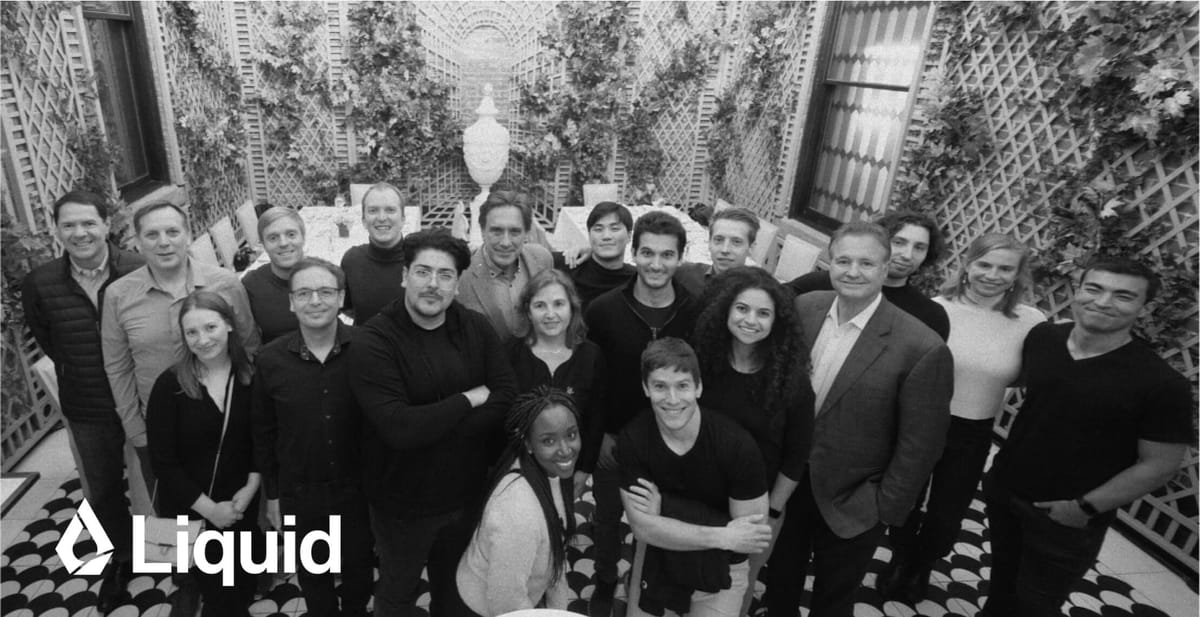
Liquid AI, an MIT spinoff co-founded by esteemed robotics pioneer Daniela Rus, emerged from stealth mode this week with $37.5 million in seed funding to develop a "new generation" of AI powered by liquid neural networks.
The startup aims to commercialize the relatively new liquid neural network architecture, which was invented at Vienna University of Technology, Austria and refined and scaled by MIT's Computer Science and Artificial Intelligence Laboratory (CSAIL). Liquid neural networks are more efficient, adaptable, and interpretable than traditional AI models.
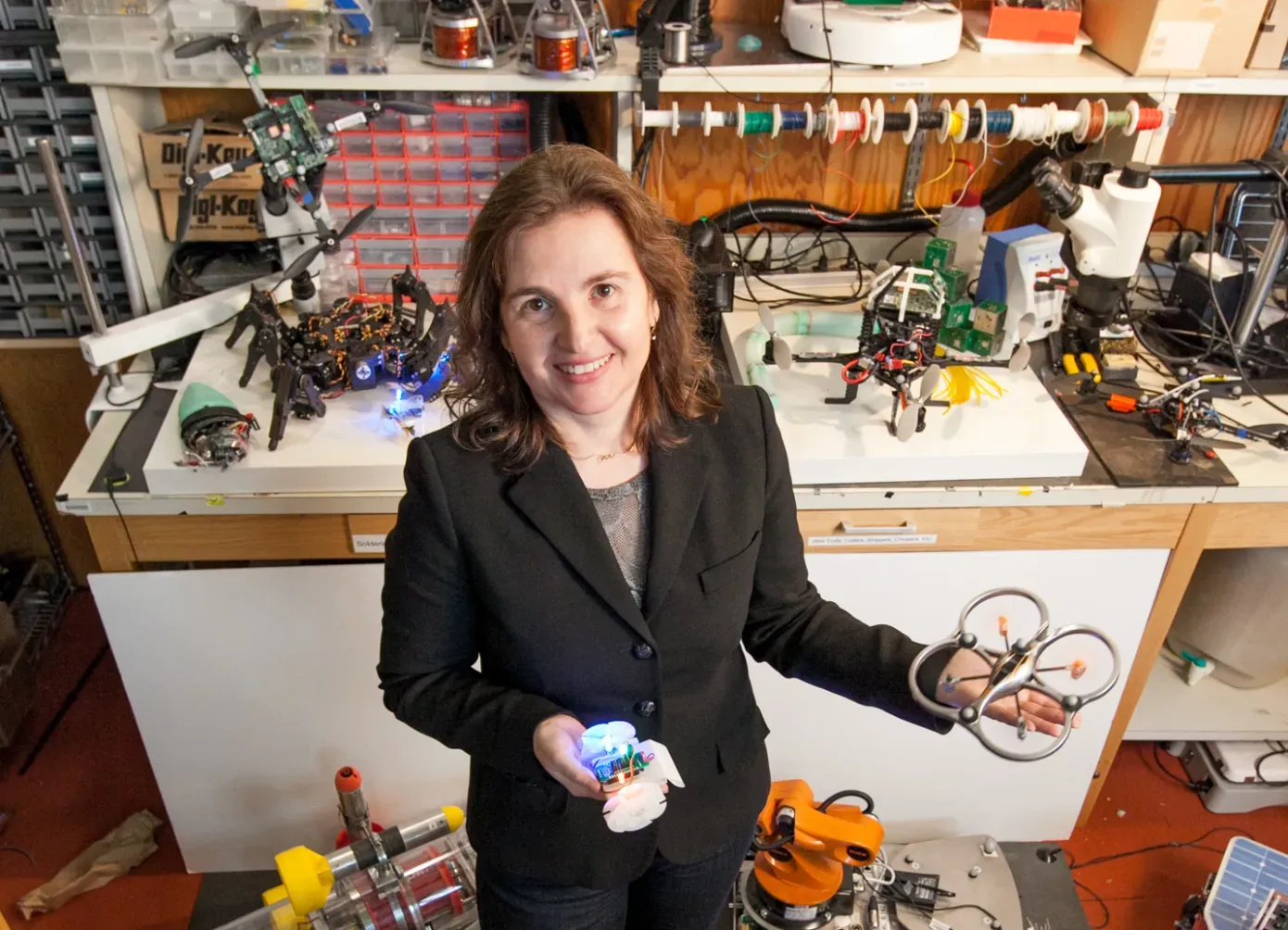
"We are developing a new generation of AI foundation models built from first principles – going beyond generative pre-trained Transformers (GPTs)," said Liquid AI in its launch announcement. "Liquid AI models build on a framework that leads to causality, interpretability, and efficiency."
The seed round, which values Liquid AI at $303 million post-money, was led by OSS Capital and PagsGroup. Other notable investors include GitHub co-founder Tom Preston Werner, Shopify co-founder Tobias Lütke, and Red Hat co-founder Bob Young.
The concept of liquid neural networks, dating back to initial research in 2018 and gaining significant attention with the "Liquid Time-constant Networks" paper in 2020, represents a shift in AI modeling. As described by Hasani in a TEDx talk at MIT, these networks remain adaptable even after training, adjusting themselves based on incoming inputs. The term 'liquid' aptly refers to their flexibility and adaptability.
What sets liquid neural networks apart is their size and the rich, focused functionality of their nodes. For instance, MIT demonstrated the driving of a car using liquid neural networks with only 19 nodes, a stark contrast to larger, noisier networks. Each node in these networks is described by differential equations, allowing for a precise approximation of the system's dynamics. This approach results in faster and less computationally expensive operations.
Liquid neural networks offer significant advantages in the field of robotics, particularly in terms of computational requirements. They can operate on less computing power, potentially using simple hardware like a Raspberry Pi for complex reasoning tasks. This capability opens up new possibilities for mobile robotic systems that face hardware constraints.
One of the key benefits of liquid neural networks is their interpretability. Due to their smaller size, understanding how individual neurons combine to create outputs is more feasible than with larger networks. However, these networks require "time series" data, which means they don't currently work with static images but need sequential data like video. As Hasani notes, "The real world is all about sequences," highlighting the relevance of time series data in creating our perception of reality.
In recent tests by the Liquid AI team, liquid neural networks outperformed other state-of-the-art algorithms in time series prediction tasks and achieved promising results in real-world autonomous drone navigation.
“Liquid’s approach to advancing the state-of-the-art in AI is grounded in the integration of fundamental truths across biology, physics, neuroscience, math, and computer science," said OSS Capital founder Joseph Jacks.
The MIT spinoff plans to offer private, on-premises AI infrastructure as well as a platform for customers to build their own liquid neural network models. Areas of application could include electric grids, medical data, financial transactions, and weather forecasting.
Liquid AI's founding team includes CEO Ramin Hasani, CTO Mathias Lechner, Chief Scientific Officer Alexander Amini, and MIT Professor Daniela Rus. The company currently has 12 employees and expects to double its headcount in the coming months.