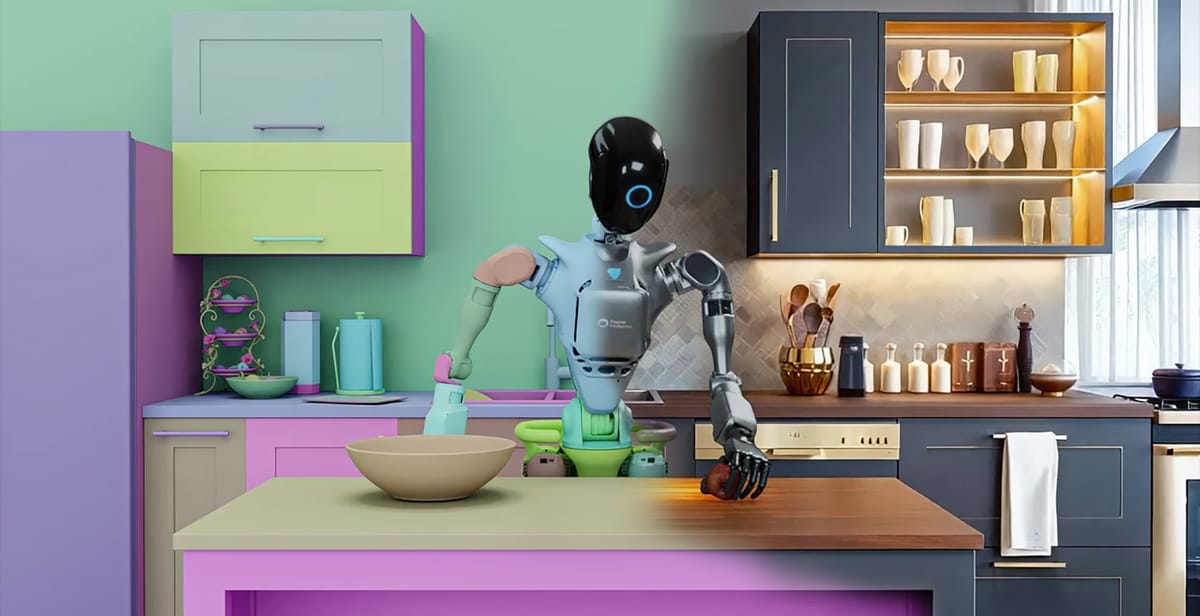
At GTC 2025, amid the headline-grabbing demos of chip roadmaps, humanoid robots, and eye-catching partnerships, a quieter but more profound narrative emerged about NVIDIA's strategic vision. The company isn't just creating tools for AI - it's architecting an entire ecosystem for the next computing paradigm: physically embodied artificial intelligence.
Key Points:
- NVIDIA's "three computers" approach forms the foundation of their physical AI strategy
- Data strategy combining real and synthetic data represents a significant advantage
- Open-source initiatives like Newton and GR00T N1 are designed to expand the developer ecosystem
- Partnerships across industries reveal a comprehensive go-to-market strategy
During a conversation with Akhil Docca, NVIDIA's senior product marketing manager for Omniverse and Robotics, he framed the company's approach as a natural evolution. "If you saw how Jensen talked through the evolution of computing in general, we moved from computer vision to generative AI to agentic AI. Physical AI happens to be the next paradigm that we're focusing on," Docca explained.
But while NVIDIA may present physical AI as just one component of their broader strategy, the sheer scale of their investments tells a different story. The company has methodically assembled the building blocks of a comprehensive physical AI ecosystem, creating what might be the industry's most complete foundation for embodied intelligence.
At the core of this strategy is what Docca described as NVIDIA's "three computers" framework. The first computer trains robot brains using a combination of real and synthetic data. The second, simulation computer — powered by Omniverse — teaches robots to operate in virtualized environments. The third deploys these algorithms in the physical world.
"We're not trying to build the end solution," Docca told me. "We're trying to give developers the full-stack tools—chips, libraries, models, data pipelines—to do it themselves."
It's a playbook that echoes NVIDIA's rise in AI: make the expensive parts accessible, handle the complexity under the hood, and win by being indispensable.
This tripartite approach isn't just theory. NVIDIA has developed concrete products for each stage, from the GR00T N1 foundation model for robots to the Omniverse Mega blueprint for fleet simulation to the DRIVE AGX in-vehicle computer for autonomous vehicles.
Importantly, for models like GR00T N1, NVIDIA isn't just releasing the model, it's also releasing the synthetic data, simulation frameworks, and training blueprints used to build it—everything developers would need to fine-tune it for their own robots. The approach mirrors what pre-trained models like Llama and DeepSeek are doing for language models today: lowering the cost of entry and speeding up iteration.
The Data Question
But ask anyone in robotics and they'll tell you: real-world data is a bottleneck. It's expensive, messy, and often too sparse to train generalizable models. NVIDIA's answer is simulation and synthetic data generation—and it's going all-in.
With Omniverse, NVIDIA offers a photoreal, physics-accurate virtual environment where robots can be trained, tested, and validated. Add Cosmos, its world foundation model engine, and developers can generate diverse synthetic datasets at scale. Docca described the combination as essential for "bridging the diversity and edge-case gaps" in physical AI.
The Safety Question
However, testing physical AI in the real world is risky and expensive. That's why NVIDIA is making simulation the proving ground for safety.
"Safety is born in simulation," Docca repeated more than once. Omniverse and Cosmos aren't just training tools—they're guardrails. By iterating in virtual environments with accurate physics and photoreal conditions, developers can stress-test robots before a single screw is turned in the real world.
It's the same logic behind NVIDIA's automotive platform, where companies like GM and Uber Freight are using simulated worlds to test AV behavior under rare or dangerous conditions.
The Scale Question
So, what comes after teaching one robot to do one thing? Teaching fleets to collaborate.
That's where Mega comes in—NVIDIA's new Omniverse blueprint for testing multi-robot environments. Mega enables simulation of robot swarms, humanoid and mobile units working in tandem across digital twins of factories and warehouses. It's the kind of scaling logic NVIDIA excels at.
Docca framed this as a transition from "sovereign loops" (one robot in isolation) to "robot-ready facilities" where diverse agents interact.
The Big Picture
All these parts come together to reveal NVIDIA's real strategy: becoming the essential infrastructure layer for physical AI across industries. While companies like Google, Tesla and Boston Dynamics might build their own AI models and robots, NVIDIA aims to provide the ecosystem with all the tools that make their development possible.
NVIDIA's intent is to accelerate the entire field. "We're not competing with anyone," Docca said. "In fact, we're working with everybody."
The industry partnerships and platform adoption so far provide a hint as to how NVIDIA's strategy may fare. The company announced collaborations with General Motors for manufacturing, GE Healthcare for autonomous imaging, and numerous robotics developers. The healthcare applications are particularly noteworthy as they show how NVIDIA's physical AI approach extends beyond industrial settings into critical services.
The Road Ahead
After GTC, one thing was clear: NVIDIA isn't content with just building the best chips in the market. It's tackling the hard problems—building the infrastructure, data, and software to make physical AI scalable, safe, and accessible.
When asked about timelines for generalized robots, Docca acknowledged it would be "a journey." This measured perspective reflects the reality of the challenge. While today's autonomous mobile robots and industrial arms are already being deployed, widespread adoption of humanoid robots in everyday settings likely remains years away.
The impact of NVIDIA's approach could be transformative across multiple industries. In manufacturing, GM and Foxconn are already adopting Omniverse and Mega to simulate and optimize robot fleets. In healthcare, GE is leveraging NVIDIA's platform to develop autonomous imaging systems that could dramatically expand global access to diagnostic tools. In logistics, companies like Uber Freight are implementing NVIDIA's technology to address critical labor shortages.
There are still many technical hurdles to address when it comes to physical AI—from developing robust manipulation capabilities to ensuring safety around humans. NVIDIA will also face competition from vertically integrated players like Tesla, which builds its own chips, robots, and AI models, and from cloud giants like Google that have their own robotics ambitions.
Still, NVIDIA's holistic approach positions it uniquely at the intersection of hardware, software, and data—the three pillars of physical AI. That's not just a bet on robots. It's a bet on becoming the operating system for the physical world.