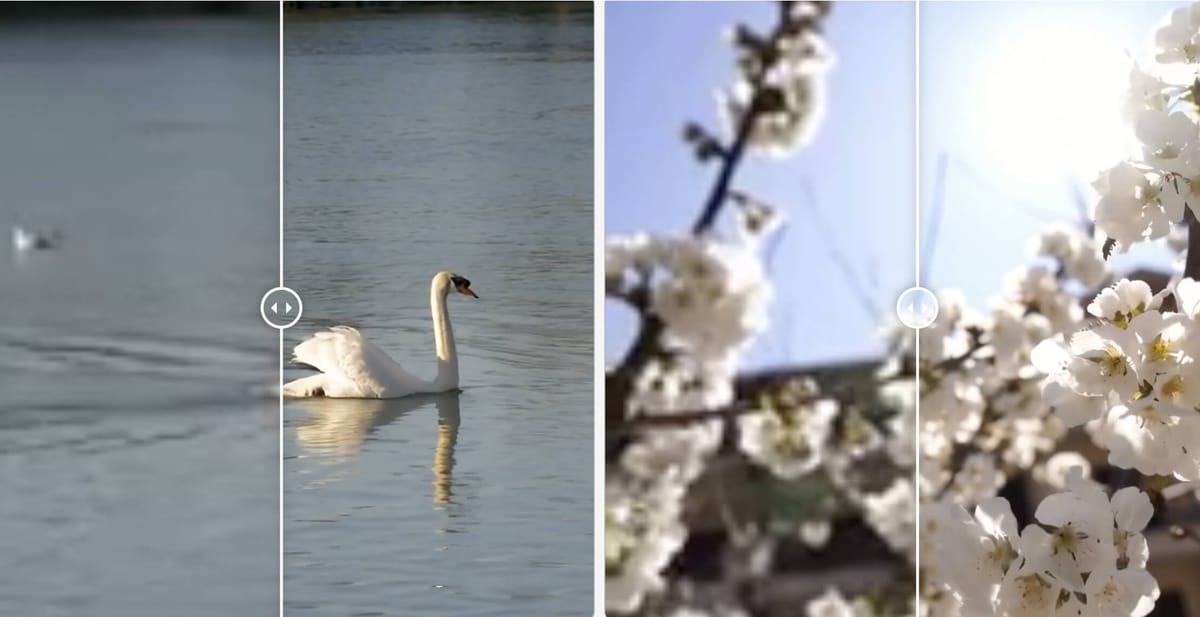
Adobe researchers have introduced VideoGigaGAN, a generative AI model capable of upsampling videos by up to 8× while preserving fine details and ensuring temporal consistency across frames. Building upon the success of the large-scale image upsampler GigaGAN, VideoGigaGAN aims to bring the same level of detail-rich upsampling to videos without compromising temporal stability.
One of the key challenges in video super-resolution (VSR) is maintaining consistency across output frames while simultaneously generating high-frequency details. Previous VSR approaches have demonstrated impressive temporal consistency but often produce blurrier results compared to their image counterparts due to limited generative capabilities. VideoGigaGAN tackles this issue head-on by adapting the powerful GigaGAN architecture for video upsampling.
The researchers discovered that simply inflating GigaGAN to a video model by adding temporal modules resulted in severe temporal flickering. To address this, they identified several critical issues and proposed techniques to significantly improve the temporal consistency of upsampled videos. These techniques include:
- Inflating the GigaGAN with temporal attention layers in the decoder blocks to enforce temporal consistency.
- Incorporating features from a flow-guided propagation module to enhance consistency across frames.
- Using anti-aliasing blocks in the downsampling layers of the encoder to suppress aliasing artifacts.
- Directly shuttling high-frequency features via skip connections to the decoder layers to compensate for detail loss during the BlurPool process.
Method aside, what we really care about is the result. And their study shows that VideoGigaGAN generates temporally consistent videos with significantly more fine-grained appearance details compared to state-of-the-art VSR methods. The researchers validated VideoGigaGAN's effectiveness by comparing it with leading VSR models on public datasets and showcasing impressive video results with 8× super-resolution.
The ablation study revealed that the strong hallucination capability of image GigaGAN led to temporally flickering artifacts, particularly aliasing caused by the artifacted low-resolution input. By addressing these issues through the proposed techniques, VideoGigaGAN achieves a remarkable balance between detail-rich upsampling and temporal consistency.
VideoGigaGAN represents a significant advancement in video super-resolution, pushing the boundaries of what is possible with generative AI. Its ability to upsample videos by up to 8× while maintaining fine details and temporal stability opens up new possibilities for enhancing low-resolution video content. As the demand for high-quality video continues to grow across various industries, VideoGigaGAN has the potential to revolutionize how we process and consume video media.
However, a lot of research still remains to be done to overcome certain limitations. For example, VideoGigaGAN faces challenges with extremely long videos and small objects. Future work can focus on improving optical flow estimation and handling small details like text and characters.