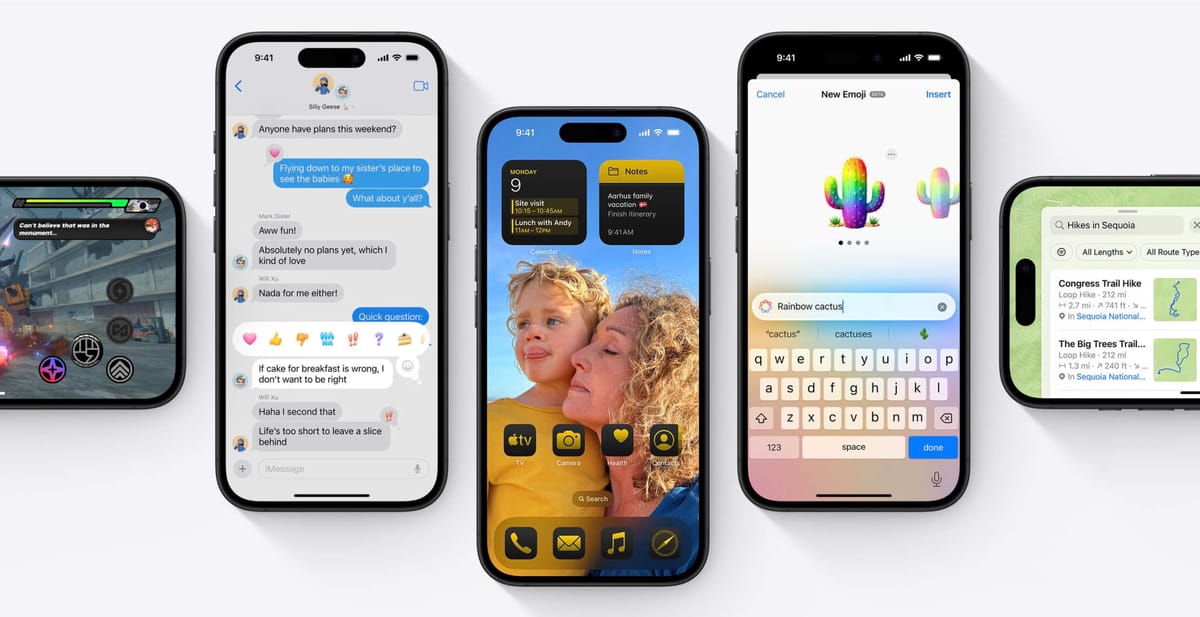
Researchers at Apple have published a paper detailing their work on Ferret-UI, a new multimodal large language model (MLLM) that excels in understanding UI elements, their functions, and potential user interactions, surpassing even GPT-4V in some basic UI tasks.
Ferret-UI is designed to perform three core tasks related to mobile screens: referring, grounding, and reasoning. These capabilities enable it to precisely understand what’s on a screen and execute actions based on that understanding.
- Referring tasks: Ferret-UI is capable of identifying and classifying UI elements like widgets, icons, and text using various input formats (e.g., bounding boxes, scribbles, or points). This allows the model to understand what specific UI elements are and how they function within the context of the overall screen. For example, Ferret-UI can distinguish between different icons, classify text elements, and even interpret widget functionality.
- Grounding tasks: Grounding refers to the model’s ability to pinpoint the exact location of elements on a screen and use that information to respond to instructions. For instance, the model can find a specific widget, identify text, or list all the widgets present on a mobile UI. This detailed spatial understanding is crucial for more advanced interactions, like when you ask Siri to “find the Wi-Fi settings,” and Siri must understand where to navigate within your phone.
- Reasoning tasks: Beyond simply identifying UI elements, Ferret-UI is equipped with reasoning capabilities that enable it to make sense of the overall function of a screen. It can describe what’s on the screen in detail, engage in goal-oriented conversations, and deduce the purpose of a UI layout. This kind of advanced understanding sets Ferret-UI apart from previous models that could only handle simple recognition tasks.
A key innovation in Ferret-UI is its any-resolution (anyres) capability, which allows the model to adapt seamlessly to different screen aspect ratios while maintaining high precision in recognizing and interacting with UI elements. The model divides screens into sub-images, allowing it to capture both the overall context and fine details of UI elements.
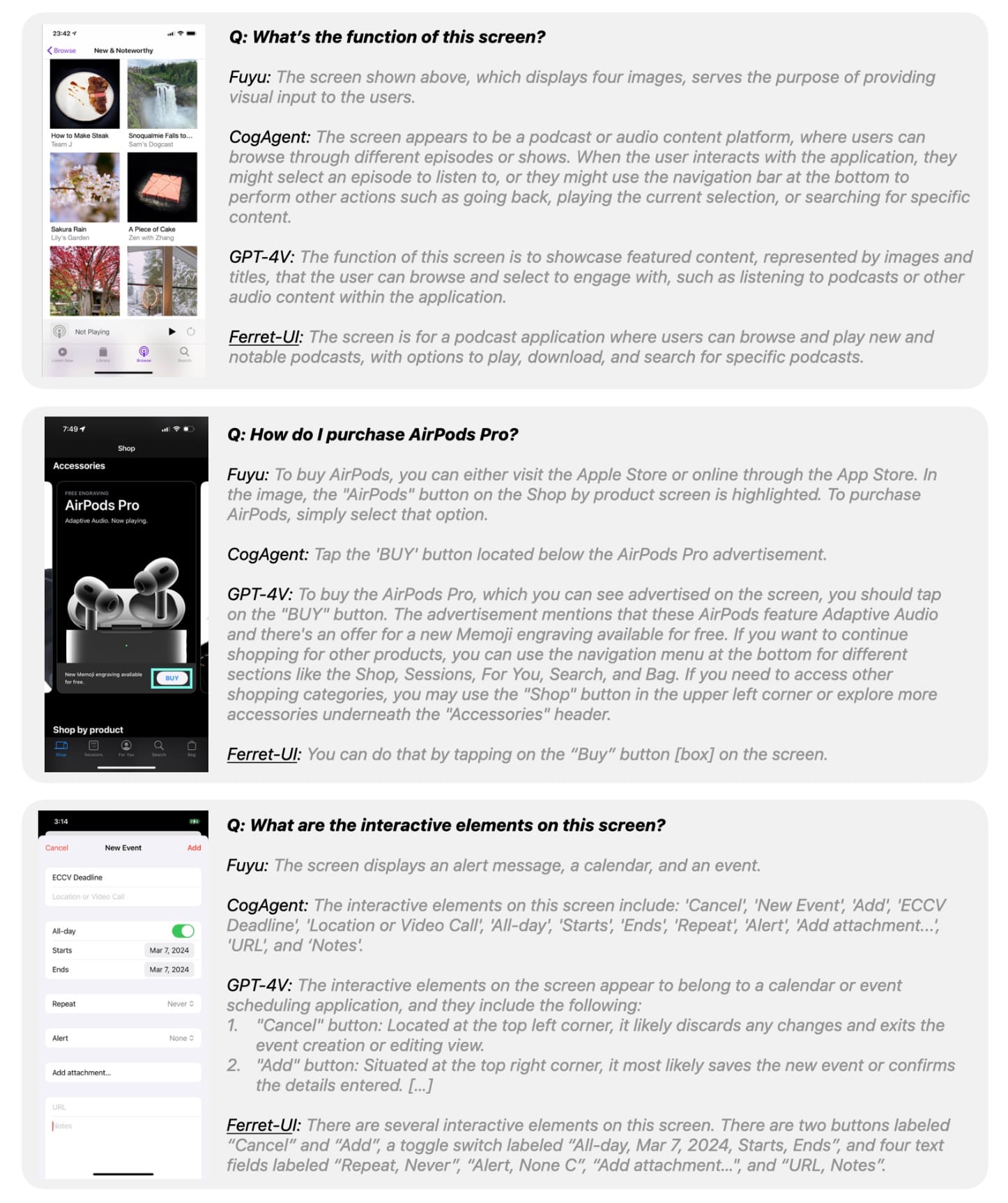
One other interesting aspect of this research is how Apple used GPT-3.5 to generate a diverse and rich training dataset. This use of synthetic data is becoming increasingly popular in AI research because it allows models to be trained on a much larger and more diverse set of examples than real-world data alone would provide. For Ferret-UI, this synthetic data helped hone its ability to perform complex mobile UI tasks with a high degree of precision.
While Ferret-UI is simply a research project, it's exciting to imagine how this technology could shape future mobile experiences. We might one day see a significantly smarter Siri with agentic capabilities, able to navigate and use your iPhone like a human would. It could understand complex voice commands referencing on-screen elements, automate multi-step tasks across different apps, or provide more nuanced assistance based on what's currently on your screen.
Another potential use case for Ferret-UI’s technology is in mobile accessibility. This technology could be used to help provide more accurate and context-aware descriptions of app interfaces. It could assist developers in automated UI testing, identifying usability issues that might be missed by conventional methods. The system could even power more intelligent app suggestions, recommending actions based on a deeper understanding of user intent and context.
However, it's worth noting that Ferret-UI, despite its impressive capabilities, still has limitations. It relies heavily on pre-defined UI element detection, which can miss nuances like overall design aesthetics. Additionally, while it excels at basic UI tasks, more complex reasoning still poses challenges.
Apple's research with Ferret-UI underscores their strategy of developing specialized, efficient AI models designed to run directly on devices. This aligns with their emphasis on user privacy and device-level processing. While the Silicon Valley giant has been relatively quiet in the new era of generative AI, the company has been publishing extensive research in this space. Earlier this year, they even released an open-source model family on Hugging Face.