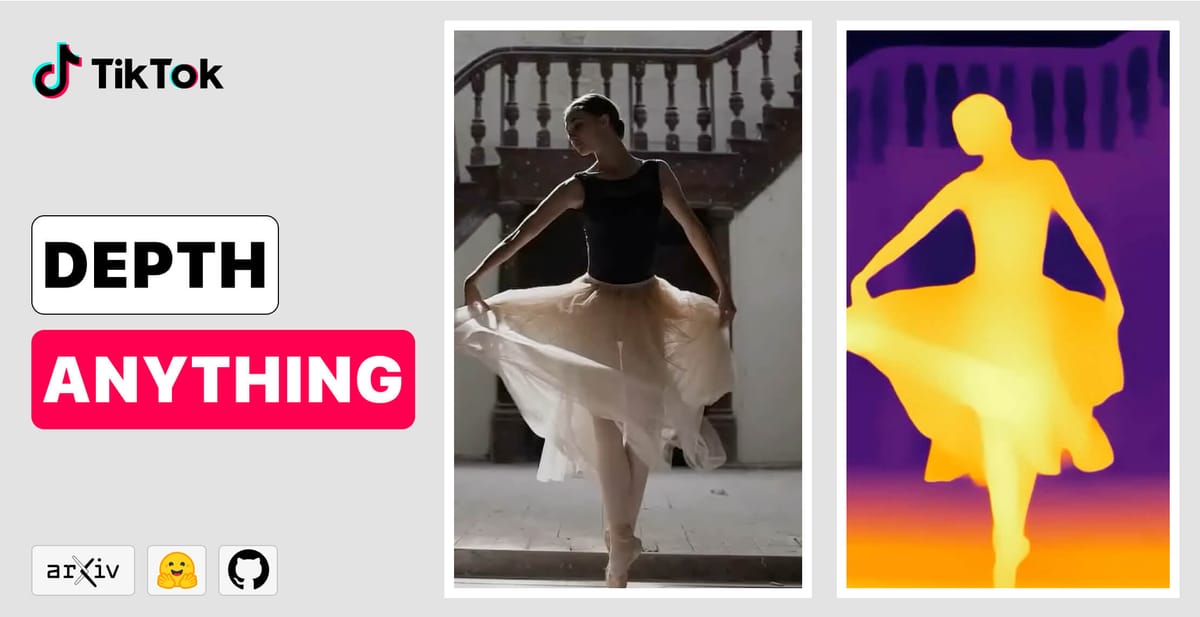
Researchers from TikTok, The University of Hong Kong and Zhejiang Lab have shared new research that set impressive benchmarks for Monocular Depth Estimation (MDE) across a variety of datasets and metrics. Titled Depth Anything, it marks a notable advancement in AI-driven depth perception, particularly in understanding and interpreting the depth of objects from a single image.
At the heart of 'Depth Anything' lies its training on a colossal dataset: 1.5 million labeled images, supplemented by over 62 million unlabeled images. This extensive training set is key to the model's proficiency. Unlike traditional MDE models that primarily rely on smaller, labeled datasets, 'Depth Anything' leverages the sheer volume of unlabeled data. This approach significantly expands the data coverage, thereby reducing generalization errors – a common challenge in AI models.
A New Benchmark in Depth Estimation
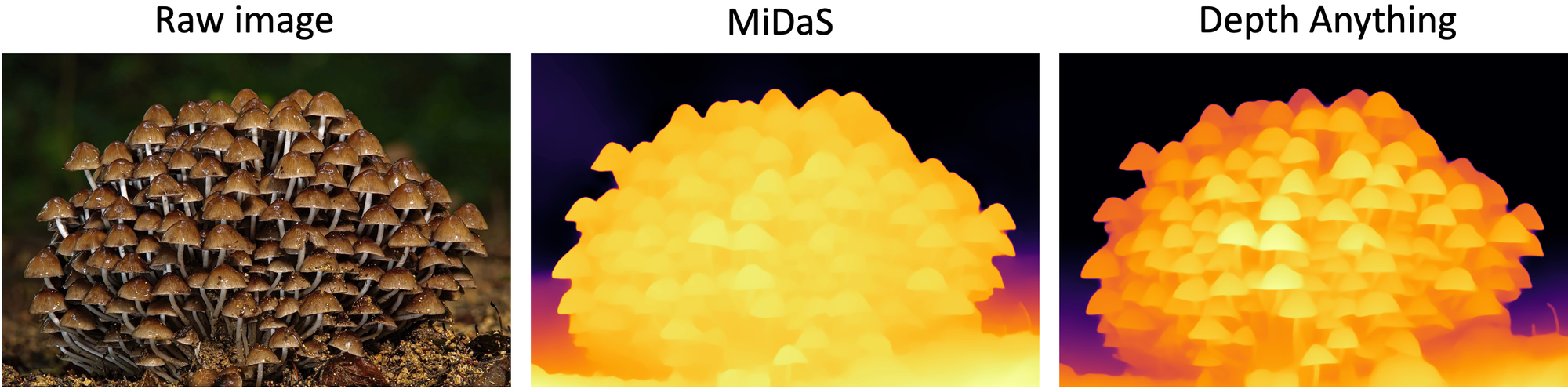
What sets 'Depth Anything' apart? For starters, it achieves zero-shot relative depth estimation with superior performance compared to MiDaS v3.1 (BEiTL-512). This means it can estimate depth in images it has never seen before without any additional training. Furthermore, it surpasses ZoeDepth in zero-shot metric depth estimation, demonstrating its ability to gauge the actual distance of objects from the camera.
When fine-tuned on metric depth data from NYUv2 and KITTI, Depth Anything models achieved new state-of-the-art results on those widely used benchmarks as well. This combination of strong generalization and fine-tuned performance cements Depth Anything as a new foundation for monocular depth estimation research going forward.
Beyond Depth: Enhancing ControlNet
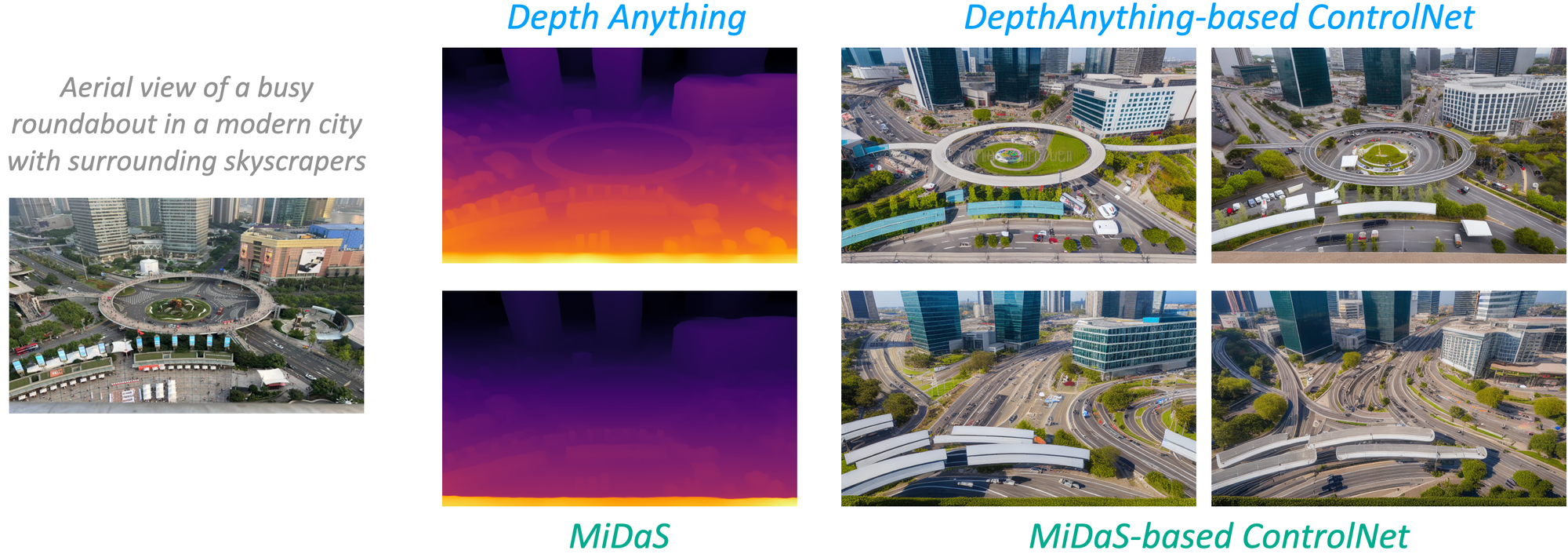
The impact of 'Depth Anything' goes beyond just depth estimation. The researchers re-trained a depth-conditioned ControlNet based on this model, achieving better results than the previous version based on MiDaS. This enhancement indicates a broader applicability of the depth model in areas like autonomous driving, where understanding the surrounding environment is crucial.
Visualizing Depth in Dynamic Scenarios
While 'Depth Anything' is primarily an image-based depth estimation method, the researchers have showcased its capabilities through video demonstrations. These visualizations are instrumental in highlighting the model's superiority in dynamic, real-world scenarios. For more detailed, image-level visualizations, the research paper is an excellent resource.
A Model of Simplicity and Power
Interestingly, the innovation of 'Depth Anything' doesn't stem from novel technical modules. Instead, the researchers focused on creating a simple yet potent foundation model that can handle images under any circumstances. This practical approach is commendable, as it prioritizes versatility and robustness over complexity.
The strategies employed are straightforward yet effective. Firstly, the use of data augmentation tools presents a more challenging optimization target, compelling the model to seek extra visual knowledge. Secondly, an auxiliary supervision from pre-trained encoders is developed, allowing the model to inherit rich semantic priors.
With impressive zero-shot abilities and leading fine-tuned performance on datasets like KITTI and NYUv2, Depth Anything represents a new high water mark in monocular depth estimation that highlights the power of scaled-up diverse training data.
If you are interested in learning more about Depth Aything, here is a link to the: