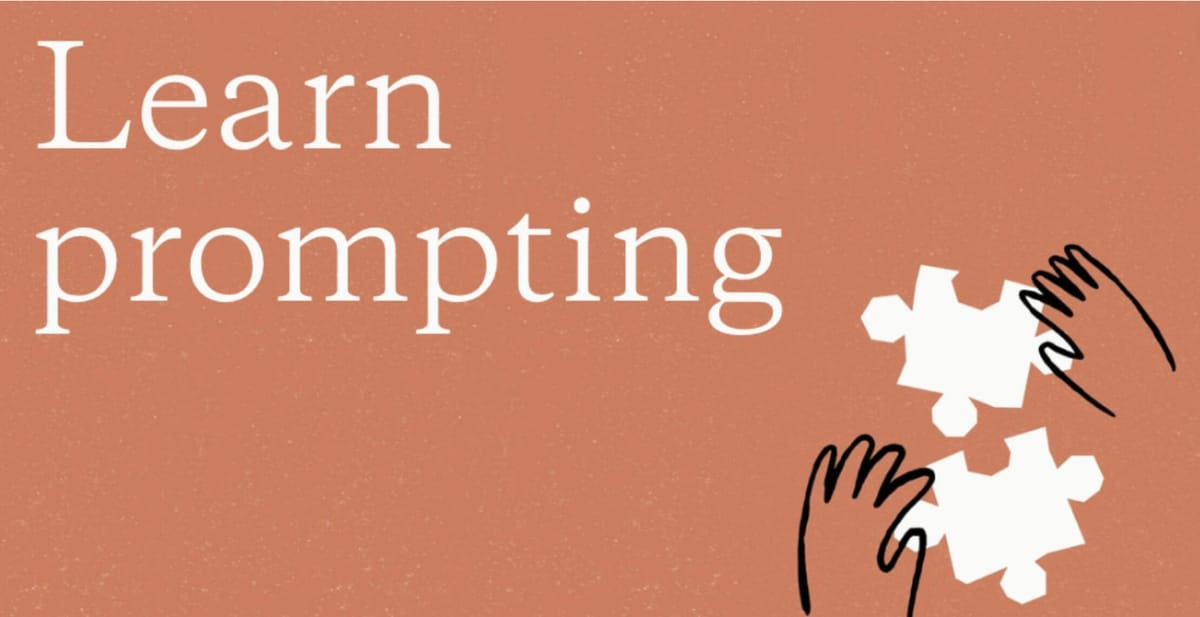
Anthropic, the AI research lab behind Claude, has unveiled two new educational resources on GitHub for developers looking to level up their prompt engineering skills. These courses offer valuable insights for those working with Claude and other large language models.
The first resource is an interactive tutorial designed for beginners. Spanning nine chapters, it covers fundamental concepts like prompt structure, clarity in instructions, and assigning roles to AI models. The tutorial progresses to more advanced topics, including output formatting and techniques to reduce hallucinations.
For experienced developers, Anthropic has created a "real-world prompting course." This advanced offering dives into practical applications of prompt engineering, showcasing examples of prompts used in production environments. The course is structured around five lessons, including a prompting recap and walkthroughs of prompts for medical, call summarization, and customer support scenarios.
We just released two new resources for learning prompt engineering.
— Alex Albert (@alexalbert__) August 21, 2024
1. An interactive intro to prompting tutorial for people just getting started with Claude
2. A real-world prompting course for developers building on the Anthropic API
Here's what they cover: pic.twitter.com/juIAlC6XLd
Alex Albert, Head of Developer Relations at Anthropic, highlighted the comprehensive nature of these resources on X (formerly Twitter). He noted that the introductory tutorial explains key terms like "chain of thought" and "few-shot learning," making it accessible to newcomers in the field.
These courses join two existing resources in Anthropic's GitHub repository: an API fundamentals course and a tool use course. Together, they form a robust curriculum for developers at various skill levels.
While the courses are tailored for Anthropic's Claude models, the principles taught are broadly applicable to other advanced language models. This makes them valuable resources for developers working across different AI platforms.
By providing developers with these accessible, hands-on resources, Anthropic not only empowers its user base but also contributes to the broader community of developers navigating the intricacies of prompt engineering. As more organizations integrate AI into their operations, mastering the art of prompt crafting will be crucial, not just for optimizing model outputs but for ensuring these systems meet real-world needs effectively.