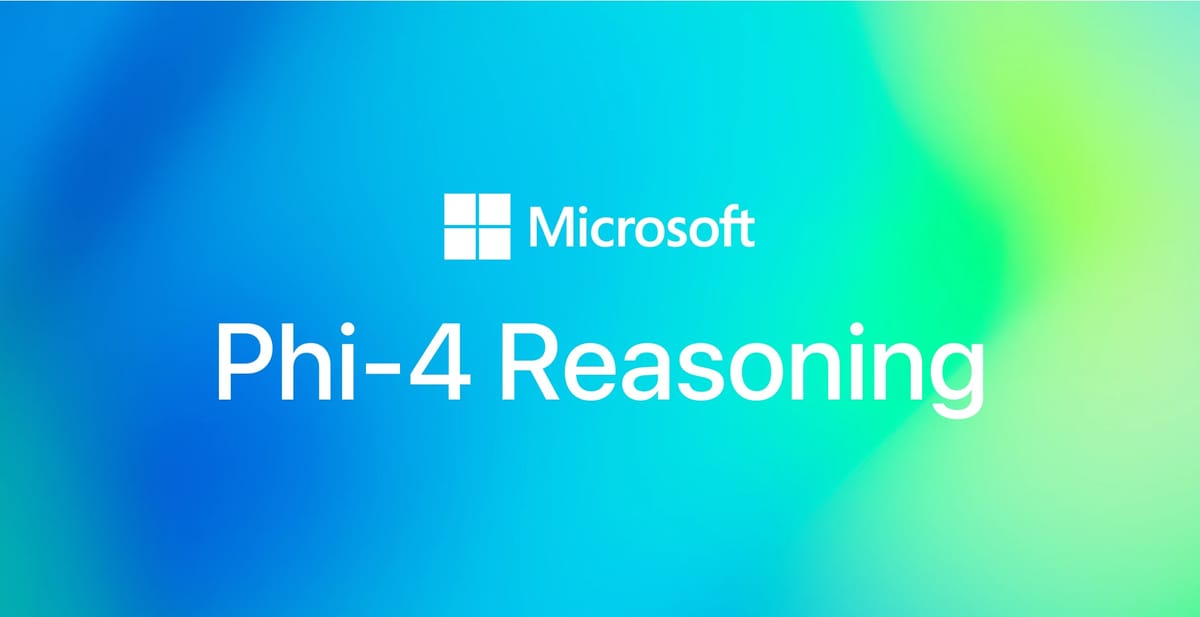
For businesses looking to adopt AI without breaking the compute bank—or shipping their data to the cloud—Microsoft's Phi family is very compelling. On Wednesday, the company released three new open-weight small language models: Phi-4-reasoning
, Phi-4-reasoning-plus
, and Phi-4-mini-reasoning
.
Key Points:
- The Phi-4-reasoning models mark a new era for small language models with impressive reasoning capabilities
- Despite being only 14B parameters, Phi-4-reasoning outperforms much larger models on math and science reasoning tasks
- These models are designed for both cloud and on-device deployment, with optimized versions for Copilot+ PCs
Let’s start with the pitch: Phi-4-reasoning is a 14-billion parameter model that outperforms OpenAI’s o1-mini and DeepSeek-R1-Distill-Llama-70B. It even edges out DeepSeek’s full 671B MoE model on the AIME 2025 math benchmark. That’s not a typo—a model about 1/50th the size is beating one of the biggest open models on the board. And it does all that while being small enough to run efficiently on modest hardware.
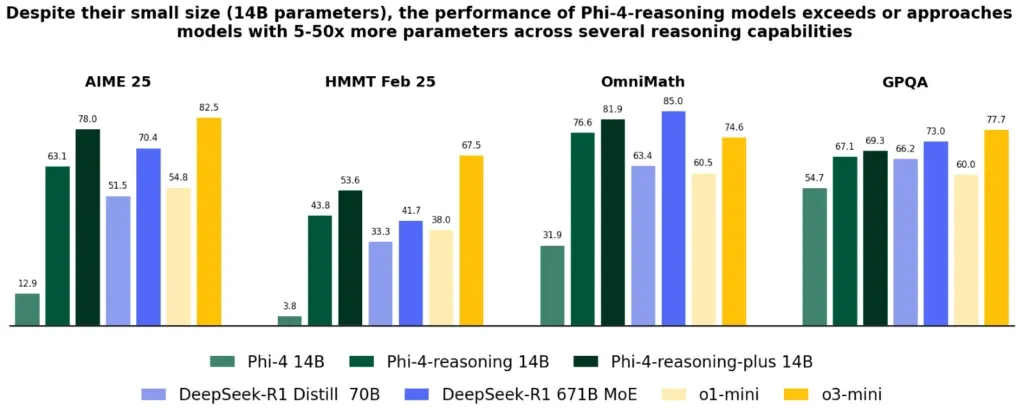
For businesses, that’s not just a technical flex—it’s a strategic advantage.
The models are designed for inference-time scaling. That means they can adapt their compute usage depending on the task at hand—lean for simple queries, full-throttle for complex reasoning. This makes them ideal for scenarios where latency, cost, or privacy are concerns: think financial modeling, offline productivity tools, industrial systems, and even customer service chatbots.
The models are fully open-weight and available via Azure AI Foundry and HuggingFace. That makes them fine-tuneable, auditable, and deployable in a wide range of environments—without navigating API rate limits or licensing constraints. Want to drop a model into your CRM system or build a custom tutor that runs on a tablet? With Phi-4-mini-reasoning—just 3.8B parameters and outperforming models twice its size on math tasks—you can.
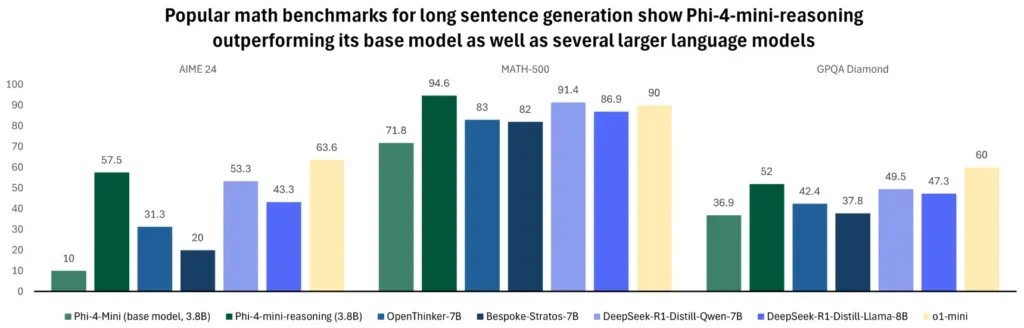
Perhaps most importantly, Microsoft isn’t just releasing these as research artifacts. They’re already integrated into Windows via the Copilot+ PC line, using a low-latency variant called Phi Silica. That means real products—like Outlook’s offline summarization and on-screen text assistants—are already powered by this tech. For IT leaders, this suggests a future where enterprise-grade AI is embedded directly in end-user devices, reducing reliance on cloud compute and enabling better data governance.
The underlying secret of the Phi-4 models isn’t raw size—it’s training discipline. Microsoft used a pipeline built almost entirely on synthetic, reasoning-focused data. Then, it applied a novel technique called Pivotal Token Search (PTS), which pinpoints the most consequential decision points in a model’s reasoning and fine-tunes accordingly. That kind of targeted training gives these small models surprising depth, especially in STEM tasks and logic-heavy scenarios.
The result: these models behave like much larger systems but come with lower cost, faster response times, and more deployment flexibility. If your business has been holding back on integrating AI due to privacy, latency, or cost constraints—this is the moment to re-evaluate.
It’s clear that Microsoft sees a future where small models do more heavy lifting—on devices, in apps, and across edge environments. And with Phi-4, they’ve made a compelling case that small doesn’t mean simple. With AI now dominated by trillion-parameter behemoths, Phi-4 is a reminder that quality—especially in data and training—is sometimes more powerful than raw size.