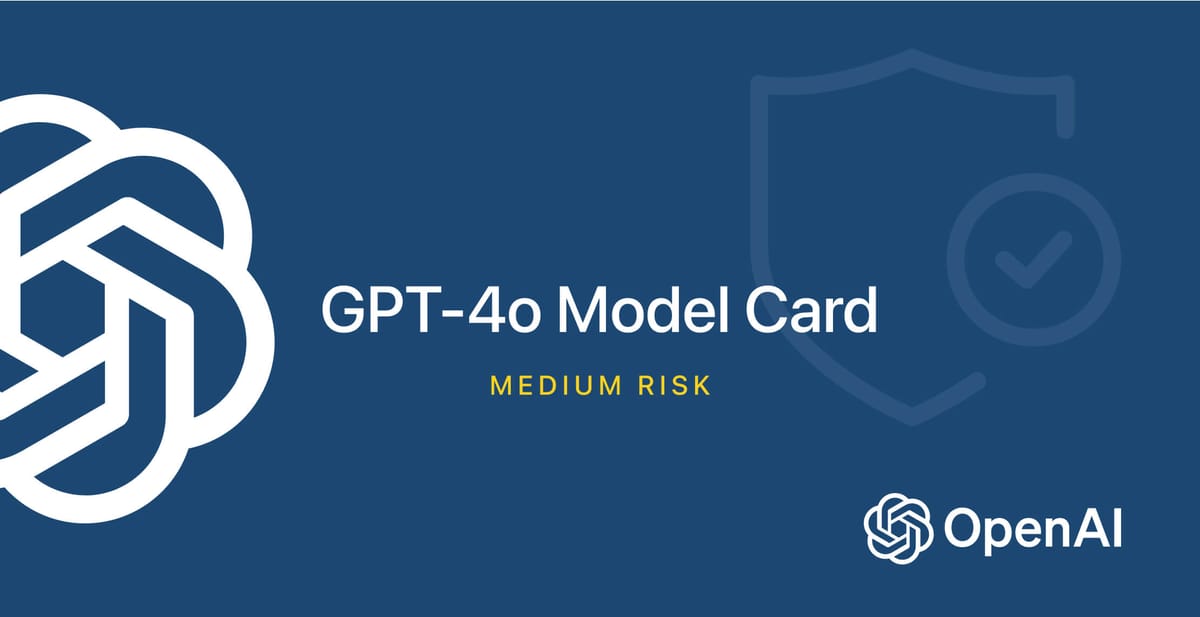
OpenAI has released a very detailed model card for GPT-4o focused on the rigorous safety measures that were undertaken before the model’s public release in May. While the company has still been cautious in the rollout of GPT-4o’s multimodal capabilities post launch, the release of this model card provides a much needed deep dive into how OpenAI has addressed any associated risks with its model.
Central to the model card is OpenAI’s Preparedness Framework, a systematic approach to evaluating and mitigating risks associated with their AI systems. This framework is designed to identify potential hazards in areas such as cybersecurity, biological threats, persuasion, and model autonomy. The release of the model card highlights the results of these evaluations and the steps taken to ensure that GPT-4o could be deployed safely.
This level of disclosure is particularly noteworthy given the AI industry's ongoing discussions about responsible development and deployment. Since last November, the ChatGPT developer has faced allegations and criticism from both former employees and external observers for not prioritizing AI safety. However, this model card tells a completely different story—detailing the company's thoughtful and methodical approach and the extensive mitigation strategies they have implemented.
The contrast between prevailing public opinion and the depth of safety considerations revealed in this report is striking. OpenAI's decision to publish such a detailed account of their safety measures appears to be a direct response to these criticisms, demonstrating a commitment to transparency that goes beyond industry norms. Here's what I mean:
- Detailed Risk Assessments: The model card provides in-depth analyses of potential risks across multiple domains, from unauthorized voice generation to biological threats.
- Mitigation Strategies: For each identified risk, OpenAI outlines specific, technical measures implemented to address concerns.
- External Validation: The report details the involvement of over 100 external red teamers and independent labs in the evaluation process, adding credibility to their safety claims.
- Acknowledgment of Ongoing Challenges: OpenAI openly discusses areas requiring further research, demonstrating a realistic and proactive approach to AI safety.
According to the framework, GPT-4o’s preparedness scores were rated low in three categories—cybersecurity, biological threats, and model autonomy. However, the model’s potential for persuasion, particularly in audio, was flagged as a borderline medium risk. This prompted additional safety measures before the model’s release, reflecting OpenAI’s commitment to preventing AI misuse.
One of the most significant risks identified in the model card is unauthorized voice generation, which could potentially be used for impersonation or fraud. OpenAI's approach to mitigating this risk is multifaceted:
- Restricted Voice Set: GPT-4o is limited to using only pre-approved voices created in collaboration with voice actors.
- Post-Training Conditioning: The model was trained to adhere to behavior that would reduce risk via post-training methods.
- Real-Time Voice Verification: A standalone output classifier was implemented to detect in real-time if the GPT-4o output is using a voice different from the approved list.
The effectiveness of these measures is impressive, with OpenAI reporting a 100% catch rate for meaningful deviations from the system voice based on their internal evaluations.
Another key risk area addressed in the model card is speaker identification, which presents potential privacy concerns. OpenAI's mitigation strategy here involved post-training GPT-4o to refuse compliance with requests to identify someone based on a voice in an audio input. Interestingly, the model still complies with requests to identify people associated with famous quotes, striking a balance between privacy protection against things like doxxing and maintaining useful functionality.
The model card also highlights OpenAI's efforts to prevent ungrounded inferences and sensitive trait attribution. This includes training GPT-4o to refuse requests for ungrounded inferences (such as determining a speaker's intelligence level from their voice) and to provide hedged answers for sensitive trait attributions (like identifying a speaker's accent).
In terms of content moderation, OpenAI has adapted its existing text-based moderation systems to work with audio conversations. This includes running their moderation classifier over text transcriptions of both audio input and output, blocking generation if potentially harmful language is detected.
The model card goes into considerable detail about the evaluation methodologies used, including the conversion of existing text-based evaluation datasets to audio formats using text-to-speech systems. This allowed OpenAI to leverage a wide range of existing evaluations while also developing new ones specific to audio capabilities.
Overall, I am encouraged by the depth and breadth of safety considerations that OpenAI has outlined in this model card. It challenges other AI model providers to be more forthcoming about their safety practices and could potentially reshape public and regulatory expectations for responsible AI development.