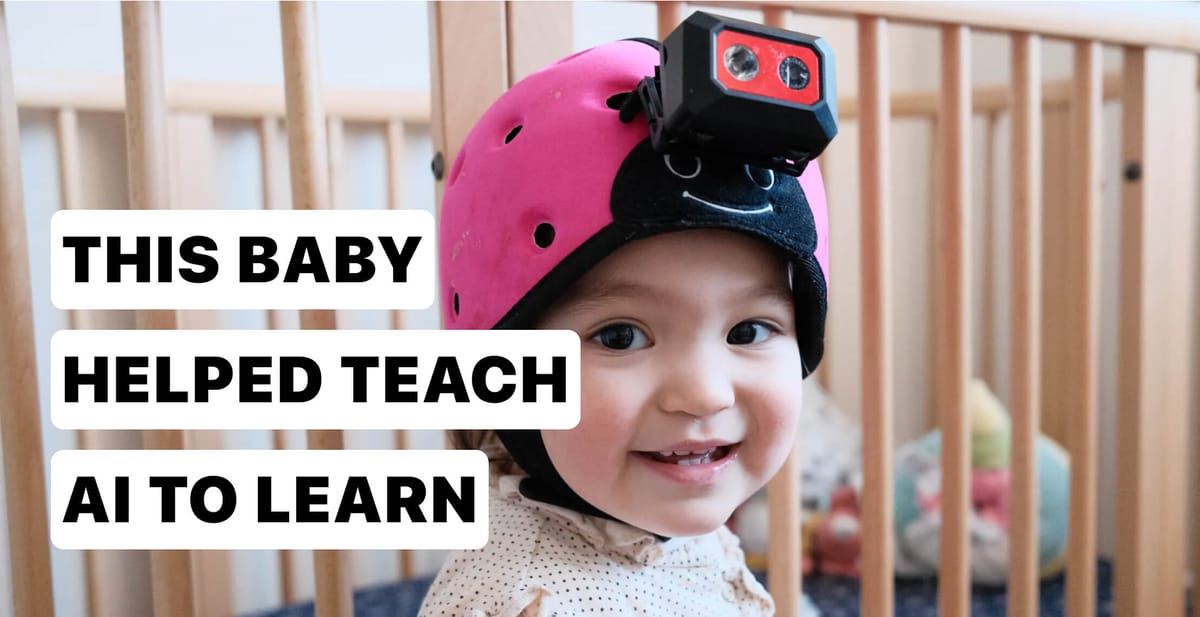
Researchers from New York University have developed an AI system that learned to associate words with objects, much like a human baby learns language. This surprisingly capable AI was trained on video footage from a camera worn by an infant as he played with toys and interacted with his parents. The findings were published this week in a paper in Science.
The system, dubbed the Child's View for Contrastive Learning (CVCL) model, was fed 37,500 utterances captured from 61 hours of first-person video recorded by a baby named Sam from ages 6 months to 2 years old. The footage gave an intimate view of Sam's life - playing with his shape sorter, grasping at blocks, even annoying his unimpressed cat.

By analyzing when certain objects and words co-occurred in the videos, the AI began to form connections. For example, when Sam's toy blocks were visible and his parent said "You want the blocks too," the system associated "blocks" with the wooden toys. Over time, these associations allowed CVCL to learn mappings between common words and visual concepts.
CVCL even grouped visually similar concepts into distinct sub-categories, like animal versus alphabet puzzles. This demonstrates an understanding of differences between related objects. The model also succeeded in generalizing words to new objects in testing. For instance, it could correctly label new images of balls after learning the word "ball" from training footage. However, its ability to identify less commonly seen objects, like knives, was limited, underscoring the challenges inherent in language learning.
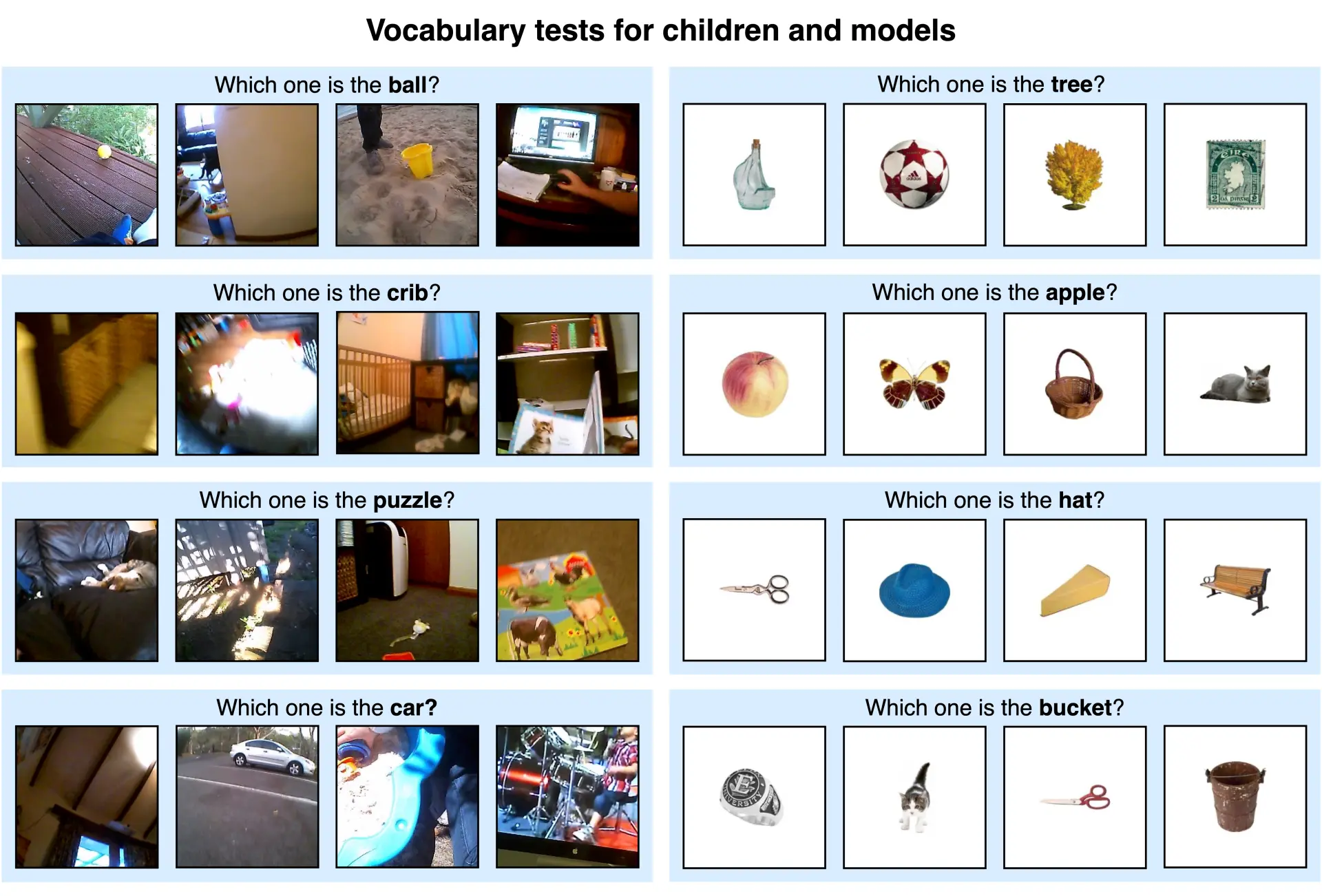
The study's authors were surprised that their relatively simple AI could achieve such language feats given the limited real-world data from one child. The results challenge the long-held belief that humans possess an innate language faculty essential for learning to speak. Instead, key components of early verbal development may be learnable from experience alone using basic but powerful learning mechanisms. However, CVCL's skills remain limited compared to a 2-year-old's language mastery.
One important thing to note is that the AI is benefiting from seeing the world from the point of view of how the baby interacts with it and how his parents react. As Andrei Barbu, a research scientist at Massachusetts Institute of Technology’s Computer Science and Artificial Intelligence Laboratory points out, the AI is essentially “harvesting what that child knows,” and that gives it an advantage in developing word associations. “If you took this model and you put it on a robot, and you had it run for 61 hours, you would not get the kind of data that they got here, that will then be useful for updating a model like this.”
Researchers plan to expand training data and tweak model architectures to better approximate children's efficient language understanding. By doing so, they hope to enhance its learning capabilities, potentially enabling it to grasp more complex linguistic patterns and behaviors. This approach mirrors the incremental learning journey of a child, emphasizing the importance of data diversity and volume in mastering language.
The research underscores the significant gap that still exists between human and machine learning. While AI can begin to learn word associations from limited datasets, the efficiency and depth of human language acquisition remain unmatched. Understanding these differences is crucial for both advancing AI technology and unraveling the intricacies of human cognition.
Who knows, maybe insights from baby-inspired AI like this will one day uncover the secrets of early learning, and lead to AI systems with more human-like common sense.