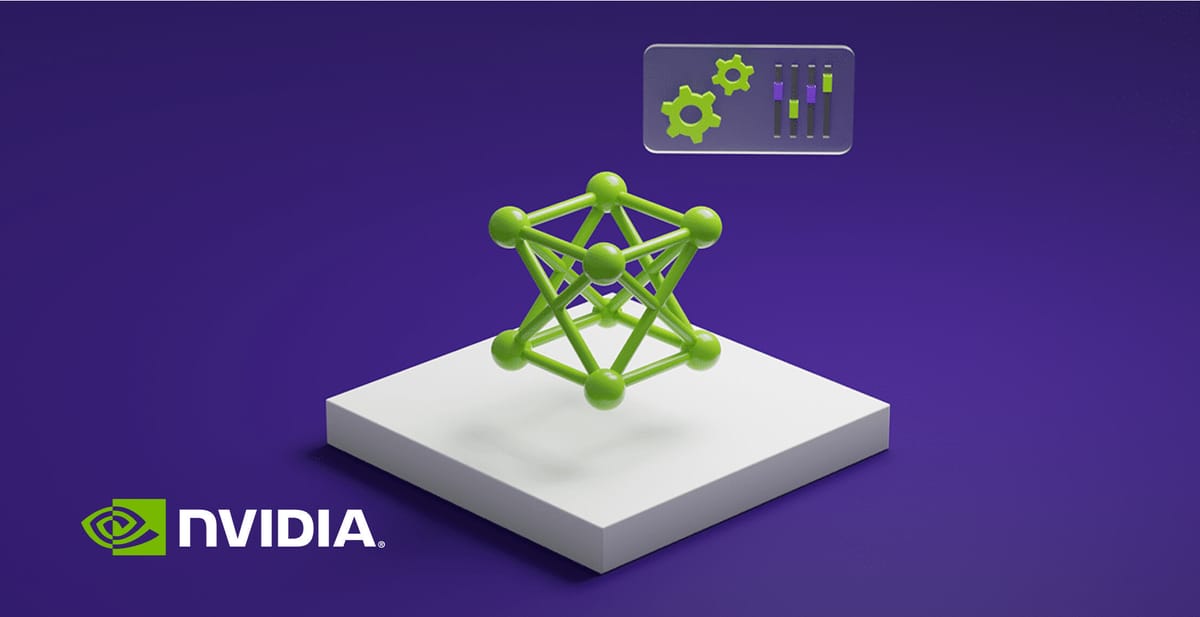
NVIDIA has released the Nemotron-4 340B model family under the permissive NVIDIA Open Model License Agreement. The family includes base, instruct, and reward models that push the boundaries of open-access AI while remaining highly efficient.
The Nemotron-4 340B models demonstrate impressive performance across a wide array of benchmarks, often outperforming existing open models. Remarkably, they achieve this while being optimized to run on a single NVIDIA DGX H100 system with just 8 GPUs. This efficiency makes them accessible to a broader range of researchers and developers. As you can see below, it outperforms Llama-3 70B and Mixtral 8X7B on quite a few benchmarks.
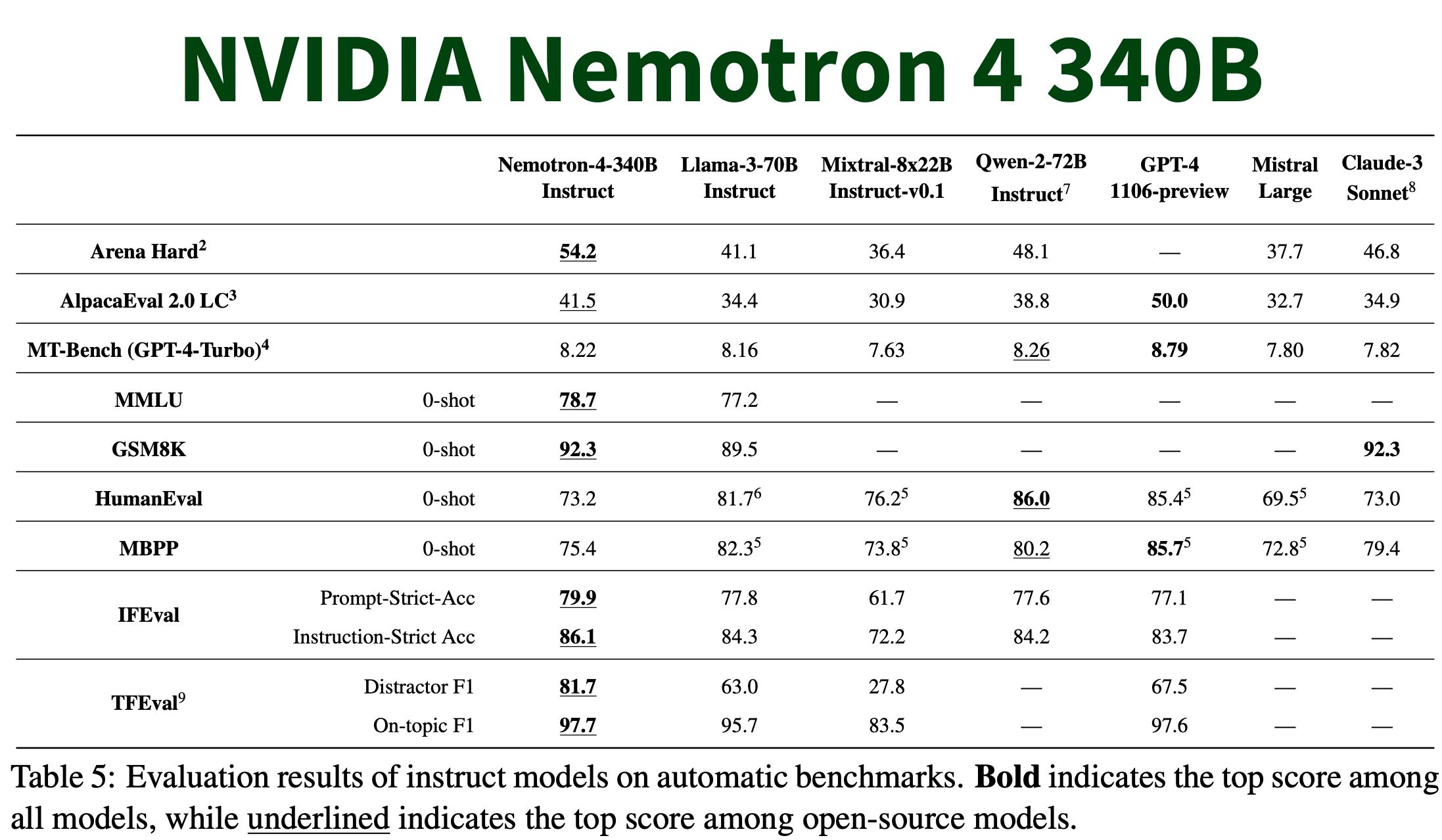
NVIDIA believes these models will greatly benefit the AI community in both research and commercial applications. One particularly exciting use case is generating high-quality synthetic data to train smaller, specialized AI models. In fact, synthetic data played a crucial role in the development of Nemotron-4 itself.
Over 98% of the data used to train the Nemotron-4 340B instruct model was synthetically generated using the base model and reward model. This showcases the immense potential of these models for creating valuable training data. Excitingly, NVIDIA is also open-sourcing the synthetic data generation pipeline used in this process, empowering others to leverage this approach.
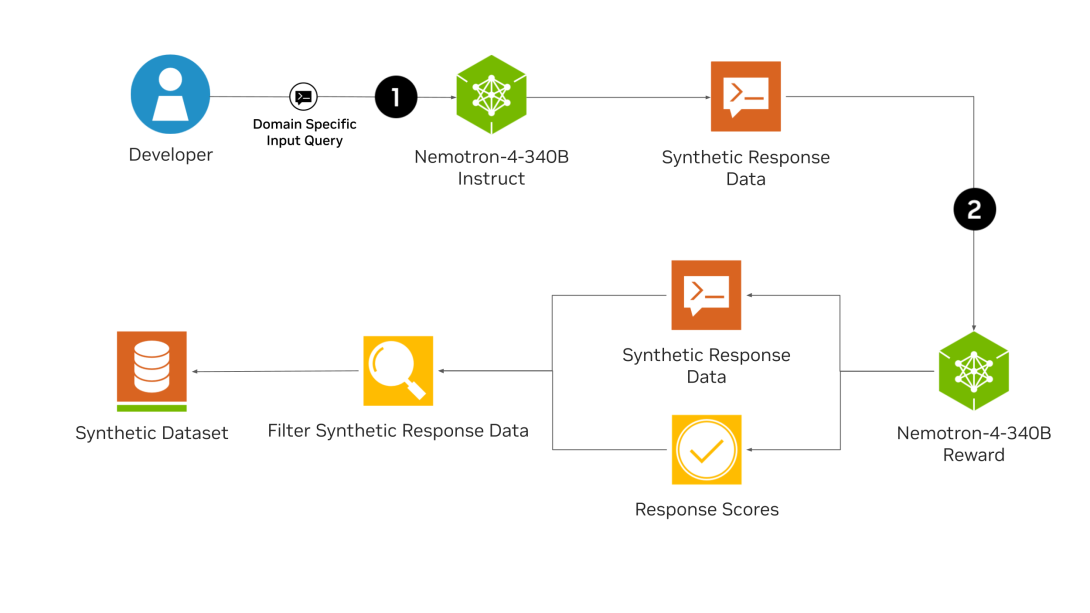
The Nemotron-4 340B base model was trained on a massive 9 trillion tokens, spanning a diverse range of English text, multilingual data, and programming languages. It utilizes a standard transformer architecture enhanced with cutting-edge techniques like grouped query attention and rotary position embeddings.
Building on this strong foundation, the instruct model underwent supervised finetuning and preference optimization using a combination of human-annotated and synthetic data. NVIDIA developed a novel "iterative weak-to-strong alignment" approach, using each generation of models to create higher-quality synthetic data for training the next.
The Nemotron-4 340B reward model is a standout in its own right, claiming the top spot on the RewardBench leaderboard. By predicting rewards across multiple nuanced attributes like helpfulness, coherence and verbosity, it captures detailed quality scores that powered the optimization of the instruct model.
Benchmark evaluations confirm the impressive capabilities of the Nemotron-4 models. The base model goes toe-to-toe with leading open models, the instruct model excels at following complex instructions and engaging in coherent dialogue, and the reward model even surpasses some well-known proprietary systems. Human assessments further validate the models' strong performance.