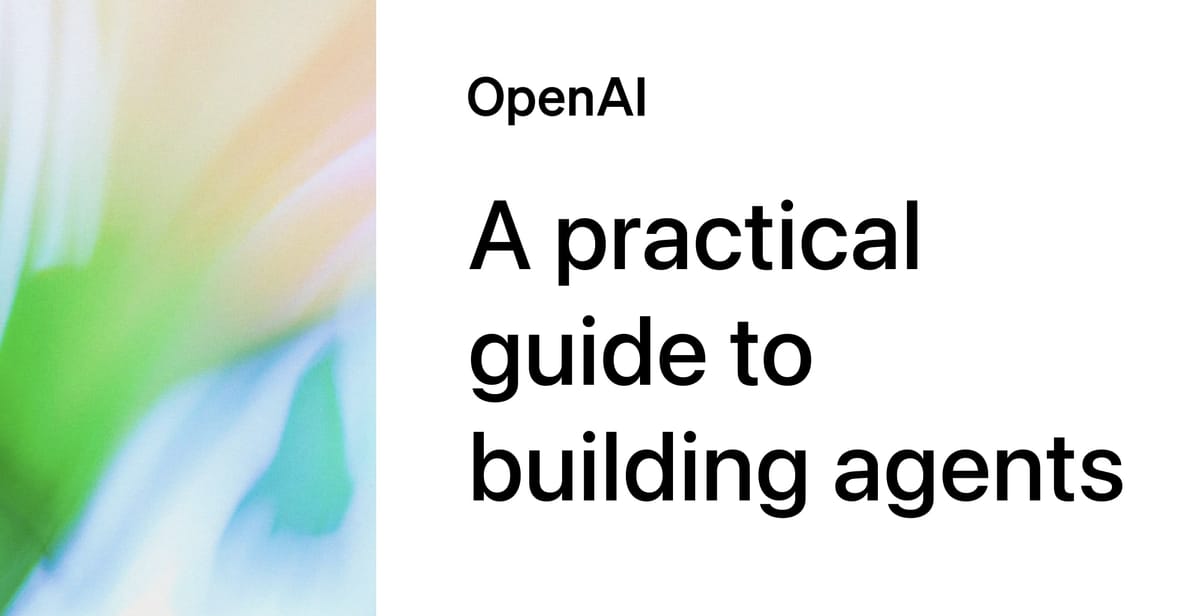
OpenAI has quietly published a substantial new resource for developers looking to build AI Agents. The 32-page document, aptly named, "Practical Guide to Building Agents", lays out a methodical approach to creating systems that can independently accomplish tasks with minimal human intervention.
Key Points
- Framework for choosing workflows that benefit most from agent autonomy
- Three foundational pillars: models, tools and instructions
- Orchestration patterns spanning single‑agent loops to multi‑agent ecosystems
- Layered guardrails and human‑in‑the‑loop safeguards for safety and reliability
As large language models become more adept at reasoning, multimodal understanding and tool use, AI agents have emerged as a critical interface through which we will access the technology. This guide distills lessons learned from real‑world deployments of AI agents into actionable best practices. But first, what is an AI agent?
Agents are systems that independently accomplish tasks on your behalf.
The guide describes agents as systems that leverage language models to control workflow execution, access tools to gather context and take actions, and operate within defined guardrails.
Not every workflow demands an agent approach. The guide suggests focusing on use cases where traditional automation falls short: complex decision-making scenarios like refund approvals, systems with unwieldy rule sets like vendor security reviews, or processes heavily dependent on unstructured data such as insurance claims processing.
"While conventional software enables users to streamline and automate workflows, agents are able to perform the same workflows on the users' behalf with a high degree of independence," OpenAI explains in the document.
The technical architecture section breaks down agent design into three core components:
- Choosing the right language models to balance accuracy, latency and cost
- Defining reusable, well‑documented tools for data retrieval and action; and
- Crafting clear, unambiguous instructions that break tasks into discrete steps and anticipate edge cases
OpenAI recommends starting with the most capable models to establish performance baselines before potentially scaling down to more cost-effective options for specific tasks.
OpenAI also outlines orchestration patterns to scale agent architectures. Teams are encouraged to begin with single‑agent systems—where an agent loops through tool calls until exit conditions are met—and evolve to multi‑agent designs only when complexity demands it. The guide details both manager patterns, in which a central orchestrator delegates to specialized agents, and decentralized patterns, where agents hand off control directly to other agents as needed.
Security receives significant attention with a section on implementing guardrails as layered defense mechanisms. These range from relevance classifiers that keep agents on-topic to tool safeguards that assess risk before actions are executed. The guide emphasizes that human intervention remains a critical safeguard, especially for high-risk actions or when agents repeatedly fail to accomplish tasks.
For developers exploring AI agents, the guide provides a practical, code-first approach. Rather than focusing on theoretical agent architectures, OpenAI provides concrete implementation examples using its Agents SDK, demonstrating how to build both single and multi-agent systems with relatively few lines of code.