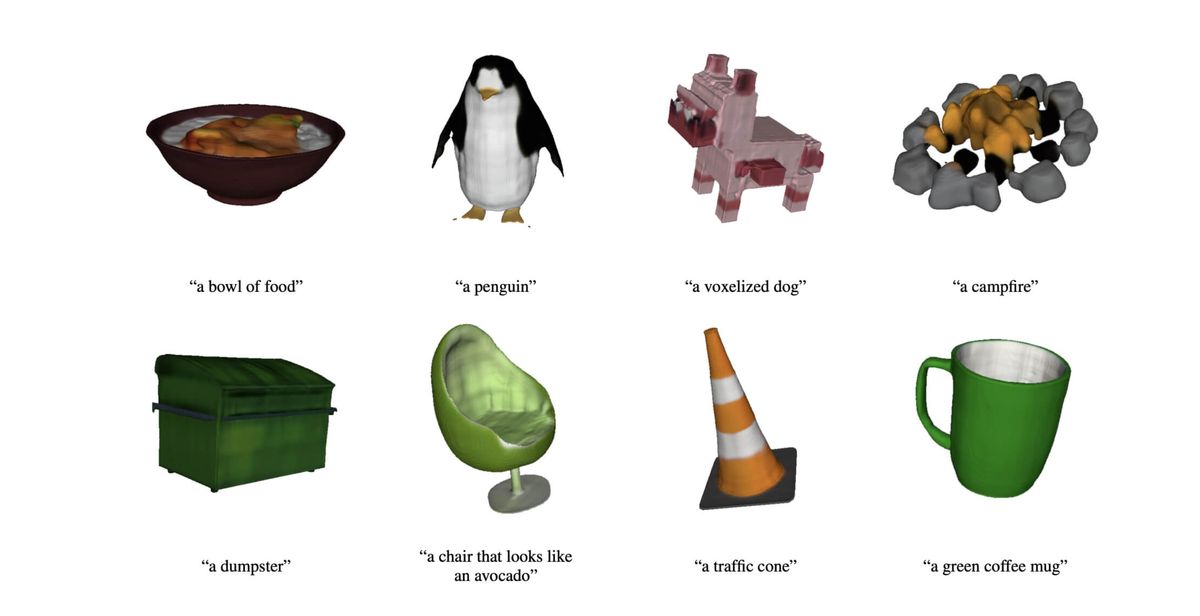
OpenAI has released their latest research showing significant progress in text-to-3D generation with Shap·E. This cutting-edge AI model is designed to generate 3D implicit functions from textual prompts, further advancing the field of 3D object generation.
The primary takeaway from the research paper is the introduction of a novel method for generating 3D models that addresses the limitations of their previous work, Point·E. Published in December 2022, Point·E was a pioneering system capable of creating 3D point clouds from complex prompts. However, it struggled with capturing fine-grained shapes and textures, which limited its practical applications.
In contrast, Shap·E is designed to generate the parameters of implicit functions directly, allowing for the rendering of both textured meshes and neural radiance fields (NeRFs). This key difference empowers Shap·E to produce more detailed and accurate 3D models compared to the point clouds generated by Point·E.
At the heart of Shap·E's success is the use of NeRFs, which are continuous volumetric scene representations. These fields have been revolutionary in the domains of augmented reality (AR) and virtual reality (VR), as they enable the generation of photorealistic renderings of 3D scenes. Basically, Shap·E combines NeRFs with diffusion models to efficiently generate detailed 3D models that capture the shape and texture of objects more accurately than its predecessor.
Shap·E is also much more performant. Each sample above took roughly 13 seconds to generate on a single NVIDIA V100 GPU (and does not require a separate text-to-image model). This compares to Point·E that produced 3D models in one to two minutes on the same hardware.
While the advantages of Shap·E are numerous, it's important to acknowledge the challenges that come with this method. The generation process can be computationally intensive, and the AI model may still struggle with certain complex objects. Despite these challenges, Shap·E represents a significant improvement over Point·E and shows great promise for future development.
Though the research is still in its early stages, the potential applications of Shap·E's technology are vast. As the technology continues to be improved, it could revolutionize industries such as gaming, animation, architecture, and engineering, among others.