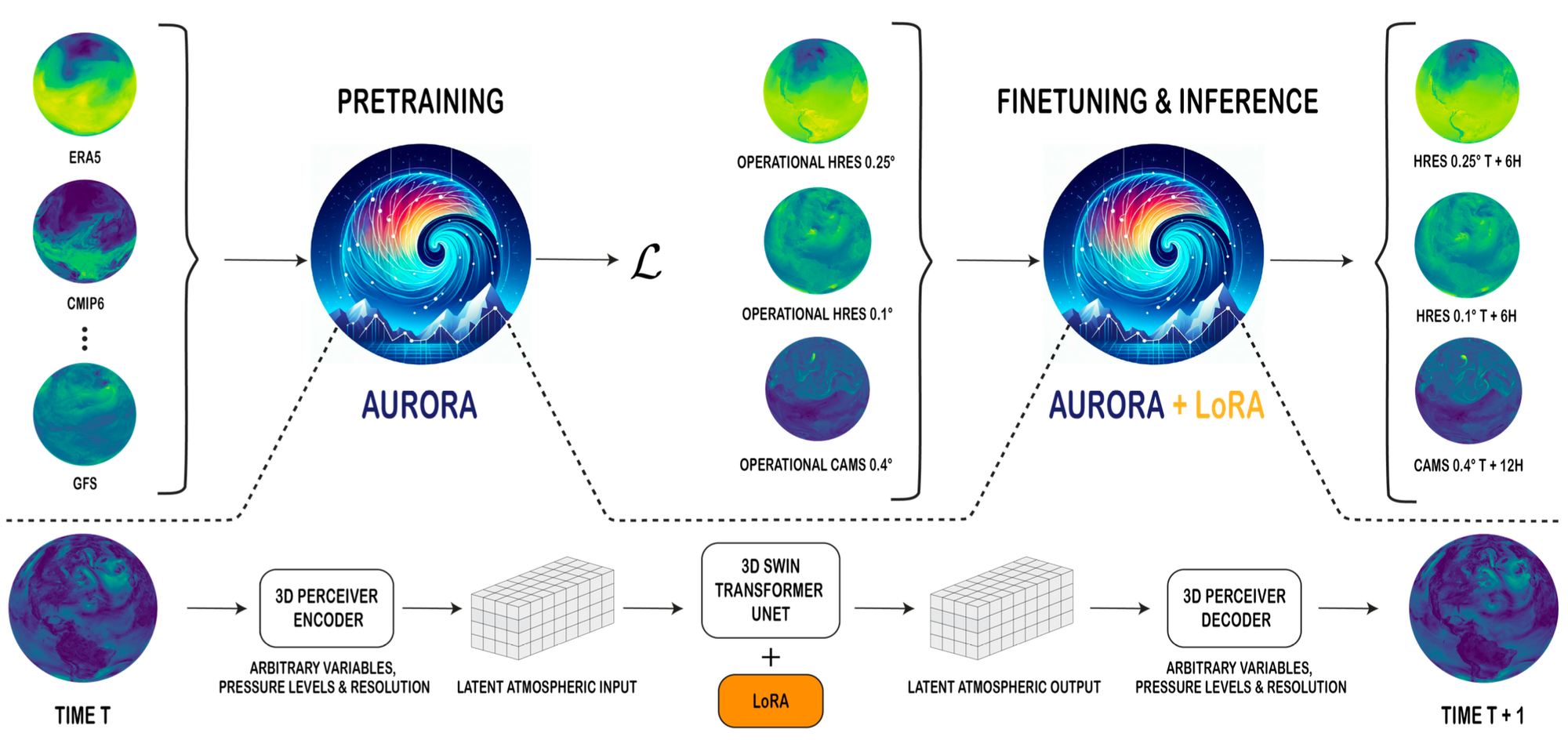
Aurora is a 1.3 billion parameter model built using a flexible 3D Swin Transformer architecture with Perceiver-based encoders and decoders. It leverages over a million hours of diverse weather and climate data, making it incredibly adept at predicting atmospheric conditions.
But what really sets Aurora apart is its speed and accuracy. It can generate a 5-day global air pollution forecast and a 10-day high-resolution weather forecast in under a minute, outperforming traditional numerical models like IFS-HRES and even Google’s GraphCast on most metrics. Its computational speed is estimated to be 5000x faster than the Integrated Forecasting System (IFS).
One of the key strengths of Aurora lies in its extensive pretraining process. The model was trained on over a million hours of diverse weather and climate simulations, enabling it to develop a comprehensive understanding of atmospheric dynamics. This pretraining allows Aurora to generalize well to new scenarios, even with limited data, making it a powerful tool for regions where observational data may be scarce.
It uses a combination of 3D transformers and neural simulators, optimized to handle a variety of atmospheric data. This includes everything from surface-level weather variables to complex atmospheric chemistry. By learning from an extensive dataset that includes ERA5 reanalysis data, climate simulations, and operational forecasts, Aurora develops a robust understanding of atmospheric dynamics.
Comparing Aurora to Other AI Models
And while Aurora is not alone in the race to harness generative AI for weather prediction, it distinguishes itself from competitors like Google DeepMind's GraphCast and NVIDIA's FourCastNet through its exceptional speed and comprehensive data processing capabilities.
GraphCast: While GraphCast is highly regarded for its skillful medium-range global weather forecasts, it is trained on a single dataset, ERA5, at a lower resolution of 0.25 degrees. Aurora's pretraining on a diverse range of datasets allows it to capture more complex atmospheric patterns and generalize better to new situations. This allows Aurora to make more accurate predictions, especially in predicting upper atmospheric conditions and extreme weather events.
FourCastNet: NVIDIA’s FourCastNet, another formidable model uses a different architecture called Adaptive Fourier Neural Operators, which is designed to handle complex geometric data. It excels in high-resolution weather predictions but operates at a 0.25-degree resolution, limiting its ability to capture rapid onset phenomena as effectively as Aurora’s 0.1-degree resolution.
Moreover, Aurora's ability to predict global air pollution levels sets it apart from other models, which primarily focus on weather forecasting.
Performance and Efficiency
In terms of performance, Aurora is nothing short of impressive. It surpasses the accuracy of the state-of-the-art numerical model IFS-HRES in 92% of variables and outperforms Google’s GraphCast in 94% of targets. In a case study, Microsoft showed that Aurora successfully predicted the rapid intensification and peak wind speeds of Europe's 2023 storm Ciarán, showcasing its potential for mitigating the impacts of severe weather and saving lives. Additionally, Aurora’s predictions for air pollution levels are superior to those of CAMS in 74% of cases, thanks to its ability to process and analyze highly heterogeneous data.
Perhaps most impressively, Aurora achieves all of this with unprecedented computational efficiency. Its predictions are approximately 5000 times faster than numerical models at comparable accuracy levels. This speed-up could democratize access to accurate weather and air quality information, particularly in developing nations and remote regions where computational resources may be limited.
What's the Takeaway?
Aurora's speed and accuracy could transform weather forecasting, especially in data-sparse regions like the developing world and polar areas. By providing timely and accurate forecasts, it can help communities better prepare for extreme weather events, ultimately saving lives and reducing economic losses. Moreover, Aurora’s ability to predict air pollution levels could play a crucial role in public health, offering early warnings for high pollution events .
It also goes without saying, that the implications of Aurora could extend far beyond atmospheric science. By demonstrating the immense potential of AI in understanding and predicting complex systems, this research opens doors to applications in fields ranging from oceanography to hydrology to ecology. As we grapple with the challenges posed by climate change, tools like Aurora could prove invaluable in building a more resilient and sustainable future for our planet.