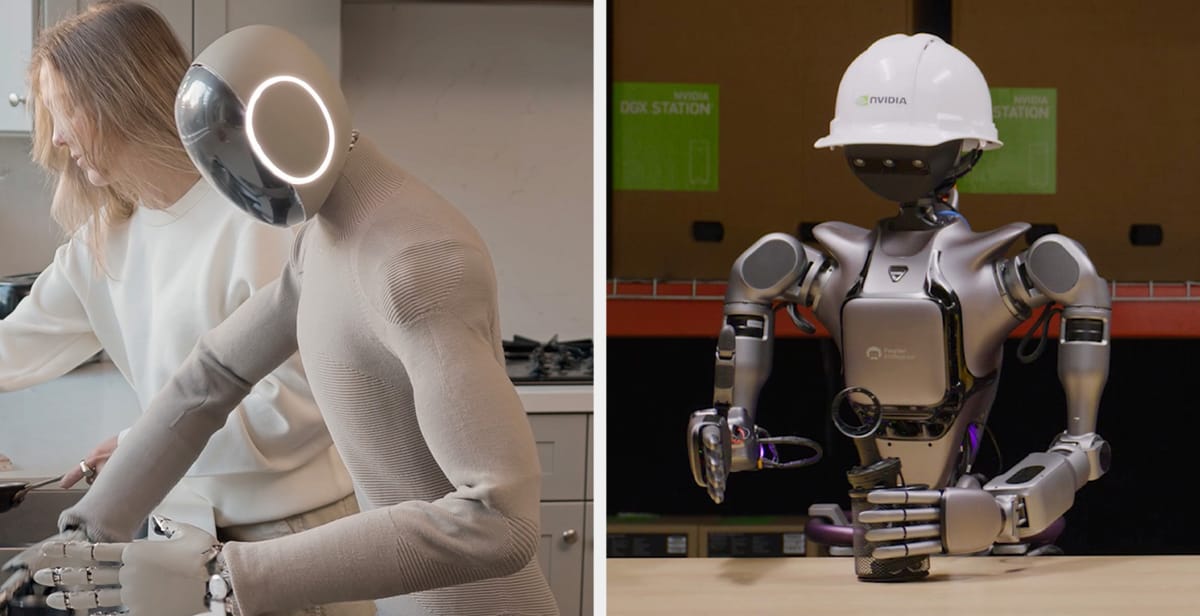
NVIDIA has introduced Isaac GR00T N1, a foundation model designed to help humanoid robots develop generalized reasoning and practical skills. The announcement, made at GTC 2025, will accelerate the development of adaptable robots that can perform a range of tasks in dynamic environments.
Key Points:
- Isaac GR00T N1 is an open-source foundation model for humanoid robots.
- It features a dual-system architecture, inspired by human cognition.
- Developers can customize the model using real or synthetic data.
- Early adopters include Boston Dynamics, Agility Robotics, and 1X Technologies.
The model is fully customizable, allowing developers to refine its capabilities for specific applications using post-training with real or synthetic data. At the core of GR00T N1 is a dual-system architecture that mimics human cognition:
- System 1 is a fast-thinking action model, akin to human reflexes and intuition, enabling quick responses to environmental changes.
- System 2 is a slower, reasoning-based model that interprets instructions using a vision-language approach to plan and execute tasks methodically.
This combination enables robots to perform a variety of complex activities, from simple object grasping to multistep tasks requiring long-term planning. NVIDIA has made GR00T N1’s training data available on Hugging Face and GitHub, allowing developers to integrate it with their own hardware and workflows.
During his keynote, CEO Jensen Huang showcased 1X’s NEO Gamma humanoid robot autonomously completing tidying tasks using a policy built on GR00T N1. Bernt Børnich, CEO of 1X Technologies, called the model a “major breakthrough for robot reasoning and skills,” emphasizing its ability to adapt with minimal additional training.
Other early adopters include Boston Dynamics, Agility Robotics, Mentee Robotics, and NEURA Robotics, all of whom are integrating GR00T N1 into their humanoid development programs.
NVIDIA also introduced the Isaac GR00T Blueprint, a simulation framework that allows developers to generate large-scale synthetic data for training. In just 11 hours, the system produced 780,000 synthetic motion trajectories—equivalent to nine months of human demonstration data—boosting GR00T N1’s performance by 40%.