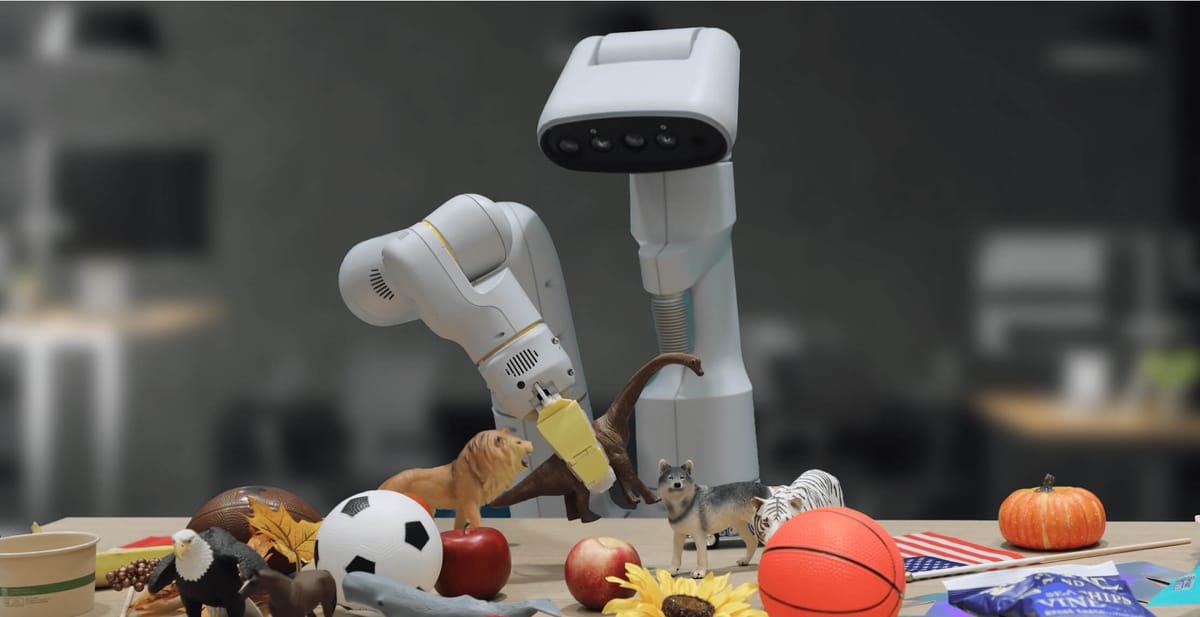
Google DeepMind has unveiled a suite of advances that tackle key challenges in robotics like autonomous data collection, computational efficiency, and task generalization. Together, these new systems - AutoRT, SARA-RT, and RT-Trajectory - demonstrate strides towards more capable helper robots.
AutoRT Uses Foundation Models to Direct Robot Fleets
AutoRT allows groups of robots to gather training data autonomously in unseen environments. It works by combining a visual language model (VLM) to perceive the surroundings with a large language model (LLM) that proposes diverse manipulation tasks suited to what the robots see in its environment. The system directs robots, each equipped with a camera and an end effector, to perform diverse tasks in various settings. Before execution, the tasks are filtered for safety by an "LLM legislator" guided by a "Robot Constitution" inspired by Asimov's Three Laws of Robotics.
In tests across multiple office buildings over 7 months, AutoRT directed up to 20 robots simultaneously (52 unique robots in total) , collecting over 77,000 sessions covering 6,650 unique tasks - all while ensuring rigorous safeguards were upheld. This real-world robot data was found to be more varied than prior datasets.
SARA-RT Enhances Efficiency of Robotics Transformers
SARA-RT, or Self-Adaptive Robust Attention for Robotics Transformers, is a new system that converts models like the billion-parameter RT-2 into faster, equally proficient versions for improved on-robot deployment. It uses a new "up-training" fine-tuning method to change quadratic complexity attention mechanisms into linear ones, sharply reducing computational load.
Applied to RT-2, SARA-RT led to a 10.6% accuracy boost and 14% faster decision-making after being provided with a short history of images. It also more than doubled the speed of Point Cloud Transformers for spatial perception - showcasing broad applicability.
RT-Trajectory Enables Robots to Generalize Skills to New Tasks
RT-Trajectory adds trajectory sketches - simplified 2D outlines of the robot's motions - to the training data. This extra visual guidance allows policies to interpret instructions in the context of the environment and generalize more effectively.
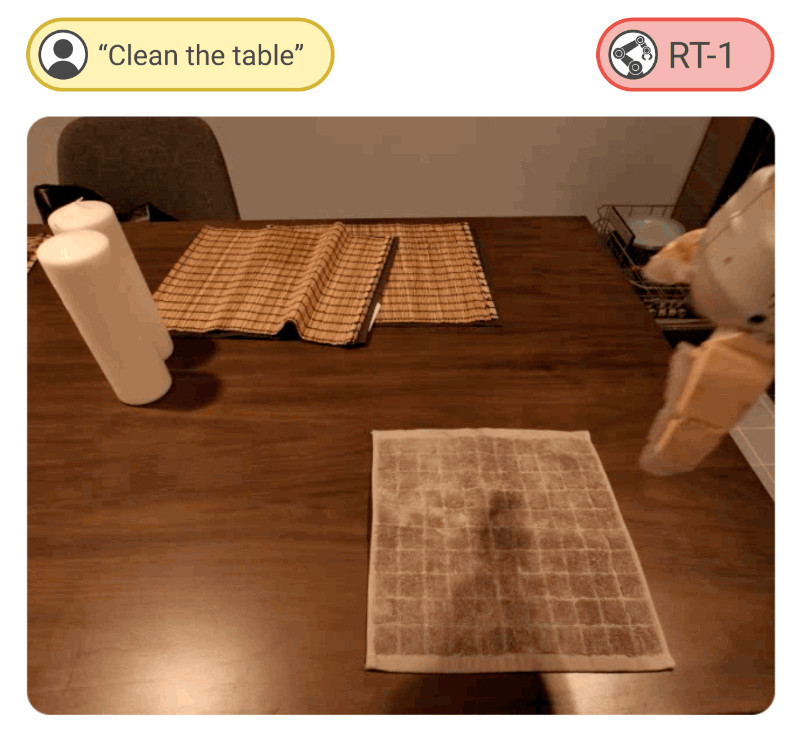
In evaluations, RT-Trajectory doubled the 63% success rate of existing methods on unseen tasks. It can also generate sketches from human demonstrations, hand drawings, or modern foundation models, making it highly versatile.
Google DeepMind's latest developments represent a cohesive effort towards creating more capable and versatile robots. The integration of AutoRT's large-scale data collection, SARA-RT's efficiency, and RT-Trajectory's motion generalization promises a future where robots can perform a wide array of tasks with precision and adaptability. These innovations not only improve current robotic capabilities but also lay the groundwork for future advancements in the field.
Together, these advances inch closer to the helper robots of the future - able to collect their own data, think quickly, and adapt skills to novel situations. While still just research prototypes, they highlight DeepMind's progress in discovering inventive ways to overcome robotics' open challenges. As these technologies continue to evolve, they hold the promise of bringing us closer to a future where robots seamlessly integrate into our daily lives and offer assistance with a range of complex tasks.