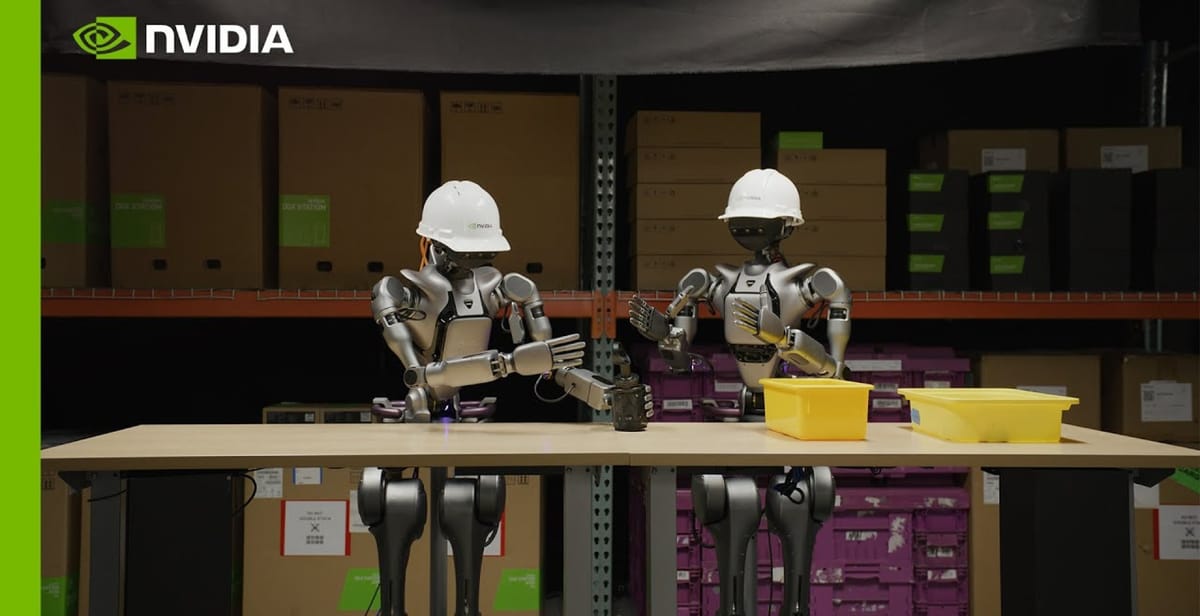
At COMPUTEX, NVIDIA CEO Jensen Huang announced a significant expansion of the company's robotics development infrastructure, including a new version of its foundation model for humanoid robots. The company is positioning these tools as critical building blocks for what Huang boldly called "the next industrial revolution."
Key Points:
- NVIDIA's GR00T N1.5 model was created in just 36 hours using synthetic data versus the nearly three months it would take with manual collection
- Major robotics companies including Boston Dynamics and Agility Robotics are already adopting NVIDIA's platform technologies
- The new tools generate synthetic motion data to train robots, potentially closing the "data gap" that's been holding back humanoid robot development
The star of the announcement is NVIDIA Isaac GR00T N1.5, an updated version of the company's open foundation model for humanoid reasoning and movement. What makes this update particularly interesting isn't just the improved capabilities but how it was created — using synthetic data generated by another new NVIDIA tool called GR00T-Dreams.
According to NVIDIA, the GR00T-Dreams system allowed them to develop the N1.5 update in just 36 hours, compared to what would have required nearly three months of manual human data collection. That's a stunning efficiency improvement for a field where data collection has been a major bottleneck.
"Physical AI and robotics will bring about the next industrial revolution," said Jensen Huang during his keynote. "From AI brains for robots to simulated worlds to practice in or AI supercomputers for training foundation models, NVIDIA provides building blocks for every stage of the robotics development journey."
The core innovation here is how NVIDIA is addressing the data problem. Training robots to perform useful tasks traditionally requires massive amounts of data — typically collected through manual demonstrations or real-world testing, which is slow, expensive, and often impractical. GR00T-Dreams offers a shortcut by generating synthetic motion data that can be used to teach robots new behaviors.
The system works by first post-training NVIDIA's Cosmos Predict world foundation models for a specific robot. Then, from just a single image, GR00T-Dreams generates videos of the robot performing new tasks in various environments and extracts "action tokens" — compressed data that can be used to teach robots how to perform these tasks.
It's a clever approach to a stubborn problem, essentially using AI to generate the data needed to train more AI.
The updated GR00T N1.5 model reportedly shows significant improvements in adapting to new environments and workspace configurations, as well as recognizing objects through user instructions. NVIDIA claims it substantially improves success rates for common material handling and manufacturing tasks like sorting objects.
What might be most telling about the significance of these announcements is the roster of companies already adopting the technology. Robotics heavyweights including Boston Dynamics, Agility Robotics, Foxconn, and NEURA Robotics are all working with NVIDIA's Isaac platform technologies.
Complementing these new AI models, NVIDIA also unveiled several simulation technologies to help further close the data and testing gap, including a new world foundation model called Cosmos Reason, an upgraded Cosmos Predict 2, and frameworks for generating synthetic motion trajectories. They're also making Isaac Sim 5.0 openly available on GitHub.
For companies looking to implement these technologies, NVIDIA announced that global system manufacturers including Cisco, Dell, HPE, Lenovo, and Supermicro are building NVIDIA RTX PRO 6000 workstations and servers designed to run robot development workloads. When more compute is needed, developers can tap into NVIDIA Blackwell systems like GB200 NVL72, which the company claims offers up to 18x greater performance for data processing.
The immediate applications target industrial settings — AeiRobot is using the models to enable their ALICE4 robot to understand natural language instructions and execute complex pick-and-place workflows, while Foxlink Group is focusing on improving industrial robot manipulator flexibility.
But the broader implications could be substantial. If NVIDIA can truly solve the data problem for training humanoid robots, we might see much faster progress in developing robots capable of operating in complex, unstructured environments — potentially bringing the sci-fi vision of capable humanoid robots much closer to reality.