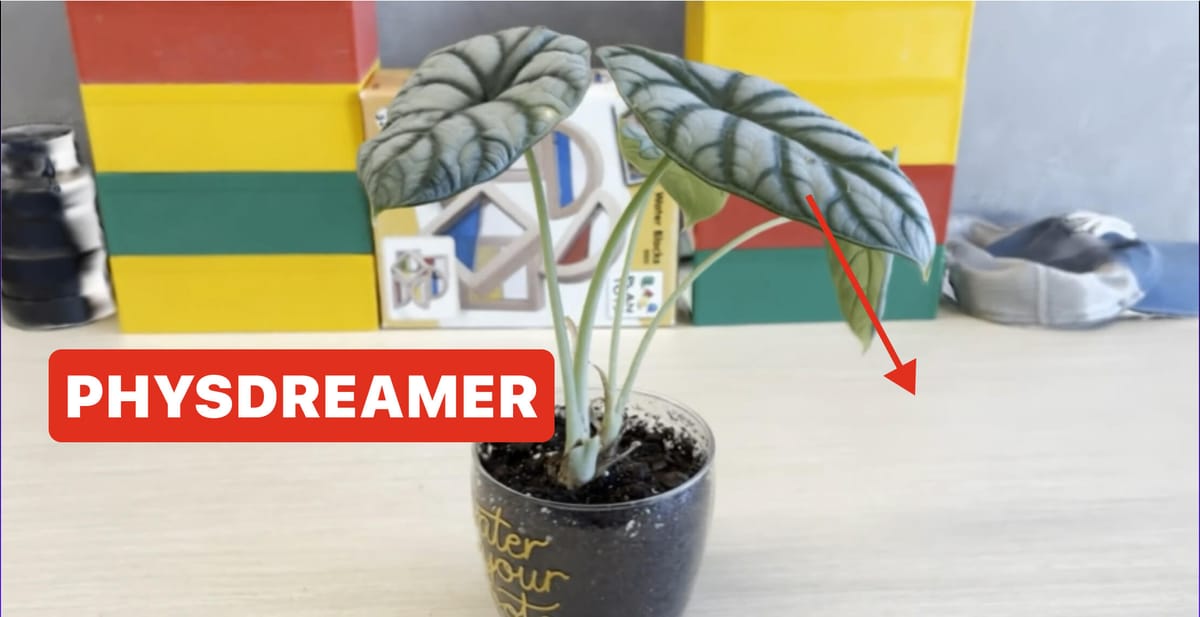
Simulating object interactions is crucial for generating realistic videos with AI or creating engaging virtual worlds. Producing action-based dynamics requires understanding the physical properties of objects, such as stiffness, and predicting 3D motion based on these characteristics. However, estimating real-world material properties is difficult due to a lack of ground truth data.
Researchers from MIT, Stanford, Columbia and Cornell have developed a novel technique called PhysDreamer to address this problem. PhysDreamer is a physics-based approach that imbues static 3D objects with interactive dynamics by distilling the motion priors learned by video generation AI models. This enables the synthesis of realistic object responses to novel interactions like external forces or agent manipulations.
The key idea behind PhysDreamer is to generate a plausible video of the object in motion and then optimize the material properties to match this synthesized motion. The researchers hypothesize that video generation models, trained on large amounts of video data, implicitly capture the relationship between object appearance and dynamics.
PhysDreamer represents 3D objects using 3D Gaussians, models the physical material properties with a neural field, and simulates the dynamics using a differentiable physics engine called the Material Point Method (MPM). By optimizing the material properties and initial velocity through differentiable simulation and rendering, PhysDreamer matches the object's motion to the AI-generated reference video.
The researchers demonstrated their approach on a diverse set of elastic objects including flowers, plants, a beanie hat, and a telephone cord. To validate the realism of the interactions generated by PhysDreamer, the researchers conducted a user study. Participants found the PhysDreamer-simulated motion to be significantly more realistic than existing methods in 53.7% of comparisons, even preferring it to real captured video. However, the authors note that PhysDreamer tends to produce smoother, lower-frequency motion compared to real-world dynamics.
Looking ahead, the researchers believe PhysDreamer is a significant step towards creating more immersive and lifelike virtual worlds. Future directions include improving computational efficiency and extending the approach to a wider range of materials and dynamic phenomena.