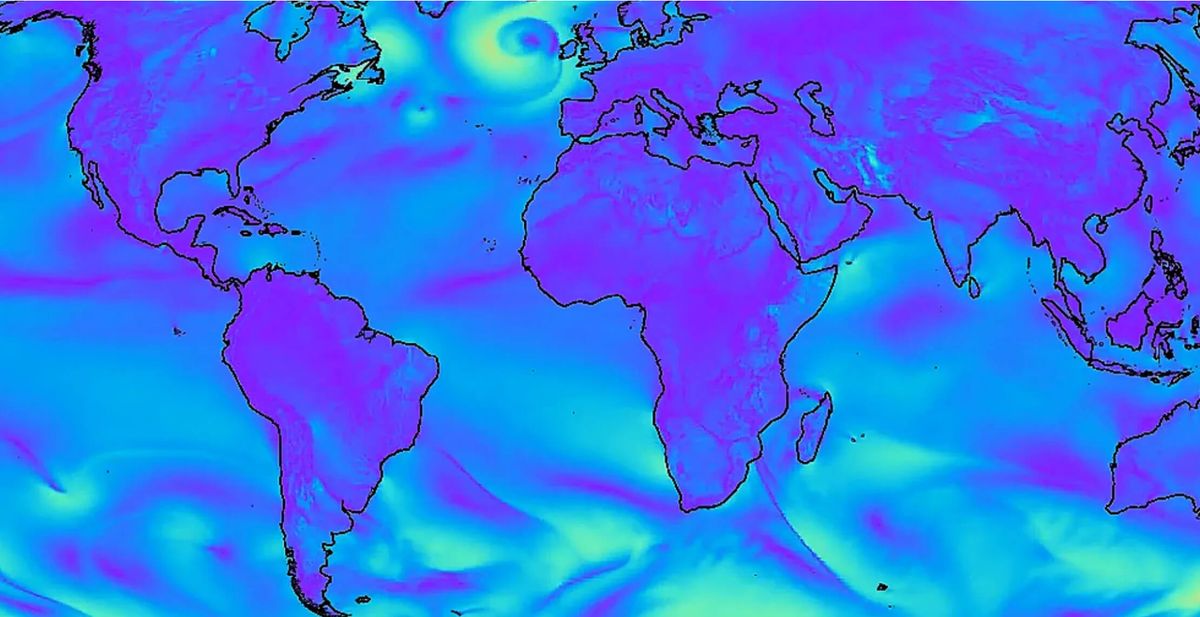
In a breakthrough for AI in weather prediction, researchers at Google DeepMind have unveiled GraphCast—a revolutionary machine learning model that can generate highly accurate 10-day global weather forecasts in under one minute.
In a paper published in Science, GraphCast represents a leap forward in forecasting skill compared to traditional numerical weather prediction (NWP) methods. The current gold standard operational model, the European Centre for Medium-Range Weather Forecasts' High Resolution Forecast (HRES), takes around an hour to run on a supercomputer. In comprehensive tests, GraphCast significantly outperformed HRES on over 90% of the evaluated weather variables and levels.
GraphCast represents a significant advancement in weather forecasting technology, employing a sophisticated combination of machine learning and Graph Neural Networks. At its core, this AI model is trained on extensive historical weather data, spanning decades. This extensive dataset enables GraphCast to discern the intricate cause-and-effect relationships governing Earth's weather patterns.
The model operates at a remarkably high resolution of 0.25 degrees longitude/latitude, effectively covering over a million grid points across the Earth's surface. At each of these points, GraphCast predicts a comprehensive set of weather variables, including temperature, wind speed, direction, and mean sea-level pressure, along with six atmospheric variables at different altitude levels.
Unlike traditional methods that rely on complex physical equations and substantial computational resources, GraphCast uses data-driven predictions, resulting in not only enhanced accuracy but also remarkable efficiency. Its ability to produce a detailed 10-day forecast in less than a minute on a single Google TPU v4 machine stands in stark contrast to the hours-long computations required by conventional systems. This efficiency, combined with its unprecedented accuracy, makes GraphCast a groundbreaking tool in the field of meteorological science.
GraphCast works by taking a snapshot of the current weather conditions across the globe, as well as the weather from 6 hours earlier. It represents this data as a grid covering the entire planet. GraphCast then uses its deep learning algorithms to analyze patterns in this gridded data. It looks at how weather in one location affects surrounding regions, both nearby and far away. The model processes these complex interconnections between all parts of the globe.
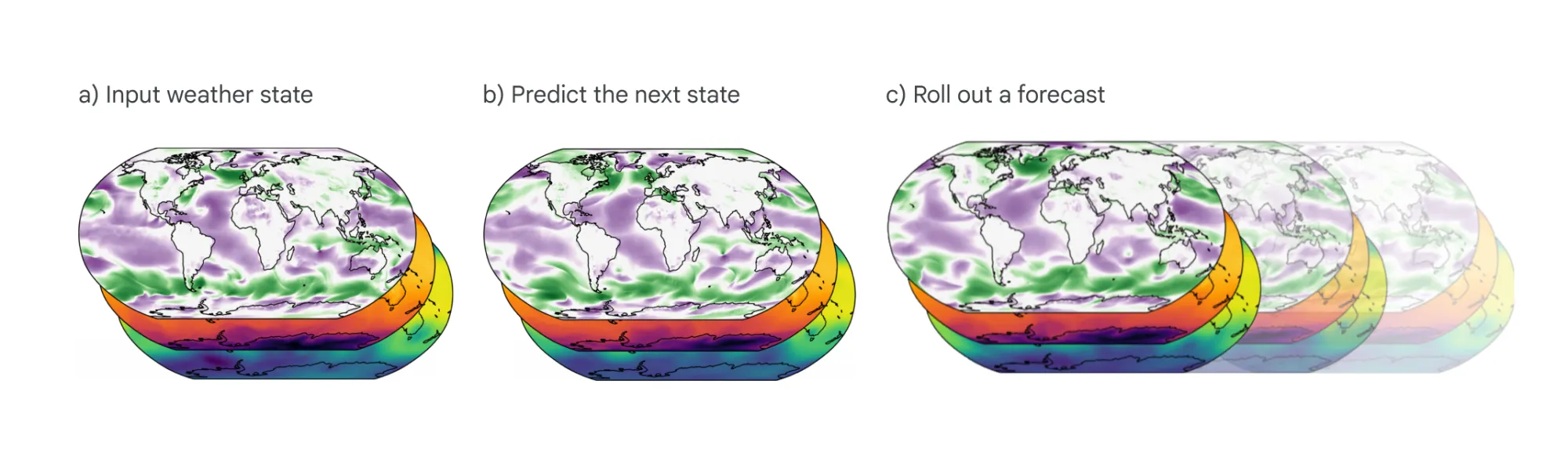
Finally, GraphCast makes a prediction of what the weather will look like 6 hours into the future, based on the patterns it has learned. To generate forecasts longer than 6 hours, GraphCast feeds its own predictions back into its algorithms as inputs. This allows it to roll forward and predict full weather states over 10 days, by recursively predicting each 6-hour step into the future.
Beyond raw accuracy, GraphCast also demonstrated skill at predicting extreme weather events like tropical cyclones, atmospheric rivers, and temperature extremes. This could provide earlier warnings to help communities prepare for dangerous storms or heat waves.
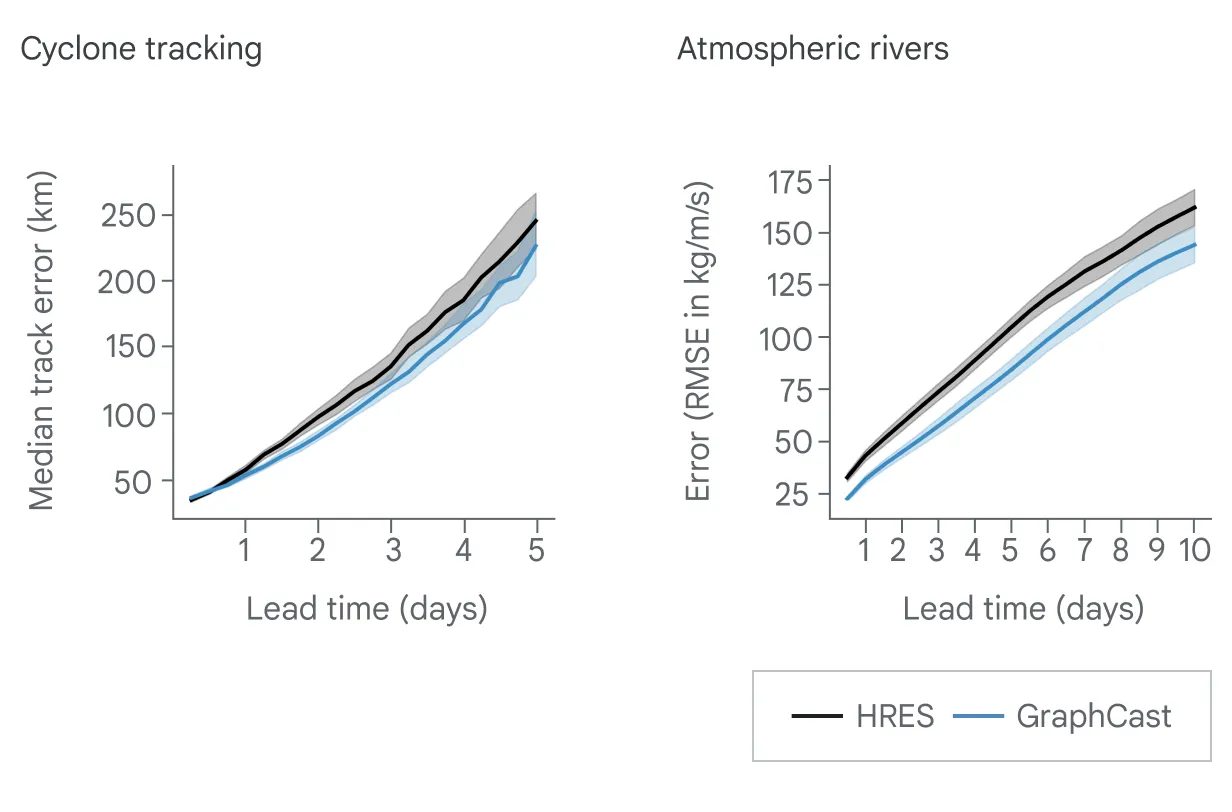
GraphCast sets a new standard for machine learning in a complex scientific domain like weather forecasting. While traditional NWP methods require extensive expert design of numerical algorithms, GraphCast shows AI models trained end-to-end on data can effectively model Earth's chaotic weather dynamics.
The researchers emphasize GraphCast should complement, not replace traditional methods. Combining AI techniques with the decades of meteorological insights baked into NWP could drive forecasting to new levels. GraphCast also currently lacks robust uncertainty estimates that ensemble NWP provides.
GraphCast is already being used by weather agencies. To see it in action, hop over to the European Centre for Medium-Range Weather Forecasts website which is running a live experiment with the model.
By open sourcing GraphCast, Google DeepMind hopes to empower researchers worldwide to build on AI's potential for accelerating weather science. More accurate and efficient forecasting promises immense social value, from powering renewable energy to protecting lives during disasters. Unlocking this with AI represents an exciting new chapter in harnessing technology to serve humanity.