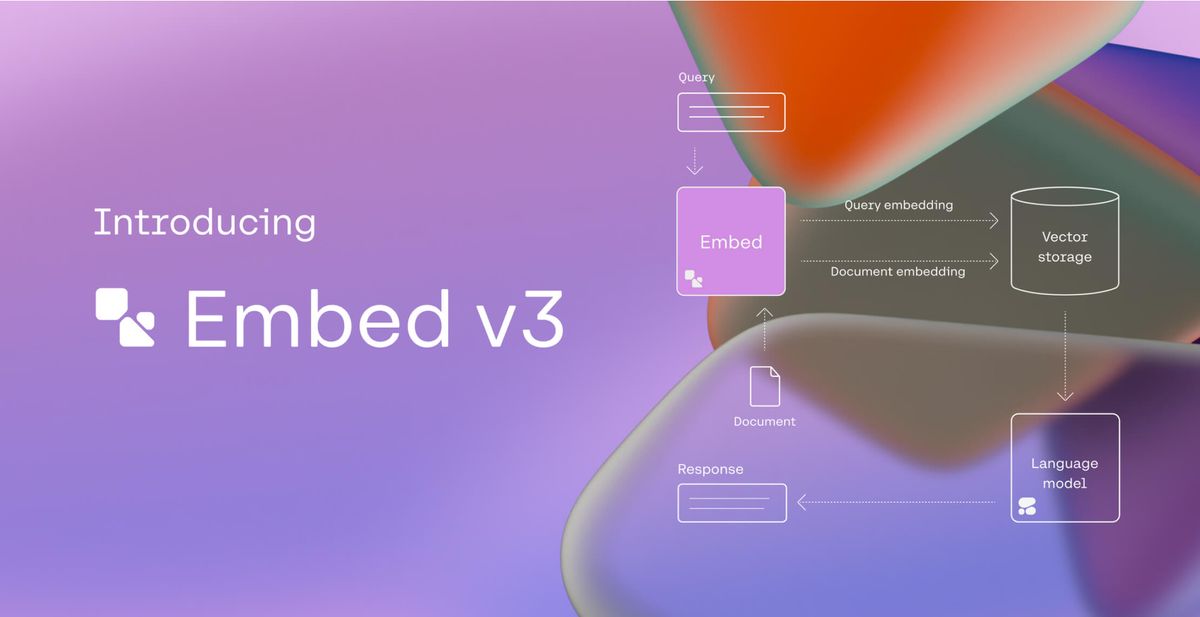
Cohere has unveiled Embed v3, its latest and most capable text embedding model that achieves state-of-the-art performance on trusted benchmarks. Embed v3 demonstrates significant improvements in semantic search accuracy, especially for real-world, noisy datasets.
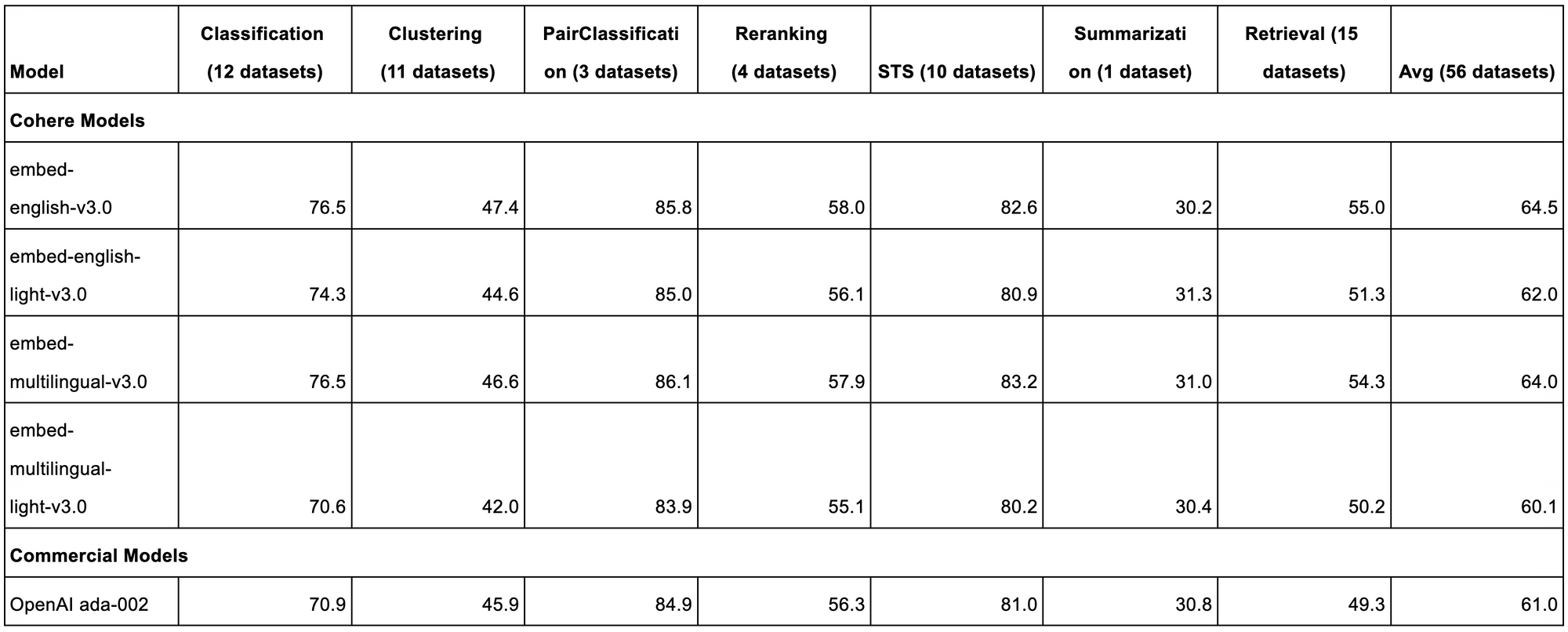
Embed v3 stands out in the field of text embedding models with its impressive performance on well-known benchmarks such as the Massive Text Embedding Benchmark (MTEB) and the Benchmark for Evaluating Information Retrieval (BEIR). This model not only tops the charts among 90+ models on MTEB but also excels in zero-shot dense retrieval on BEIR.
Embeddings are vector representations of text that capture semantic meaning. They enable powerful applications like semantic search by allowing comparisons between search queries and documents based on their vector similarity.
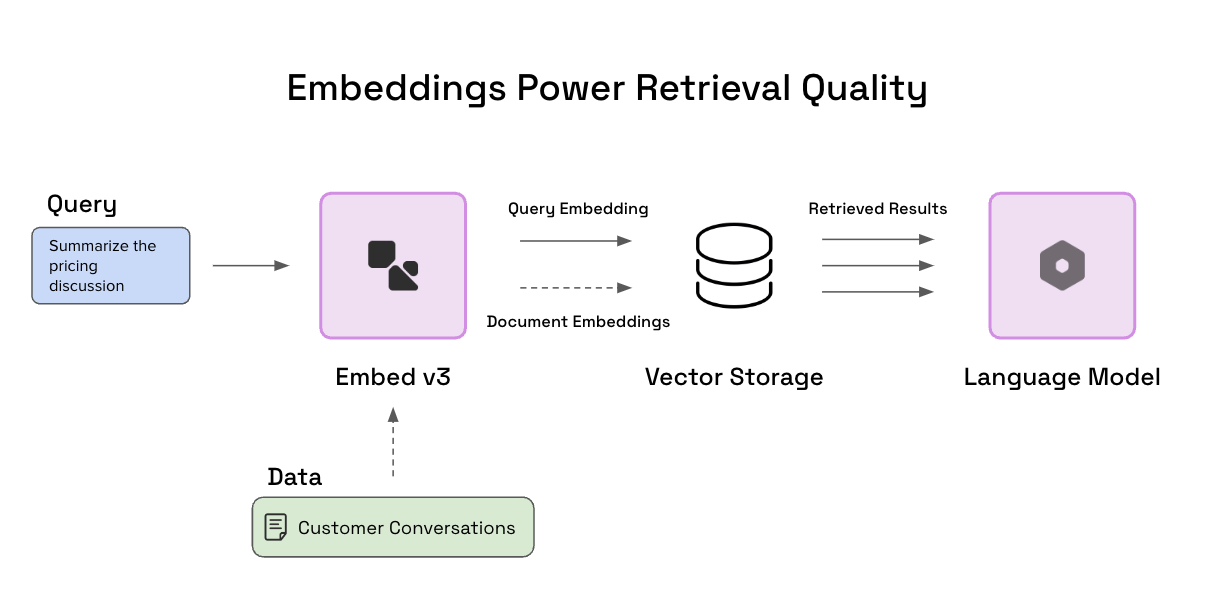
However, consistently retrieving the most relevant results remains challenging when dealing with noisy real-world data of varying quality. Documents may match a search query topic while containing little useful information.
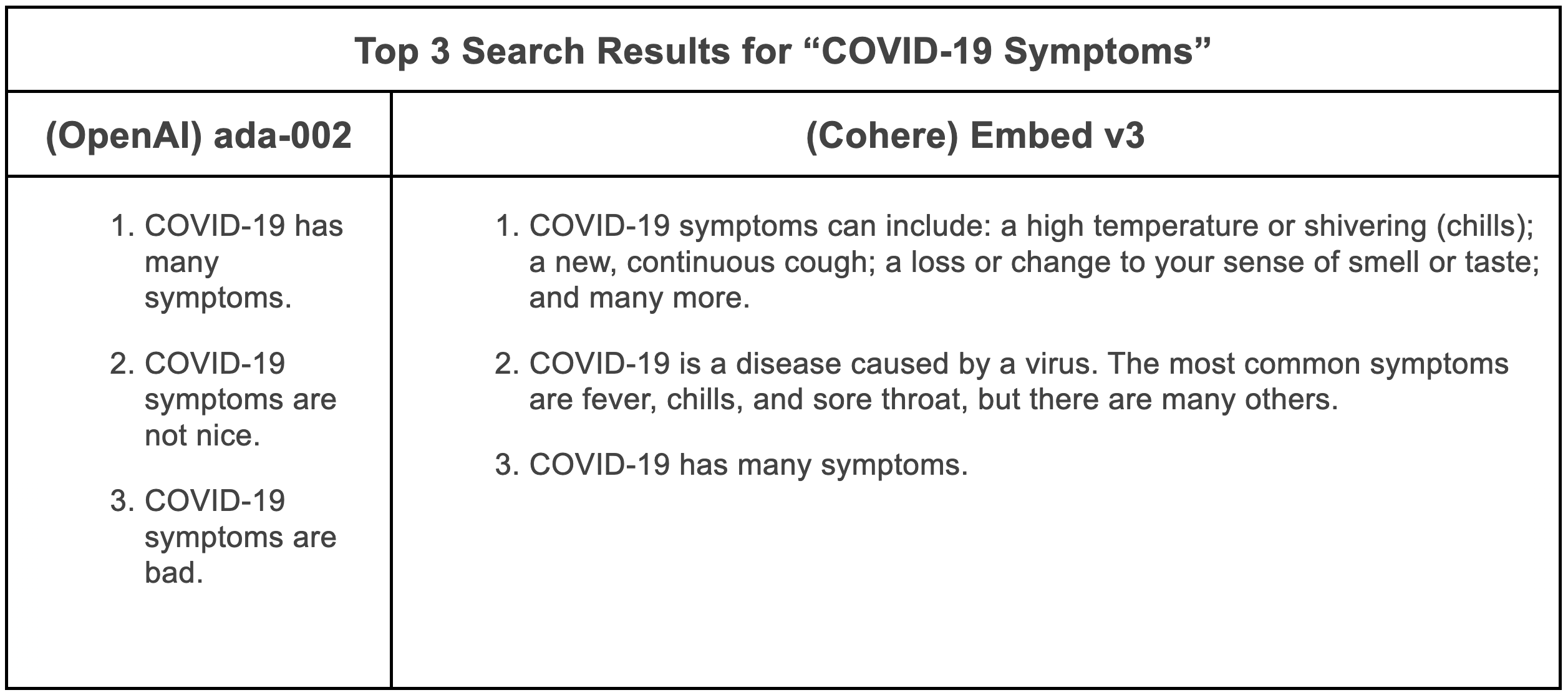
Embed v3 tackles this problem through enhancements like evaluating content quality and relevance within the vector space itself. This allows properly ranking documents by both topic match and information quality.
Evaluations show these improvements yield substantial gains for semantic search applications. For example, on the TREC-COVID dataset based on a noisy CORD-19 web crawl, Embed v3 achieves much higher search accuracy than benchmarks like OpenAI's ada-002, correctly surfacing documents with the most pertinent details.
It also excels at "multi-hop" questions that require synthesizing details from multiple documents, critical for retrieval-augmented generative AI applications. Testing on HotpotQA showed significantly better performance at retrieving all necessary context to answer complex queries.
Cohere says Embed v3's training methodology is optimized for compression-awareness, enabling efficiently handling billions of embeddings without excessive infrastructure costs. It introduces a new mandatory parameter: input_type
. This ensures that the embedding model is optimized for specific tasks such as search_document
, search_query
, classification
, and clustering
, enhancing the quality and relevance of the results.
Embed v3 is available in English and multilingual versions supporting over 100 languages. The multilingual models support 100+ languages and can be used to search within a language (e.g., search with a French query on French documents) and across languages (e.g., search with a Chinese query on Finnish documents).
Cohere's Embed v3 represents a significant leap forward in the field of text embeddings. Its unparalleled performance, robustness to noisy data, and cost-efficient operation make it a valuable tool for developers looking to improve search applications and RAG systems.