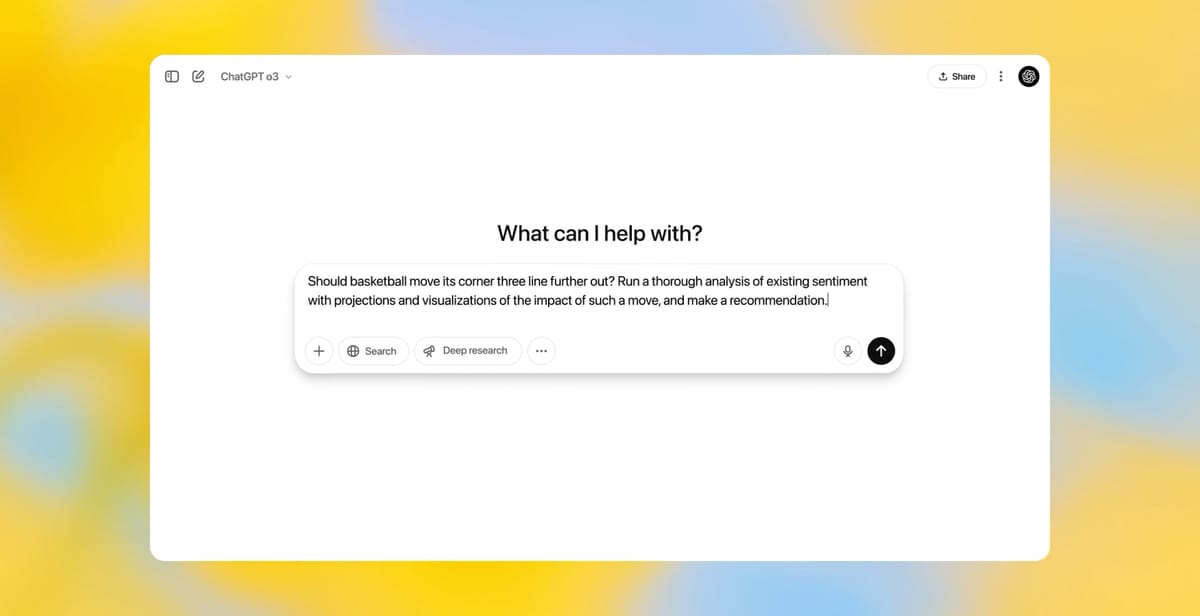
In what feels like a significant evolution of AI capabilities, OpenAI has released two new models that push the boundaries of what we've come to expect from large language models. The company's latest offerings—o3 and o4-mini—are being touted as their most capable releases to date, representing what OpenAI research lead Mark Chen described during the announcement livestream as "a qualitative step into the future."
Key Points:
- The models can use all ChatGPT tools including Python, image analysis, web search, and image generation
- First models to directly integrate images into their "chain of thought" reasoning process
- o4-mini achieves remarkable performance despite being smaller and more cost-efficient than o3
What makes these models particularly interesting isn't just their raw intelligence, but rather how they integrate with other tools. For the first time, OpenAI's reasoning models can independently decide when and how to use the full suite of ChatGPT tools—including web browsing, Python code execution, image analysis, and image generation—to solve complex problems.
"These aren't just models. They're really AI systems," explained Greg Brockman during the launch presentation. This distinction is subtle but important. While previous models could use individual tools when specifically prompted, these new models have been trained to reason about when and how to deploy tools based on the desired outcomes.
This capability is perhaps most evident in how the models handle visual information. Unlike previous iterations that could merely "see" images, o3 and o4-mini can "think with images" by manipulating them in their reasoning process—rotating, zooming, or transforming uploaded photos as part of problem-solving. This integration has led to state-of-the-art performance on multimodal benchmarks, allowing models to interpret even blurry or oddly oriented visuals with impressive accuracy.
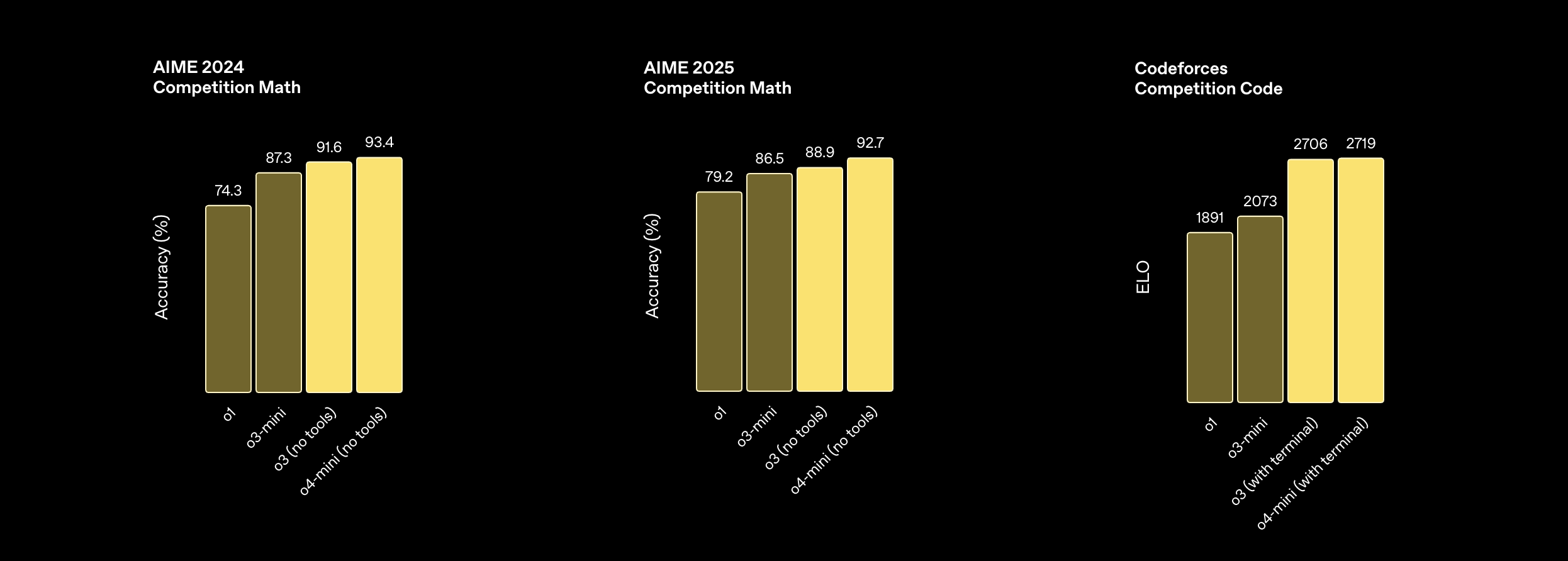
The benchmark results are indeed noteworthy. On AIME 2024, a challenging math competition, o4-mini achieved 93.4% accuracy without tools. On Codeforces, a competitive programming platform, o3 with terminal access reached an ELO of 2706—placing it among the top competitors globally. Perhaps most impressively, on PhD-level science questions in the GPQA Diamond benchmark, o3 without tools scored 83.3% accuracy, outperforming previous models by a significant margin.
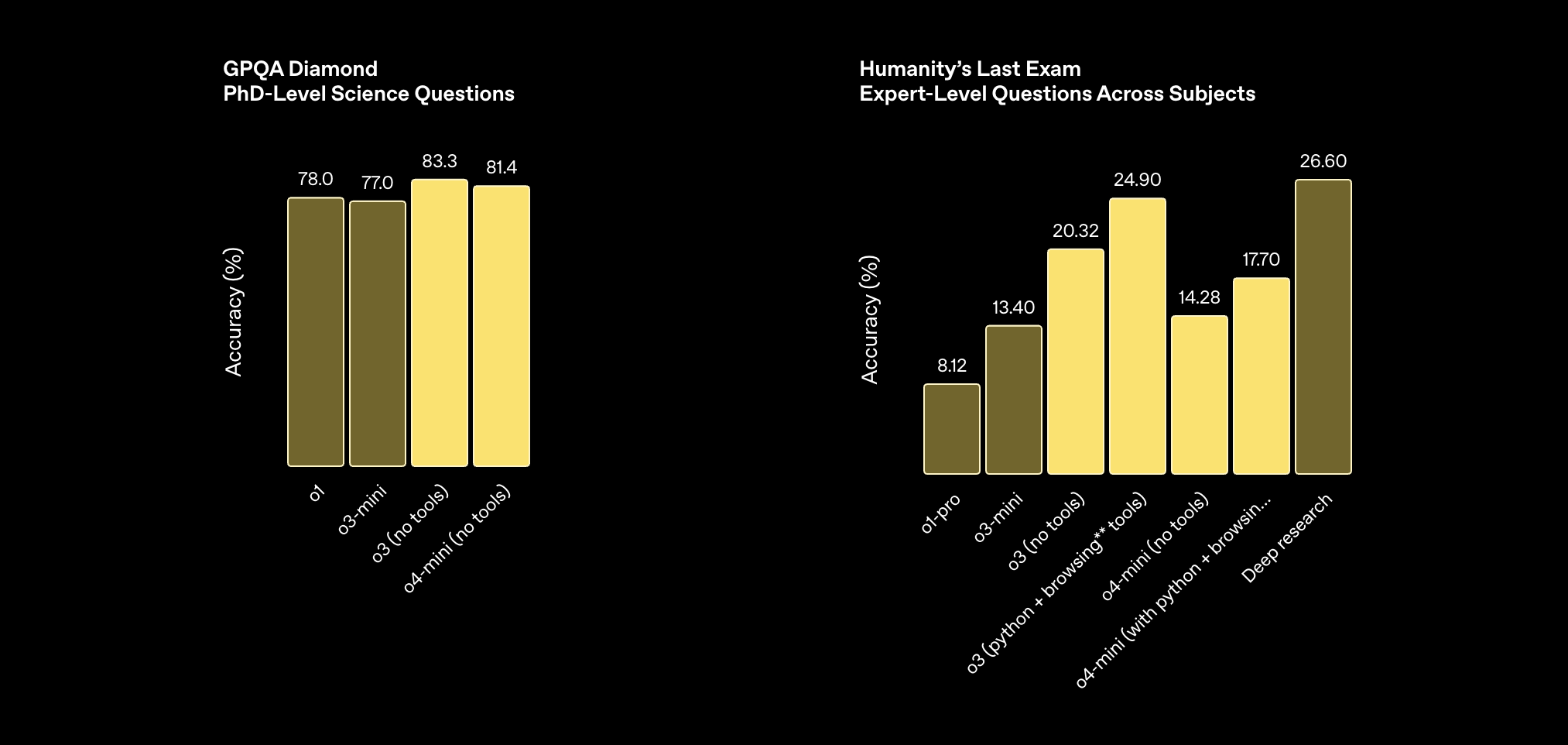
What's particularly remarkable about o4-mini is that despite being smaller and more cost-efficient than o3, it achieves comparable or even superior performance on certain benchmarks. This efficiency translates to higher usage limits, making it particularly valuable for high-volume applications.
Behind these improvements lies an order of magnitude increase in training compute compared to previous models. OpenAI reports investing more than 10 times the training compute of o1 to produce o3, with performance continuing to improve with additional resources—validating the company's belief that "more compute equals better performance" holds true for reinforcement learning just as it did for pre-training in the GPT series.
Perhaps the most intriguing demonstrations came from researchers showing how these models solve real-world problems. In one example, a researcher uploaded a blurry physics poster from a decade-old internship and asked the model to extract key information and compare it with recent literature. The model not only navigated the complex poster but also identified that the specific result wasn't included, proceeded to calculate it based on available data, and then compared it with current research—a task the researcher admitted would have taken him days.
The models also showed impressive coding capabilities. During the demonstration, o3 successfully debugged a complex issue in a Python mathematical package by navigating through source code, identifying inheritance problems, and applying the correct fix—all while running appropriate tests to verify the solution worked.
Alongside these releases, OpenAI announced a new developer tool called Codex CLI, a command-line interface that connects the models directly to users' computers. The tool allows models to run commands locally with safety constraints, potentially reshaping how developers interact with their machines. To support this vision, the company is launching a $1 million initiative to provide API credits for open-source projects using these new models.
For users, these models are already replacing previous versions in ChatGPT. Plus, Pro, and Team users now have access to o3, o4-mini, and o4-mini-high in the model selector, with Enterprise and Edu users gaining access next week. The models are also available to developers through the Chat Completions API and Responses API.
As impressive as these advancements are, they raise important questions about the evolving relationship between humans and increasingly capable AI systems. With models now able to chain hundreds of tool calls together and independently navigate complex decision trees, we're seeing the first glimpses of truly agentic AI—systems that can independently execute multi-step tasks on a user's behalf.
What's clear is that the boundary between "using a model" and "working with an AI system" continues to blur. As these systems become more capable of independent problem-solving, the relationship becomes increasingly collaborative rather than instructional—a shift that may fundamentally change how we think about AI tools in the years ahead.