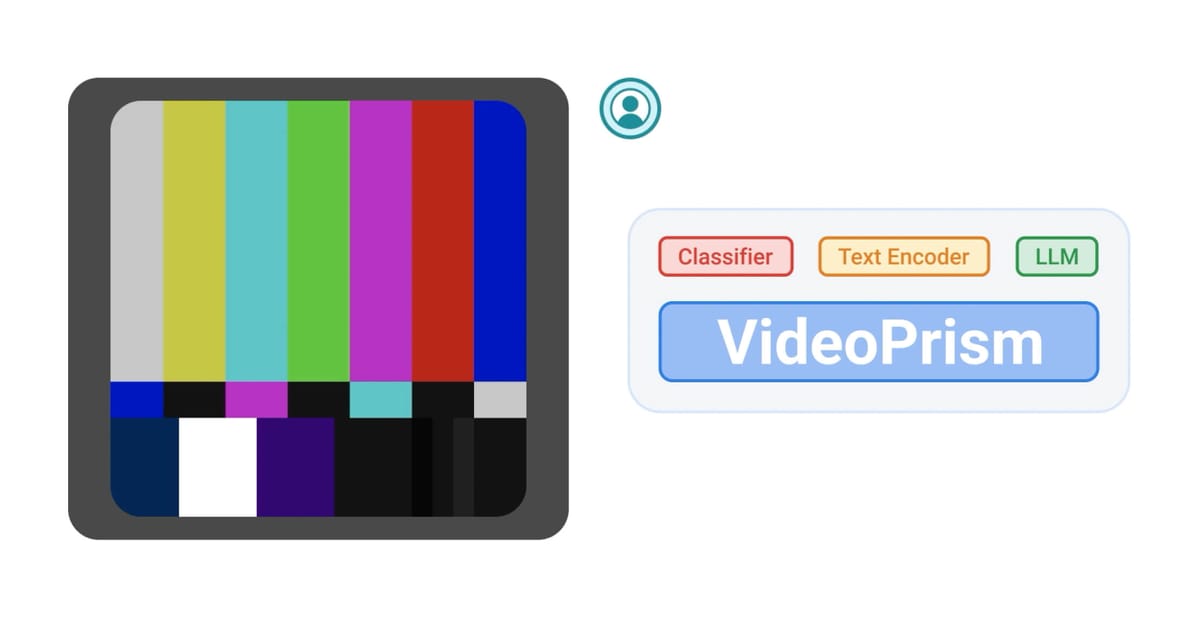
Google AI has introduced VideoPrism, new research that introduces a groundbreaking single model for general-purpose video understanding. This foundational visual encoder is designed to handle a wide array of tasks, including classification, localization, retrieval, captioning, and question answering.
In their paper, Google says VideoPrism's development is driven by innovations in both pre-training data and modeling strategy. The model is pre-trained on a massive and diverse dataset, comprising 36 million high-quality video-text pairs and 582 million video clips with noisy or machine-generated parallel text. This hybrid data approach allows VideoPrism to learn from both video-text pairs and the videos themselves.
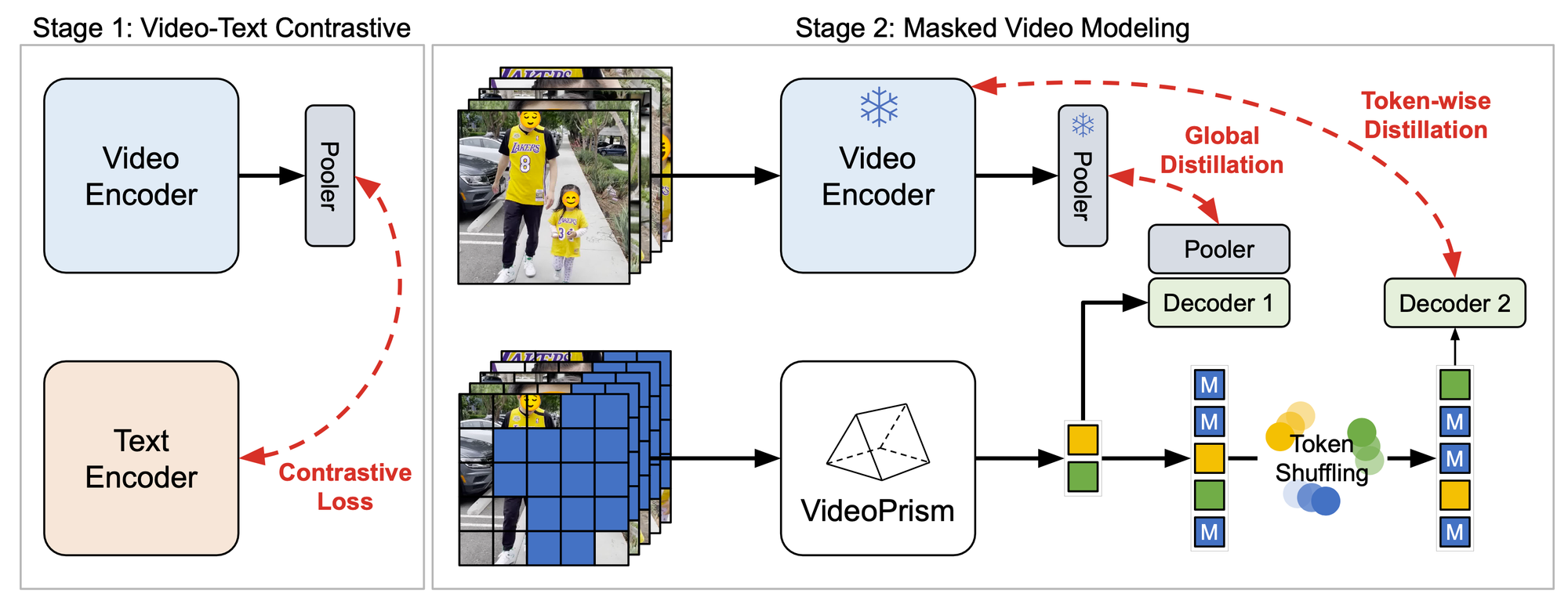
The pre-training process involves two stages. First, contrastive learning is used to teach the model to match videos with their text descriptions, building a foundation for matching semantic language content to visual content. Then, the model predicts masked patches in videos, leveraging the knowledge acquired in the first stage. This unique setup enables VideoPrism to excel in tasks that demand an understanding of both appearance and motion.
Extensive evaluations across four broad categories of video understanding tasks demonstrate VideoPrism's exceptional performance. The model achieves state-of-the-art results on 30 out of 33 video understanding benchmarks, all with minimal adaptation of a single, frozen model. These benchmarks include video classification and localization, video-text retrieval, video captioning, question answering, and scientific video understanding.
VideoPrism's ability to combine with large language models further unlocks its potential for handling various video-language tasks. When paired with a text encoder or a language decoder, VideoPrism sets new standards on a broad and challenging set of vision-language benchmarks. The model's capability to understand complex motions and appearances in videos is particularly impressive.
Perhaps most exciting is VideoPrism's potential for scientific applications. The model not only performs exceptionally well on datasets used by scientists across domains like ethology, behavioral neuroscience, and ecology but actually surpasses models designed specifically for those tasks. This suggests that tools like VideoPrism could transform how scientists analyze video data across different fields.
"VideoPrism paves the way for future breakthroughs at the intersection of AI and video analysis, helping to realize the potential of video foundation models across domains such as scientific discovery, education, and healthcare" - Long Zhao, Senior Research Scientist at Google Research, and Ting Liu, Senior Staff Software Engineer
VideoPrism's introduction marks a significant milestone in the development of general-purpose video understanding models. Its ability to generalize across a wide range of tasks and its potential for real-world applications make it a promising tool for researchers and professionals across various domains. As Google AI continues its responsible research in this space, guided by its AI Principles, we can expect to see more breakthroughs that harness the power of artificial intelligence to understand and interpret the vast amount of video data available.