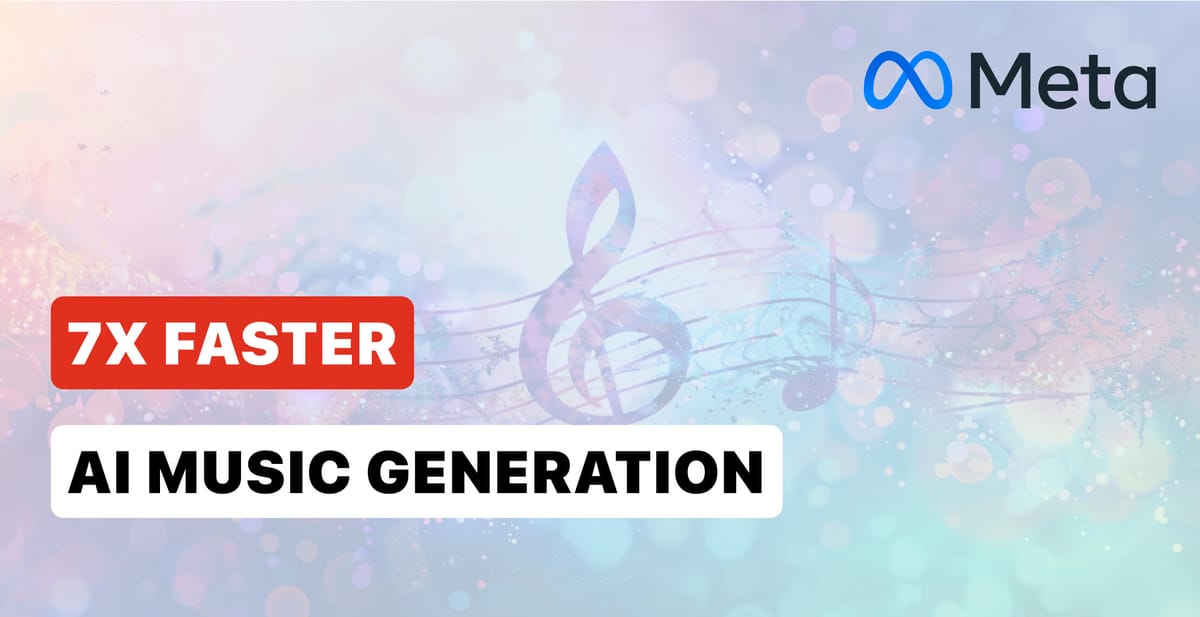
Researchers at Meta have open-sourced MAGNeT (Masked Audio Generation using Non-autoregressive Transformers), a new AI model capable of generating studio-grade text-to-music and text-to-sound results – at speeds up to 7 times faster than current state-of-the-art models.
Meta says MAGNeT was trained on 16K hours of licensed music. Specifically, used an internal dataset of 10K high-quality music tracks in addition to ShutterStock and Pond5 music data.
Unlike leading model that rely either on slower autoregressive decoding that sequentially generates audio signals, or on diffusion-based architectures that require lengthier sampling procedures, MAGNeT uses parallel masked predictive coding. This allows the model to generate 30-second musical compositions and soundscapes in a fraction of a second, with quality rivaling conditional language models and diffusion techniques.
This impressive speed stem from its masked generative sequence modeling directly on raw audio waveform tokens. The model is composed of a single transformer that predicts spans of masked input conditioned on visible context in a series of non-autoregressive decoding iterations. A novel rescoring component then refines audio fidelity by leveraging an external pre-trained model to rescore and rank predictions, which are then utilized in subsequent decoding steps.
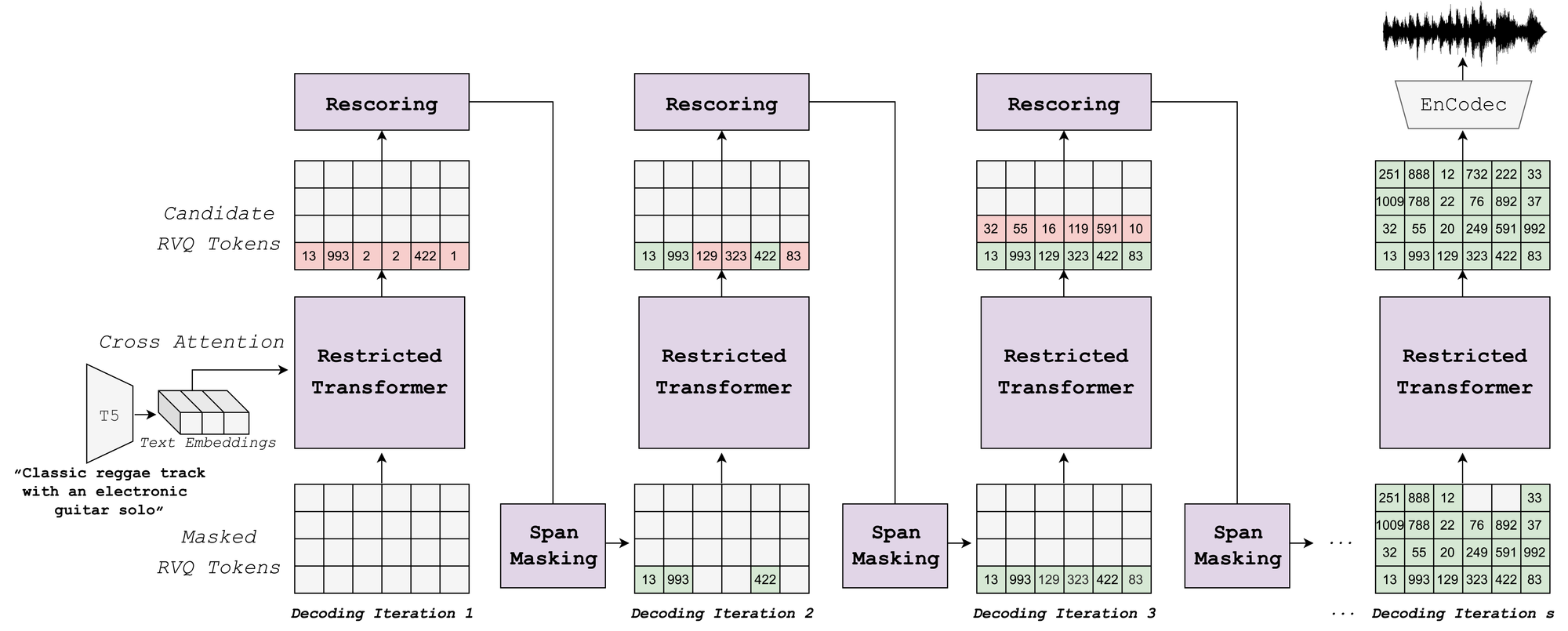
The researchers also introduced a hybrid MAGNeT version that combines the strengths of both autoregressive and non-autoregressive models that generates initial seconds of audio sequentially before switching to high-speed parallel generation - capturing the benefits of both approaches.
Evaluations found MAGNeT achieved parity with or marginally trailed baselines on metrics like Frechet Audio Distance while exceeding their speed by up to 700%. For applications like interactive music creation demanding real-time sound synthesis, MAGNeT promises a transformative advance.
MAGNeT's development sheds light on the trade-offs between autoregressive and non-autoregressive modeling, particularly in terms of latency, throughput, and generation quality. By offering a detailed analysis of these aspects, the researchers behind MAGNeT provide valuable insights into the potential directions for future research in audio generation technology.
Meta has open-sourced MAGNeT as a part of AudioCraft, their single-stop code base for all generative audio solutions.