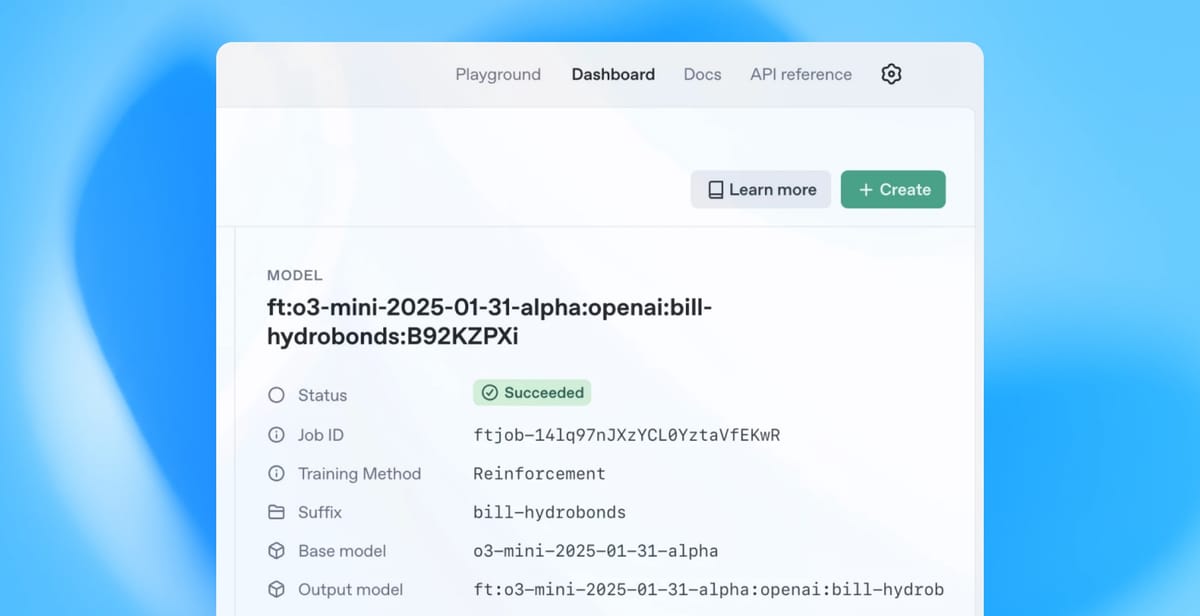
OpenAI has announced two major fine-tuning options for API customers: reinforcement fine-tuning (RFT) on o4-mini
and supervised fine-tuning (SFT) for GPT-4.1 nano
. RFT, which uses chain-of-thought reasoning and a user-provided grader to refine outputs, is available starting today to verified organizations on the o4-mini model. In parallel, all paid API tiers (Tier 1 and above) can now apply supervised fine-tuning to GPT-4.1 nano—the company’s fastest and most cost-effective model to date.
Key Points
- RFT on o4-mini: Reinforcement fine-tuning, previewed in December, is now generally available to verified organizations using the o4-mini reasoning model.
- Chain-of-thought upgrades: RFT leverages task-specific grading and chain-of-thought to push models further in complex domains.
- GPT-4.1 nano SFT: Supervised fine-tuning support lands on the fastest, cheapest GPT-4.1 nano, opening customization to all paid tiers.
RFT was first previewed in December as part of OpenAI’s alpha research program, inviting select partners to test its chains of thought optimization. Under the hood, developers supply both a dataset and a task-specific grading function; the model then iteratively hones its reasoning to maximize the grader’s reward signal. This method marks the first time OpenAI has enabled custom reasoning training on its o-series models.
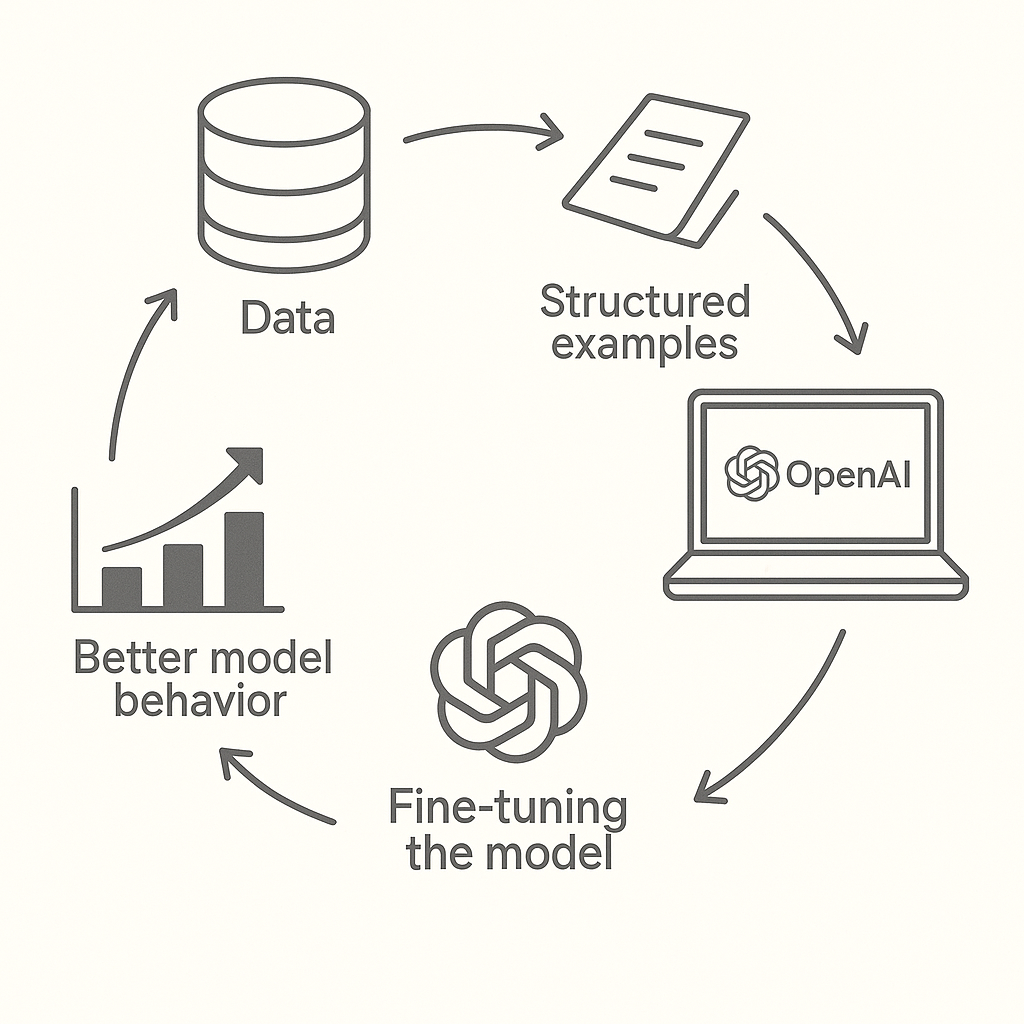
Several startups have already leveraged RFT in closed previews. Thomson Reuters used it to sharpen legal‐document analysis, while Ambience fine-tuned agentic assistants for customer support. ChipStack, Runloop, Milo, Harvey, Accordance, and SafetyKit also feature in OpenAI’s use-case guide, highlighting RFT’s versatility across industries.
Traditional supervised fine-tuning teaches a model to mimic example outputs; by contrast, RFT teaches a model how to think through problems, resulting in more robust performance on complex tasks. Early benchmarks suggest RFT-tuned models achieve higher data efficiency—requiring fewer examples to reach parity with supervised methods—and exhibit better error correction when faced with novel prompts.
Alongside RFT, OpenAI is now supporting classic supervised fine-tuning for GPT-4.1 nano, its smallest and swiftest model, across all paid API tiers. GPT-4.1 nano boasts a 1 million-token context window and delivers strong performance on benchmarks like MMLU (80.1%) and GPQA (50.3%), all while slashing latency and cost.
Developers can now upload their labeled datasets to train the nano model to their use cases, enabling custom classification, extraction, or domain-specific conversational agents—at unmatched speed and affordability. Microsoft’s Azure AI Foundry and GitHub integrations will soon support this fine-tuning path, further democratizing model customization.
These fine-tuning options represent OpenAI’s push toward hyper-customizable AI, catering to both deep-domain experts and cost-sensitive teams. RFT opens doors to specialized reasoning—ideal for legal, medical, or scientific applications—while supervised SFT on nano addresses straightforward classification or generation tasks without breaking the bank.
By offering RFT on o4-mini and SFT on nano, OpenAI now spans the spectrum of model customization: from lightweight, fast turns on the nano model to complex reasoning pipelines on o4-mini. This dual approach is poised to accelerate adoption of AI across sectors, from startups to large enterprises.
OpenAI’s roadmap includes expanding RFT access beyond verified organizations and introducing SFT support for GPT-4.1 mini and full-size GPT-4.1 soon. As the ecosystem matures, we can expect a diverse landscape of expert models, each fine-tuned for distinct tasks—shifting the paradigm from generic AI to tailored, domain-specific intelligence.